Abstract
This research paper delves into the complex topic of social robots as well as human robot interactions (HRI), and aims to provide a comprehensive overview to inform how social robots should develop in the future. Through an extensive literature review, we have synthesised information on their evolution, different functions, design principles, impacts, challenges, and future endeavours. From simple sketches to artificial intelligence (AI) integrated pieces of machinery, robots have evolved considerably over a short period of time. One thing that has remained constant, however, is the desire for a productive coexistence between humans and robots. In recent years, there has been an increase in the implication of socially intelligent robots in different sectors, such as education, healthcare, and manufacturing. This has therefore given rise to scepticism in terms of potential job displacement and ethical considerations. Consequently, despite the potential for socially intelligent robots, the challenges of their assimilation into human society are evident. This requires careful consideration of their design features, both physical and in terms of code, as well as policies to determine their place in our societal framework. They have had economic, social, cultural, and political impacts, rendering interdisciplinary collaboration crucial as research reveals more about the steps that need to be taken in optimising HRI.
1. Introduction
1.1. Evolution of Social Robots
Although the term “robot” is a relatively recent term – first coined by Czech writer, Karel Čapek, in his 1920 play “R.U.R.” (Rossum’s Universal Robots) – robots are not a novel concept (Reilly, 2011). Historical evidence reveals the history of robots spans over a thousand years, with some examples dating back to the ancient Greeks. Throughout history, however, much of these robots have been concepts and ideas rather than realisations, ranging from ten-metre-tall robot guardians to Leonardo Da Vinci’s technical drawing of a fully automated robot knight (Thalmann, 2022).
Before exploring key developments of social robots and HRI in the 20th century to the present, let’s define what a robot is. A robot, according to the International Organization for Standardization (ISO) (2021, 3.1), is “a programmed actuated mechanism with a degree of autonomy to perform locomotion, manipulation or positioning.” The definition of a social robot, however, is more up to interpretation, with different papers proposing definitions of varying degrees of ambiguity. The book Emotions and Affect in Human Factors and Human-Computer Interaction underscores the need for intentional communication that is comprehensive to humans, and the desire to achieve goals (Daily et al., 2017, Ch. 9). On the other hand, David et al. (2017) use a brief and more vague description, simply outlining the necessity for social capabilities that generate social responses. While there are still discrepancies, the theme of being able to communicate and interact with humans is recurrent in all of the definitions.
The following section lists some examples of robots which have created milestones in the development of social robots over the past 100 years.
1.1.1. Eric the robot (1928)
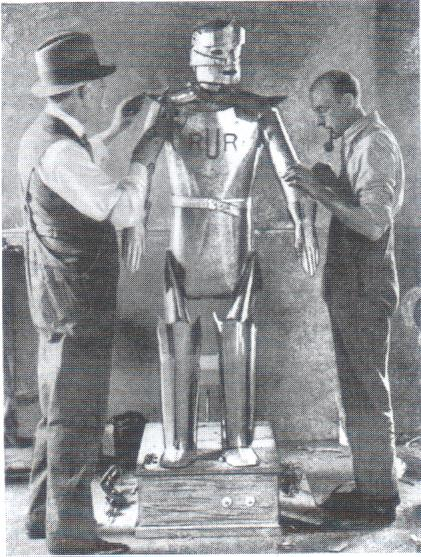
Figure 1: Eric the Robot (Cyberneticzoo, 2009, sec: The Morning Herald).
Originally built in 1928 by Captain WH Richards and AH Reffell, Eric was one of the world’s first robots (Burgess, 2017). The advancements that Richards and Reffell made were vast: Eric could move his arms, walk, talk, and obey instructions. Thus, Eric was described as the “perfect man” by the New York Press as he dazzled audiences with his abilities (Jozuka, 2017). While ethics and job displacement dominate present-day dialogue on robots, audiences in the 1920s celebrated Eric’s ability to perform the toilsome labour of mechanical workers. Despite his mysterious disappearance soon after, his legacy survives with a replica built by the London Science Museum in 2017 (Jozuka, 2017).
1.1.2. William Grey Walter’s Tortoises (1940s-50s)
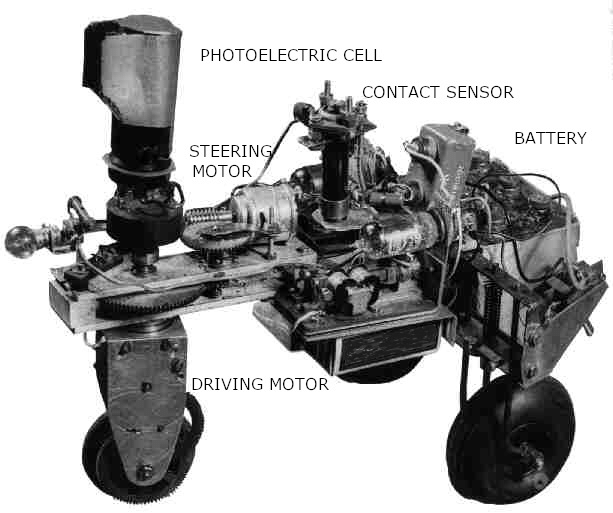
Figure 2: Machina Speculatrix (Walter, 1953 cited in Nolfi, 2021, sec. 2.1).
American neurologist William Grey Walter, responsible for identifying the brain waves known as Alpha, Delta, and Theta, constructed remarkably advanced robots for his day in order to advance his theories on nervous systems (LeBouthillier, 1999). To better understand the operation of animal brains, two of his robots, named Elmer and Elsie (short for Electro Mechanical Robots and Light Sensitive), represented some of the first autonomous robots. This was one of the earliest efforts to understand the mechanics behind animal and human behaviour and reproduce it artificially. Walter’s work inspired generations of robotics enthusiasts and demonstrated the wide array of possibilities for HRI (LeBouthillier, 1999).
1.1.3. Kismet (1990s)
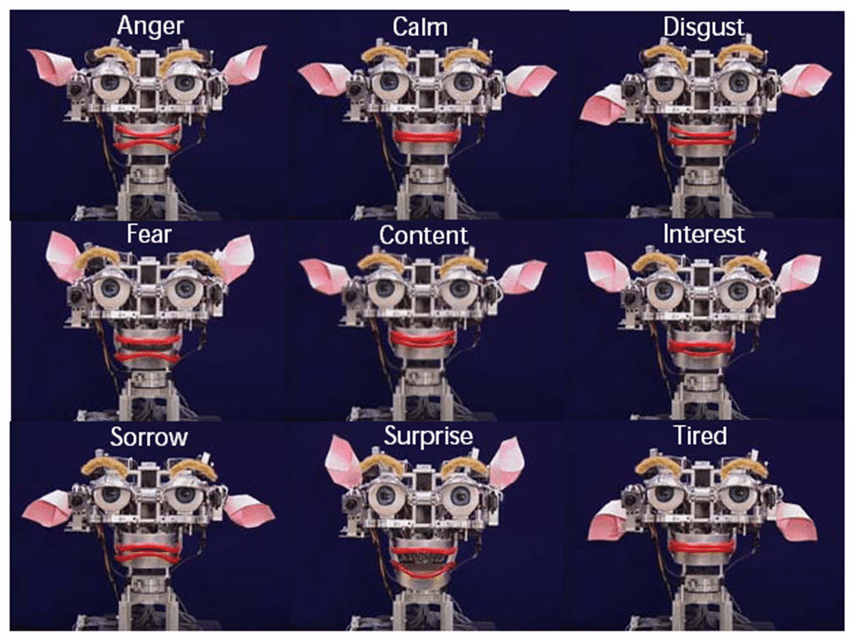
Figure 3: The Nine Expressions of Kismet (Pautler, 2007, sec: sociable agents).
Kismet, designed by Dr. Breazeal at the Massachusetts Institute of Technology (MIT) AI Lab in the late 1990s, was the first robot designed explicitly for HRI, and is widely recognised as the foundation for a new area of study in social robotics (Breazeal, 2000). Kismet was inspired by infant social development, to engage in natural interactions with a human as a parent figure, nodding to parent-child exchanges. According to Dr. Breazeal (2000), these cognitive interactions are essential to infant development, and may be helpful in social robot development as well.
1.1.4. Pepper (2014)
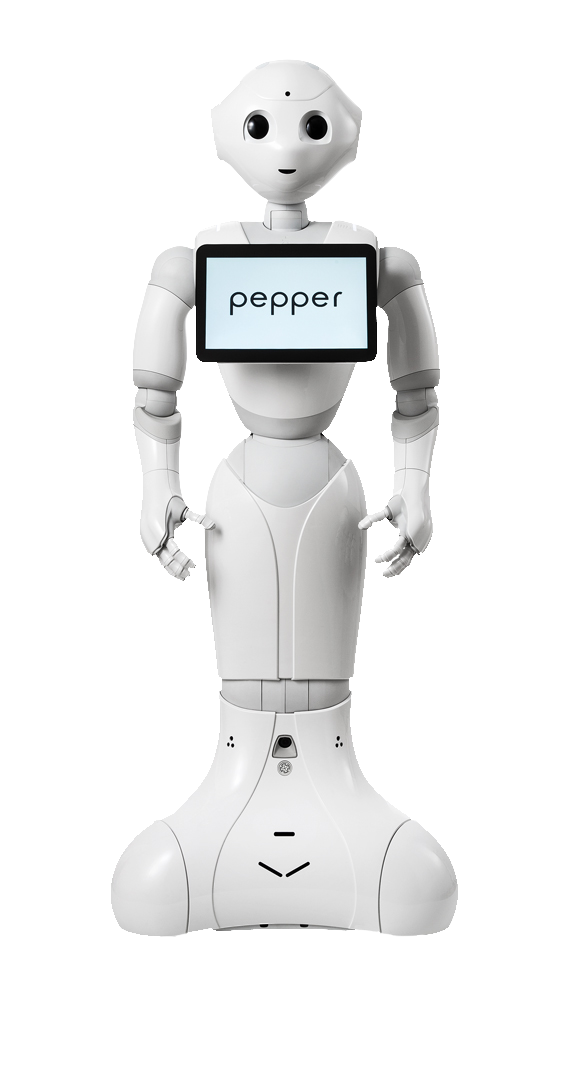
Figure 4: Pepper Robot (Pandey and Gelin, 2018, sec: The Need and the Design Principles).
The Pepper robot, designed by SoftBank Robotics, was created with the vision of automated robots coexisting alongside humans, allowing them to progress to a smarter, safer, healthier, and happier existence (Wakefield, 2021). Capable of exhibiting body language, perceiving and interacting with its surroundings, and moving around, it is equipped for multifaceted interactions. Designed to be more approachable, it does not have any sharp edges, is 1.2 metres tall, can roam around on wheels, and is adorned with a tablet (Gelin and Pandey, 2018). However, Pepper was expensive to buy and maintain, and its functionality reportedly limited to that of an iPad (Nussey, 2021). Hence, the sale of Pepper has since slumped and Softbank discontinued its production in 2021. This case study shows how important the design features of social robots are in order to make them worthwhile in society. The exaggeration of Pepper’s abilities may even have been detrimental to research in robotics as it gave the wrong impressions about the communication abilities of AI (Wakefield, 2021). Nevertheless, Pepper has demonstrated the potential roles social robots could have in society, widening the scope to integrate new technologies that are more suitable for human coexistence (Gelin and Pandey, 2018).
Over the past century, the face of robots has evolved considerably, with each milestone contributing in some form to the current developments in robotics. With each example, the challenges of incorporating the nuances of human communication into robots have been revealed. The progress made in this field has led to social robots having an increased potential to play significant societal roles.
1.2 Social Robots
While all robots have evolved and gained a more prominent role in society today, the rise of social robots has had the largest effect on day-to-day HRI, especially for those in need of emotional support. In recent years, social robots have gained a more important role in various sectors such as healthcare, entertainment, and hospitality (Reis et al., 2020 cited in Obaigbena et al., 2024).
According to social scientist, Kate Darling, social robots are programmable machines with a physical body that can mimic human social behaviour patterns and emotions to interact with humans (Campa, 2016). Thus, they differ from other types of robots, such as industrial or service robots, in their ability to adapt their behaviour based on human emotions and social cues. Campa (2016) further claims that social robots are often based on humans or animals since they allow for humans to communicate in a more natural and comfortable way when interacting with these machines. For these mechanoids to mimic and adapt based on human social behaviour, however, they often need key characteristics and functions, many of which have to do with the recent advances in AI and its rise in HRI.
1.2.1. Human Robot Interactions (HRI)
According to Sheridan (2016), there are four main areas of HRI. One area of HRI includes human supervised control of robots in basic and routine tasks. These tasks are often related to telerobots which can carry out limited actions automatically based on a computer program, which allows it to sense its environment and its own joint positions while giving data back to a human who constantly updates the instructions of the robot (Sheridan, 2016). Another area of HRI are teleoperators which can perform mobility tasks based on human control movements in dangerous or naturally inaccessible areas (Sheridan, 2016). An example of these robots are space rovers such as the Mars Rover, Spirit (MER-A), which has previously landed on Mars, a planet that is currently inhabitable for humans (Standard Bots, 2024). The third area of HRI is in automated vehicles with a human supervisor and passenger, including self-driving cars. Lastly, Sheridan (2016) claims the final area of HRI involves human-robot social interaction, which is the basis of this paper. In this area of HRI, robots provide entertainment, therapy, comfort, assistance, or teaching. These social robots adapt their responses to the actions of people, allowing them to act socially as if they were humans (Knox et al., 2013). Despite their success, these social robots can also be the most concerning and dangerous since they have the capability to be used with little to no supervision or changing of their programming.
1.2.2 Physical Features of Social Robots
Socially intelligent robots contain many key features that allow them to interact naturally and easily with humans. For one, most social robots contain a physical structure with a body, a head, and some type of focal point to respond to and look at (Onyeulo & Gandhi, 2020). However, according to Onyeulo and Gandhi (2020), these mechanisms do not need to be humanoid for an engaging interaction, but many humans prefer for them to look like something they can recognise, which includes a human, an animal, or a machine. In addition, many can respond to touch, sound, and visual movements due to advanced equipment and technology like sensors, cameras, microphones, and more. Since social robots are defined as autonomous beings, they also need to be able to adapt based on human feedback and their environment. This means these robots also have the ability to adjust to the cultural and societal norms of their surroundings (Korn et al., 2021).
1.2.3 Programmable Features of Social Robots
The use of AI is the source of many of the main traits of social robots. Social robots can often become personalised and tailored to each individual user’s needs, so they modify their behaviour accordingly (Tarakli et al., 2023). According to Obaigbena et al. (2024), the use of AI algorithms can allow them to perceive human emotions, speak naturally, and adapt their behaviour based on human actions. For example, machine learning (ML) algorithms, which are used to analyse user data, are often incorporated into social robots to tailor their behaviour based on users’ preferences and feedback (Obaigbena et al., 2024). The various AI algorithms that are employed in these social machines are described in further detail in Section 1.3.
1.2.4 Roles of Social Robots
Briefly touched on earlier in Section 1.2.1, the human-like characteristics of social robots enable them to participate in essential activities across various sectors, including hospitality, entertainment, and healthcare. In recent years, social robots have increasingly taken on roles at the front desk, ranging from check-in assistants to concierges, due to their ability to improve the guest’s experience. According to hospitality expert, Martijn Barten, social robots are enhancing guest convenience through efficient self-service, 24/7 customer support, and personalised service through analysis of guests’ preferences and behaviours (Barten, 2024).
Moreover, Zhenhua and Feng (2024) claim that social robots have been widely received in the realm of entertainment due to the added fun and vitality they provide to the art of performance. These socially intelligent robots partake in roles such as performance, crowd engagement, and immersion to refine the audience’s experience. This branch of HRI is particularly valuable for performers, as its AI algorithms allow artists to better understand the audience’s preferences and emotional responses, enabling them to create more impactful and resonant works (Zhenhua & Feng, 2024).
Finally, social robots play a significant role in the healthcare industry, where they are utilised for alleviating and preventing illnesses. AI algorithms leverage vast amounts of data collected by sensors to accurately interpret social situations. This is particularly important in promoting well-being through which social robots’ companionship and presence alleviate anxiety and depression. Additionally, social robots were crucial in reducing the spread of COVID-19, having monitored and supported patients in physical distancing and isolation (González-González et al., 2021). The impacts of these roles and the challenges they raise are described further in Sections 2 and 3 respectively.
1.2.5 Examples of Social Robots
There are many specific social robots that play a key role in such sectors. Ben Powell’s robot, named Orbit (Figure 5(a)), is an example pertaining to healthcare as it is designed to help children with autism develop social skills. Children with autism often struggle to socialise because of difficulties with emotional awareness in social contexts (Digitale, 2023). Therefore, Orbit is equipped with multifaceted mediums of expression and engagement (storytelling, physical touch, and visual communication like facial expressions) to teach social appropriateness and awareness (Biba, 2024).
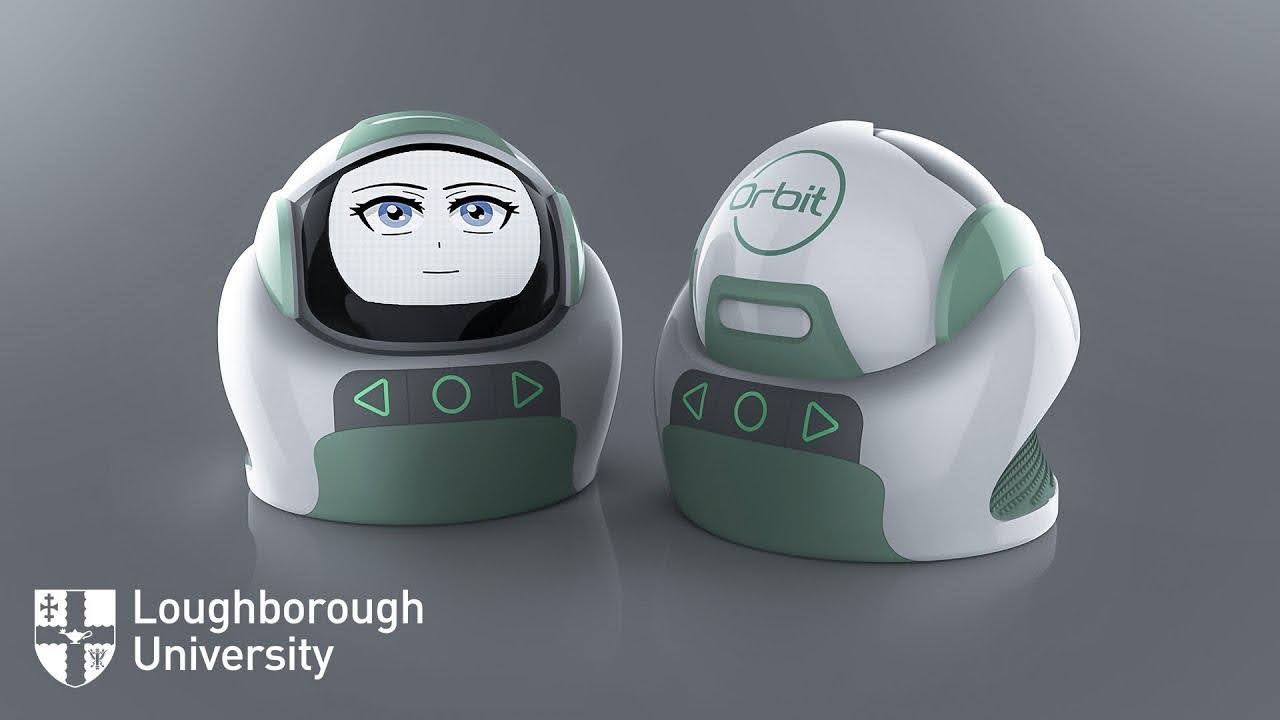
Figure 5: (a) Orbit Robot (Cox, 2022, sec: 1).
Moreover, healthcare robots have evolved to include pets like Jennie, the robotic dog (Figure 5(b)). Jennie offers emotional support to people with chronic conditions such as dementia, alleviating symptoms of depression, anxiety and loneliness (Biba, 2024). Similar to a golden Labrador retriever puppy, the robot engages with humans by wagging its tail and making real puppy sounds.
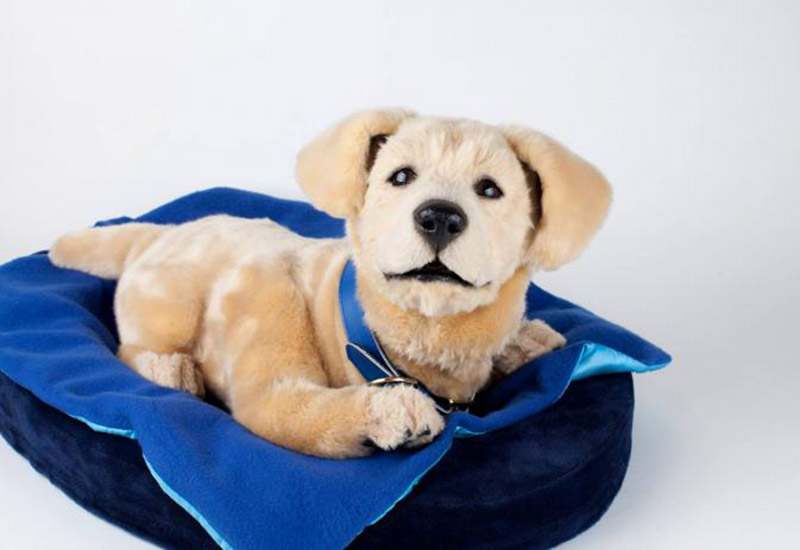
Figure 5: (b) Jennie Robot (Darrisaw, 2019, sec: introduction).
In conclusion, social robots are robots essential in day-to-day interactions that have started to rapidly grow and advance throughout society. They contain many crucial internal and external characteristics that are all essential to their design. In order to function, these robots often learn and act using AI. They also generally contain a central focus point and look recognisable to humans. As they continue to grow, they will bring countless benefits to an array of sectors, including hospitality, entertainment, and healthcare. Their wide range of usage will also help a variety of people ranging from those with autism to tourists in new countries.
1.3. Design Principles of Social Robots
Since there are hundreds of robots with varying properties and purposes, there is no single way in which social robots can be designed to look or to interact with humans. However, their design is a main factor in their efficacy in interacting with humans and is therefore an essential consideration when developing socially intelligent robots.
1.3.1. Physical Structure
Many social robots are designed based on human features and feedback. Kalegina et al. (2018) argue that facial features are imperative for human survival, as they are used to infer emotion, intention, and personality traits. Kalegina et al. (2018) found a number of factors that influence the perception of traits, including friendliness, trustworthiness, and intelligence. Reeves, Hancock, and Liu (2021) extended this research from a psychological perspective by evaluating the impressions that humans have of robots and how humans perceive warmth and competence. They concluded that a mix of human and robotic attributes evoked a greater sense of warmth, as well as the appearance of a robot being young (Reeves, Hancock, and Liu, 2021). Furthermore, the robot’s perceived mobility proved to have had a great influence on human judgments. The authors speculate that this could be due to the fact that greater degrees of mobility are believed to engender greater capabilities in the roles robots could play in the future (Reeves, Hancock, and Liu, 2021). These factors are hereby important to consider when designing robots in order for them to be well suited for their roles.
When considering the physical appearance of robots, the extent to which robots should resemble humans is put in to question. DiSalvo et al. (2002) suggest that certain features should remain robotic to highlight their limitations during HRI. As these abilities become more human-like, robots also become more human-like in their physical appearances. For example, researchers, such as Takeuchi and his colleagues at the University of Tokyo, have been developing increasingly realistic materials for robots to mimic the feel and appearance of human skin (Kawai et al., 2021). In light of these conflicting examples, the effects of these more humanistic design features on HRI and the extent to which they should be used is an aspect that needs to be considered.
Japanese roboticist, Masahiro Mori (2012), theorised a graph which mapped the relationship between humans’ affinities to robots and their human likeness (Figure 6). In his original 1970 essay, he argues that positive affinity to robots increases with their human resemblance, up until robots achieve a convincing but not quite life-like appearance. At this point, human perceptions drop into an uncanny valley where their reactions change from empathetic to repulsed. Thus, when designing robots, Mori suggests that there should be a limit on a robot’s human likeness to improve HRI so that humans react positively to robots. Support for this theory can be found in Kalengina et al.’s (2018) paper that showed more dislike for the human-like faces of robots, with participant feedback calling them “creepy”.
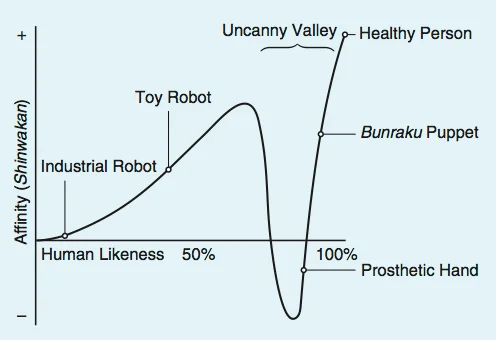
Figure 6: The graph depicts the uncanny valley, the proposed relation between the human likeness of an entity and the perceiver’s affinity for it (Mori, 2012, sec: A Valley in One’s Sense of Affinity).
1.3.2. Manner of Communication (Verbal/Non-Verbal)
When designing socially intelligent robots, it is important to consider how they will communicate in order to interact with humans. By communicating both verbally and non-verbally, humans will be able to better understand the robot, and thus naturally respond to the robot as if it were another person. Research conducted by Onyeulo and Gandhi (2020) has shown that robots do not need to speak a verbal language in order to interact with humans. However, if there is no verbal language, non-verbal conversational cues, including facial expressions, raising of the eyebrows, and vocal babbles, are necessary for interaction (Onyeulo & Gandhi, 2020). Furthermore, Onyeulo and Gandhi (2020) elaborate, affirming that if a robot is to speak, they need to be able to maintain conversations, pause naturally between talking, and flexibly change the rhythm and tempo of the words, based on cues by humans. Therefore, for the most effective HRI, social robots should have the capability to communicate with humans in fully understandable ways through a combination of verbal and non-verbal cues.
1.3.3. Coding/Programming Structure
Elaborating on Section 2 regarding the role of AI in social robotics, since the code behind the robots controls how they function, designing their programs appropriately is key to enhancing HRI. Often, social robots need to be programmed in order to function and contain intelligent and autonomous behaviours that can be continuously improved through experiences (Stange et al., 2022). As such, AI has played a key part in allowing robots to learn and adapt their behaviour to fit the interaction and environment. By using machine learning to improve based on past experiences, robots can learn and develop constantly, reducing the amount of time and effort to program such a complex machine (Soori et al., 2023). Reinforcement learning is one example of how computer models learn to identify patterns in data and produce optimal results by giving it example sets of data with inputs and their corresponding outputs. Measuring its efficacy is simple: testing it on a test set of data and evaluating how often it outputs the correct result (García-Martínez et al. 2024). For example, a robot could learn which factors provoke a smile after being trained on data where the outputs are different facial expressions.
On the other hand, for robots to perform specific functions such as listening, talking, or walking, different AI algorithms need to be incorporated. Natural language processing, for example, has given robots the capacity to both understand human language and infer the meaning of incomplete commands with high accuracy. In order to create appropriate responses for each situation, large language models (LLMs) are required to train robots on a vast amount of data that allows them to be able to converse in a seemingly natural way (Obaigbena et al., 2024). Speech recognition technologies then work hand in hand with these advances to give robots the ability to understand human voice inputs with different accents and languages (Soori et al., 2023).
For vision and manoeuvring, robots have sensors and cameras to retrieve information about the environment. Convolutional neural networks (CNNs) are optimal for analysing visual data and using it to make decisions. CNNs analyse visual data through layers of operations. These layers highlight important features like edges and textures, allowing the network to learn complex patterns. Their final output are class probabilities in image classification tasks, effectively allowing the CNN to recognise objects in the view of their cameras (O’Shea and Nash, 2015).
There are many more AI algorithms which social robots use, including deep learning algorithms and recurrent neural networks. For the variety of roles social robots take on, each individual type needs a programming algorithm and data that is tailored to the area in which the social robot is employed (healthcare, entertainment, etc.) (Diprose et al., 2016). Due to this, each social robot contains a different programming structure and uses specific AI algorithms in order to be employed effectively.
In conclusion, the development of social robots requires a holistic consideration of multiple design principles such as physical appearance, programming, and manner of communication. As a result of the diversity of these combinations, social robots can be designed to take on varied roles in human lives, each with a different impact on society.
2. The Impacts of Social Robots
The rapid integration of social robots and HRI into various sectors of the economy is poised to transform industries, bringing about significant economic implications. This section explores the potential for job displacement resulting from this technological advancement, examining both the positive impacts on efficiency and productivity, as well as the potential negative consequences for employment and wages.
2.1. Economic Implications
The integration of social robots and HRI into various sectors of the economy has significant implications. Social robots have the potential to transform industries by improving efficiency, enhancing customer service, and reducing labour costs. For instance, in healthcare, social robots can assist in patient care, providing companionship and performing routine tasks, thus allowing healthcare professionals more time to focus on more complex duties (SpringerLink, 2021). This can lead to cost savings and improved patient outcomes, addressing labour shortages in the sector.
In the service industry, social robots can perform roles such as customer service representatives, concierges, and even retail assistants, which can streamline operations and reduce overhead costs (International Journal of Social Robotics, 2021). These robots can handle repetitive and mundane tasks, allowing human employees to engage in more value-added activities, potentially boosting productivity and job satisfaction.
The deployment of social robots also has broader economic benefits by fostering innovation and creating new market opportunities. The development, production, and maintenance of these robots require a skilled workforce, potentially generating new jobs in robotics engineering, programming, and maintenance (SpringerLink, 2021). Moreover, by improving efficiency and productivity across various sectors, social robots can contribute to overall economic growth. Although technology has brought new work opportunities, robots have increased productivity and reduced labour demand. Their insertion into the workforce has disproportionately affected lower and middle-income earners, particularly in routine manual occupations. Research conducted by MIT’s Daron Acemoglu and Boston University’s Pascual Restrepo (2020) has underscored this duality, therefore cautioning that the future impact of robots on employment and wages is uncertain and could vary as the technology becomes more widespread. However, their research also found that for every robot added per 1000 workers in the U.S., wages declined by 0.42% and the employment-to-population ratio by 0.2%, translating to about 400,000 lost jobs. This displacement is emphasised in areas with high robot deployment, and can expect to grow with direct proportionality to its advancement (Acemoglu & Restrpo, 2020).
It is often reflected in the media and popular business press that there is widespread anxiety concerning the possibility of workplace displacement by increasingly socially intelligent robots. However, a study conducted by Dahlin (2019) shows that this fear might be exaggerated since regression models show that robots are not displacing workers in the metropolitan areas examined. On the other hand, robots positively impact high-skill jobs and complement middle-skill jobs by working alongside humans. There have been some discrepancies with previous findings, such as those of Acemoglu and Restrepo (2020), but these may be due to the recent rise in manufacturing occupations, suggesting that repetitive manual labour has been automated, and that more complex jobs engender human-robot collaboration. Therefore, while automation has historically broadly displaced jobs, it may herald a new wave of HRI and coexistence (Dahlin, 2019).
2.2. Social and Cultural Impacts
The integration of migrant children into new educational systems is a significant social and cultural challenge, especially when considering their academic performance hindered by language barriers. Despite a strong motivation to learn, these students often find themselves lagging behind their native peers in literacy and overall academic achievement (OECD, 2021). In this context, the deployment of educational robots emerges as a viable technological solution to provide language support (Louie et al., 2022). Furthermore, research indicates that cultural factors significantly influence the acceptance of robots, as seen in differing responses between Chinese and Iranian individuals (Abdollahi & Alemi, 2021). This cultural variability suggests that for educational robots to be effective, technologists and designers must consider and adapt to cultural differences, creating robots that are not only functional but also culturally sensitive. Integrating such innovative solutions can enhance the educational experience for migrant children, fostering a more inclusive and supportive learning environment.
To conclude, the integration of social robots and HRI into the economy presents a dual-edged sword. While it promises increased efficiency, innovation, and economic growth, it also poses significant challenges related to job displacement and wage suppression, particularly for lower and middle-income workers. As we move forward, it is crucial to develop strategies that maximise the benefits of robotic integration while mitigating its adverse impacts on the workforce.
3. Challenges of Social Robots
Although socially intelligent robots have the potential to improve society and help those in need of social and emotional support, there are also many potentially harmful drawbacks and challenges. According to Lutz (2019), there are many ethical, legal, and social issues of social robots. This section covers four of the main categories of challenges that Lutz (2019) lists: (1) Privacy and security issues, (2) legal uncertainty, (3) extent of autonomy, and (4) the replacement of human to human interactions. While these broad categories may cover many key challenges of social robotics, there are numerous other issues that can occur with such robots, encompassing topics such as trust, job replacement, safety, cost, and socio-economic difficulties as described in Section 2 (Boada et al., 2021).
The ethical implications of integrating social robots and HRI into society are complex and multifaceted. As robots become more integrated into social settings, they can influence and reshape human behaviours, responsibilities, and interactions – a concept known as “technical mediation”. This can lead to significant changes in societal norms and values (International Journal of Social Robotics, 2021). In order to overcome these challenges, collaborative efforts from a diverse range of fields are needed in order to provide important insight to solving the multitude of problems that social robots may contain (Obaigbena et al., 2024).
3.1. Privacy and Security
Amid the growing use of social robots, privacy and security have continued to be a huge concern. As part of their functionality, social robots often gather extensive arrays of data, such as voice, images, and profile information, for accurate HRI (Subramanian, 2017). Robots are therefore equipped with an array of sophisticated sensors and processors. However, the collection of data brings major risks of hacking and data breaches (Subramanian, 2017). If the data collected by social robots is not properly secured, it can be accessed by unauthorised parties, leading to identity theft, fraud, ransom attacks, and other malicious activities. Moreover, corporate firms may retain the rights to store this data on their servers and repurpose it in ways that raise ethical concerns (Vulpe, 2019).
An existing example is when firms sell user preferences to advertising companies for a profit. This is a significant privacy concern as it can lead to unauthorised sharing of sensitive personal information, increased surveillance, and potential misuse of data without the individual’s informed consent (Lutz, 2019). Thus, companies must be transparent about their data collection practices and ensure compliance with privacy laws to build trust with users (Harkison and Mooney, 2021). This still poses great challenges for the development of social robots as the success of their companionship hinges on human trust (Dautenhahn, 2007).
Stemming from this issue is the potential for discrimination and bias. Robots, being designed and programmed by humans, may inadvertently perpetuate existing racial or gender biases, for example. This challenge stems from the data the robots are trained on, raising further questions about the sources of data used. For example, Microsoft’s Tay chatbot, originally aimed to partake in casual conversation with 18 to 24-year-olds, rapidly picked up racist and offensive language after learning from various Twitter users who used similar language (Hunt, 2016). Consequently, it caused controversy ending with Tay being quickly shut down. In contexts such as law enforcement or customer service, where biased behaviour can have severe consequences, this would have been much more problematic and could be dangerous to those historically discriminated against in society (Michaud, 2021). Therefore, it is important to ensure that these robots are as fair and unbiased as possible before their launch into society.
3.2. Legal Uncertainty
Although social robots have started to integrate, Fosch‑Villaronga et al. (2019) explain the lack of general formulation of liability in cases of robot accidents. In instances of incorrect algorithmic predictions by robots, including misperception and deception, accountability will fall in limbo. Therefore, several legal and economic scholars across the world have argued for the need to rethink legal remedies to achieve meaningful HRI (Fosch‑Villaronga et al., 2019). In light of the legal ambiguities surrounding social robots, comprehensive and universal legislation are necessary to resolve any uncertainty.
3.3. Autonomy and Agency
As robots become more autonomous, one of the biggest concerns in the use of social robots is the manner and extent to which they make decisions. According to Fosch‑Villaronga et al. (2019), many people question if robots should be able to override human decisions due to their greater knowledge on certain topics. This is reinforced by robots’ ability to process information faster and without human emotions, potentially leading to more rational decisions. However, this lack of human emotion limits robots from a sound ethical framework which is necessary in critical decision making. Consequently, the autonomy of robots is widely debated. Furthermore, there will be a lack of accountability since determining who is responsible for the actions of robots is complex. Ethical frameworks need to address this ambiguity between the responsibility of robots and their creators, ensuring that any harm caused by robots is traceable and appropriately dealt with (Michaud, 2021).
3.4. Replacement of Human-to-Human Interactions
The overuse of social robots in human interactions, especially for those still in developmental stages of life (babies and children), may cause harm to human-to-human social interactions, raising ethical concerns. According to Sharkey and Sharkey (2020), social robots can often be deceiving due to their perceived ability to show emotions. Due to the popularity of the human-like emotional nature of social robots among children, many parents may leave their children alone in the hands of these machines for hours on end, which may cause such children to gain emotional attachments to these robots (Sharkey and Sharkey, 2020). This overtrust in and overexposure to these robots will cause many of these children to believe such machines can express real emotions and actually care for humans (Turkle, 2017). In reality, a robot’s emotions have no depth or true understanding and therefore robots do not actually feel anything for a human, only acting as if they do (Hirsch, 2024). As some parents allow their children to overuse such robots, they do not gain necessary social benefits from interactions with their children.
In addition, these social robots may also change the way people act, making them antisocial and narcissistic. Since social robots are taught to be customised to the needs of each individual, they often do not have conflicting views with each human (Sharkey and Sharkey, 2020). Attributable to this, some vulnerable people may reject all human-to-human interaction, which may contain argumentative views, and opt for a lifestyle socialising with a robot that often will not disagree with them (Sharkey and Sharkey, 2020). These vulnerable individuals may never learn the true nature of human relationships which will cause detrimental effects on their social life, often causing them to become antisocial and self-centred. Furthermore, while robots can alleviate loneliness and provide companionship, this can lead to the dehumanisation of users and a reduction in genuine human interactions. The ethical dilemma here revolves around whether it is morally acceptable to replace human contact with robotic interaction, potentially leading to a society where human social skills and emotional connections are diminished (Harkison and Mooney, 2021).
The displacement of many jobs by robots will also replace human-to-human interactions. Since social robots will replace the jobs of people in sectors including travel, tourism, education, healthcare, retail, entertainment, and hospitality (Pandit Vishwakarma et al., 2024), many regular interactions that exist today will disappear.
Overall, the overuse of and overtrust in social robots can have detrimental impacts on social interactions between humans. Although there are multitudes of problems that can stem from these robots, ethical, legal, and social issues have been the most prominent (Beetz et al., 2023). Such issues can be broken down into concerns about privacy and security, legal uncertainty, autonomy and agency, and the replacement of human-to-human interactions. While robots may not see any harm in their actions, they may result in detrimental consequences and challenges with responsibility and course of action. As social robots continue to diffuse throughout society, improving their functionality and making regulatory laws are especially important in order to prevent any potential destruction that may be caused by these robots (Sokolov and Kuperman, 2024).
4. Future of Social Robots
The current use of social robots spans across various sectors, including healthcare and customer service, which signals a transformative future where HRI has the potential to become increasingly integral to everyday life. This section explores how these robots, enhanced by AI, are designed to adapt to user interactions, emotions, and preferences, providing personalised and effective support. The development of soft robotics and sustainable material also showcases the innovations being made in this field. As these continue, there is great potential for social robots to improve quality of life and redefine human interactions.
4.1. Emerging technologies and their impacts
The materials for the robot market are forecasted to grow to 89 billion by 2029, driven by increased investments and innovative developments (IndustryArc, 2024). In addition to cutting-edge bio-inspired materials, sustainable options are gaining a greater presence in this field, especially as electronic waste is being recognised as a major problem of the 21st century. For example, in 2023, LG Chem created a lightweight, walking aid robot out of environmentally friendly plastic (IndustryArc, 2024). Moreover, a key area of development in robotic materials is soft robotics. These materials are soft, and material scientists have already been able to employ them to design parts of fragile objects, perform microscopic tasks, and generate control motion without electronics. Though this technology has potential, it has yet to reach it. Rothemund et al. (2021) highlight two main challenges that soft materials face: firstly, technological limitations have not gained commercial attraction; secondly, the issue of creating a suitable, reliable interface between functional soft robotic parts and their environment.
AI has the potential to further enhance social intelligence in robots and improve HRI (Rothemund et al., 2021). Increasingly advanced natural language processing will transform the way social robots interact, incorporating more contextual details into robots’ verbal communication (Khurana et al., 2022). In addition, deep learning and behavioural modulation will improve social robot’s non-verbal communication, allow them to adapt to individual use preferences, and give personal and appropriate responses (Spezialetti, Placidi, & Rossi, 2020). However, as the development of AI progresses, we are met with several ethical questions that should be addressed before the widespread use of social robots, as discussed in Section 3. For example, the extent of a robot’s autonomy and agency when there are still risks concerned with the abilities of AI.
4.2. Future Research Areas
Future research areas for the integration of social robots and HRI hold immense potential for advancing both technology and societal benefits. One rising area is the development of adaptive social robots that can modify their behaviour based on user interactions, emotions, and preferences. These robots can enhance user experience by providing more personalised and effective interactions, which is crucial for their long-term applicability in various social domains such as education, healthcare, and customer service (Ahmad et al., 2017).
Another key research direction is exploring the emotional design of social robots. Understanding and implementing emotional expressions in robots can significantly improve user acceptance and engagement, making interactions more natural and meaningful. This involves studying human emotional responses and integrating sophisticated emotional intelligence into robotic systems (Chen et al., 2023).
Again, the ethical implications of deploying social robots raised in Section 3 also warrant extensive research. Issues such as privacy, data protection, and the moral responsibilities of robots need to be thoroughly examined. Developing frameworks to address these concerns is essential to ensure the ethical use of robots in society, particularly as they become more autonomous and capable of making decisions (Frontiers in Robotics and AI, 2021).
Additionally, investigating the role of social robots in education and therapy offers exciting possibilities. Robots can serve as tutors or therapeutic aids, providing tailored support to students and patients. Research in this area can focus on optimising the interaction design and effectiveness of robots in these roles, ensuring they complement human efforts rather than replace them (Sensors, 2023).
The cultural dimensions of HRI are also a critical research area. Understanding how different cultures perceive and interact with robots can inform the design of culturally adaptive robots, enhancing their acceptance and usability worldwide (Lim et al., 2020).
4.3. Long-term Relationships/Evolution of These Interactions
When considering the advent of social robots, we need to consider the reception people will have towards them. One of the settings these robots could be employed in is education, engaging students. In a 2014 research workshop in Sweden involving 45 students, Serholt and Barendregt (2014) found that the students’ perceptions of robots were overall positive, but only to the extent that they should be recognised as solely tools without agency to make big decisions like assigning grades. They recorded scepticism regarding robot assistants for pre-school children as students had fears that the young children wouldn’t just view the robots as “useful tools.” However, they recognised the importance of real-life experiences in building up trust towards robots (Serholt and Barendregt, 2014).
Furthermore, different applications of social robots might have nuanced design principles. For robots operating in the care system, Jordan Miller of Arizona University suggested features such as facial recognition capabilities and secure cybernetworks to improve robots’ security and abilities to communicate effectively (Miller, 2022).
In conclusion, the future of social robots reflects both opportunities and challenges. As these technologies advance, it is crucial to address ethical implications such as privacy, data protection, and moral implications. Continued research into adaptive behaviours, emotional design, and cultural adaptability will be essential to enhance user acceptance and effectiveness. The future of social robots hinges on balancing technological innovation with ethical responsibility, ensuring they complement human efforts and contribute to society positively.
5. Conclusion
Social robots epitomise a key emerging technology which holds the potential to both transform day-to-day life throughout the world and begin a new era of profound development in all robots (Breazeal, 2009). The rapid growth of AI over the past few years has already altered the course of upcoming human advancements and social robots represent central entities in the transformation of AI into physical bodies (Kalotra, 2023). Furthermore, the use of social robots in HRI is currently changing the landscape of pervasive areas including healthcare, hospitality, therapy, education, and customer service (Biba, 2024).
Currently, these robots can be used by people of all ages, gender, culture and race as they are built to adapt to their environment and are able to personalise user experience (Recchiuto & Sgorbissa, 2022). As their use continues to grow throughout the world, these robots might bring powerful benefits to society, including education for those with autism, companionship for the elderly, stress reduction, therapy for people with mental disorders, personalised guides in tourism, and faster and longer customer service (Cifuentes et al., 2020; Tolle et al., 2023). However, these robots still have many challenges and concerns. Some of these drawbacks include human job loss, lack of privacy and security, legal concerns, worries with regards to the extent of their autonomy, and potential replacement of human-to-human interactions (Lutz, 2019).
Further research on ethical restrictions, regulatory laws, and potential dangers is needed in order to combat these obstacles. Furthermore, in order to improve these robots, research on improving AI integration, the emotional design of robots, the cultural aspect of environments, and the sectors that such robots can be used in, is necessary. According to Mahdi et al. (2022), by reflecting on the past, improving on the functionality of previous robots, and using creative exploration to find new solutions to difficult problems, we can further enhance the development and design of forthcoming social robots, and take one step closer to unlocking a robot’s full potential.
In conclusion, social robots foreshadow and symbolise a revolutionary change to HRI and its coordination with AI. With the aim of improving all interactions, especially for those in need of emotional support, social robots have the capability to change all of life. By continuing their development, reducing their challenges, and improving their efficacy, humans can create pivotal changes in the direction of the world’s robotic technology, allowing for social robots to enrich the world.
Bibliography
Abdollahi, B. and Alemi, M. (2021) ‘A Cross-cultural Investigation on Attitudes Towards Social Robots: Iranian and Chinese University Students,’ Journal of Higher Education Policy And Leadership Studies, 2(3), 120-138. Available at: https://johepal.com/article-1-132-en.html (Accessed: 21 July 2024)
Acemoglu, D. and Restrepo, P. (2020) ‘Robots and Jobs: Evidence from U.S. Labor Markets,’ Journal of Political Economy, 128(6). Available at: https://shapingwork.mit.edu/wp-content/uploads/2023/10/Robots-and-Jobs-Evidence-from-US-Labor-Markets.p.pdf (Accessed: 21 July 2024)
Ahmad, M. I., Mubin, O., and Orlando, J. (2017). ‘Adaptive social robots in education,’ International Journal of Social Robotics, 9(1), 51-60.
Barajas-Lopez, F. (2009). Language Barriers in Education. Retrieved from ResearchGate.
Barten, M. (2019) 8 Examples of Robots Being Used in the Hospitality Industry. Available at: https://www.revfine.com/robots-hospitality-industry/ (Accessed: 21 July 2024).
Beetz, M., Engel, U., Hoyer, N., Lorenz Kähler, Langer, H., Schultheis, H., and Straube, S. (2022) ‘Trustworthiness and Well-Being: The Ethical, Legal, and Social Challenge of Robotic Assistance’, in S. Chatterjee (ed.) SpringerBriefs in Sociology. Springer International Publishing, pp. 1–26. Available at: https://link.springer.com/chapter/10.1007/978-3-031-11447-2_1 (Accessed: 28 July 2024).
Biba, J. (2024) ‘What is a social robot?’, Built In. Available at: https://builtin.com/robotics/social-robot (Accessed: 20 July 2024).
Boada, J.P., Maestre, B.R., and Genís, C.T. (2021) ‘The ethical issues of social assistive robotics: A critical literature review’, Technology in Society, 67, 101726. Available at: https://www.sciencedirect.com/science/article/pii/S0160791X21002013 (Accessed: 27 July 2024).
Breazeal, C. (2000). ‘Kismet: Overview.’ [online] web.media.mit.edu. Available at: https://web.media.mit.edu/~cynthiab/research/robots/kismet/overview/overview.html (Accessed: 21 July 2024).
Breazeal, C. (2009) ‘Role of expressive behaviour for robots that learn from people’, Philosophical Transactions of the Royal Society B: Biological Sciences, 364(1535), 3527–3538. Available at: https://www.ncbi.nlm.nih.gov/pmc/articles/PMC2781892/ (Accessed: 24 July 2024).
Bristol, U. of (2008). ‘2008: Grey Walter and His Tortoises.’ [online] www.bristol.ac.uk. Available at: https://www.bristol.ac.uk/news/2008/212017945378.html (Accessed: 21 July 2024).
Burgess, M. (2017). ‘Rebuilding Eric, ‘the Man without a soul’: the UK’s First Robot Gets an Upgrade.’ [online] Wired. Available at: https://www.wired.com/story/eric-robot-science-musuem/ (Accessed: 26 July 2024).
Chen, J., Lin, Y., and Wang, Z. (2023). ‘Emotional design in social robots.’ Sensors, 23(6), 2891.
Cifuentes, C.A., Pinto, M.J., Céspedes, N., and Múnera, M. (2020) ‘Social Robots in Therapy and Care’, Current Robotics Reports, 1, 59–74. Available at: https://link.springer.com/article/10.1007/s43154-020-00009-2 (Accessed: 25 July 2024).
Cox, M. (2022) Meet Orbit, the Interactive Robot That Looks to Help Children With Autism Spectrum Disorders Develop Social Skills, Neuroscience News. Available at: https://neurosciencenews.com/asd-orbit-social-robot-21497/ (Accessed: 24 July 2024).
Cyberneticzoo.com (2009) 1928 – Eric Robot – Capt. Richards & A.H. Reffell (English). Available at: https://cyberneticzoo.com/robots/1928-eric-robot-capt-richards-english/ (Accessed: 28 July 2024).
Dahlin, E. (2019). ‘Are Robots Stealing Our Jobs?,’ Socius, 5. Available at: https://journals.sagepub.com/doi/full/10.1177/2378023119846249#sec-4 (Accessed 21 July 2024).
Darrisaw, M. (2019) This New Robotic Dog Provides Companionship to Elders. Available at: https://www.oprahdaily.com/life/health/a27286768/tombot-puppy-jennie-robotic-dog/ (Accessed: 25 July 2024).
Dautenhahn, K. (2007) ‘Cooperation and competition in group knowledge – the role of positive emotions, Socially intelligent robots: dimensions of human–robot interaction,’ Philosophical Transactions of the Royal Society B, 362(1480). Available at: https://royalsocietypublishing.org/doi/abs/10.1098/rstb.2006.2004 (Accessed: 28 July 2024).
Dewaele, J. (2019). Multilingualism and Education. Retrieved from JSTOR.
Digitale, E. (2023) ‘Brain wiring explains why autism hinders grasp of vocal emotion, says Stanford Medicine study’, Stanford Medicine | News Center, 29 January. Available at: https://med.stanford.edu/news/all-news/2023/01/brain-autism-speech-emotion.html (Accessed: 27 July 2024).
Diprose, J., MacDonald, B., Hosking, J., and Plimmer, B. (2017). ‘Designing an API at an appropriate abstraction level for programming social robot applications,’ Journal of Visual Languages and Computing, 39, 22-40. Available at https://researchspace.auckland.ac.nz/bitstream/handle/2292/35060/diprose-designing-jvlc-2016.pdf?sequence=41&isAllowed=y (Accessed: 17 July 2024).
Disalvo, C., Gemperle, F., Forlizzi, J., and Kiesler, S. (2002) ‘All Robots Are Not Created Equal: The Design and Perception of Humanoid Robot Heads,’ Proceedings 4th Conference on Designing Interactive Systems: Processes, Practices, Methods, and Techniques. Available at: https://www.researchgate.net/publication/221441291_All_robots_are_not_created_equal_The_design_and_perception_of_humanoid_robot_heads (Accessed: 16 July 2024).
Dronkers, J. and De Heus, M. (2016). Migrant Integration in Europe and the US. Retrieved from SpringerLink.
Fosch-Villaronga, E., Lutz, C., and Tamò-Larrieux, A. (2019) ‘Gathering Expert Opinions for Social Robots’ Ethical, Legal, and Societal Concerns: Findings from Four International Workshops’, International Journal of Social Robotics, 12. Available at: https://doi.org/10.1007/s12369-019-00605-z (31 July 2024).
Frontiers in Robotics and AI. (2021). ‘Ethical implications of social robots,’ Frontiers in Robotics and AI, 8, 56.
García-Martínez, J., Carlos Castillo, J., Marqués-Villaroya, S., and Angel Salichs, M. (2023) ‘Active Object Learning for intelligent social robots’, Engineering Applications of Artificial Intelligence, 127(Part B), 107329–107329. Available at: https://www.sciencedirect.com/science/article/pii/S0952197623015130 (Accessed: 18 July 2024).
González-González, C.S., Violant-Holz, V., and Gil-Iranzo, R.M. (2021) ‘Social Robots in Hospitals: A Systematic Review’, Applied Sciences, 11(13), 5976. Available at: https://www.mdpi.com/2076-3417/11/13/5976 (Accessed: 20 July 2024).
Handwerk, B. (2022) ‘How Humanlike Do We Really Want Robots to Be?’, Smithsonian Magazine. Available at: https://www.smithsonianmag.com/science-nature/how-humanlike-do-we-really-want-robots-to-be-180980234/ (Accessed: 16 July 2024).
Harkison, T. and Mooney, S. (eds) (2024) Hospitality Insights. Available at: https://ojs.aut.ac.nz/hospitality-insights/ (Accessed: 30 July 2024).
Hirsch, K. (2024) Why Robots Will Never Experience Emotions: An Informed Perspective. Available at: https://www.linkedin.com/pulse/why-robots-never-experience-emotions-informed-karl-hirsch-udp8c#:~:text=According%20to%20a%20report%20by (Accessed: 25 July 2024).
Hunt, E. (2016). ‘Tay, Microsoft’s AI chatbot, Gets a Crash Course in Racism from Twitter,’ The Guardian, 24 March. Available at: https://www.theguardian.com/technology/2016/mar/24/tay-microsofts-ai-chatbot-gets-a-crash-course-in-racism-from-twitter (Accessed: 28 July 2024).
IndustryArc (2024). Materials for Robots Market – Forecast(2024 – 2030). Available at: https://www.industryarc.com/Research/materials-for-robots-market-research-800625?utm_source=article&utm_medium=Social&utm_campaign=AlekhyaD&trk=article-ssr-frontend-pulse_little-text-block (Accessed: 19 July 2024)
International Journal of Social Robotics (2021). ‘Cultural dimensions of HRI,’ International Journal of Social Robotics, 13(3), 447-459.
ISO – International Organization for Standardization. (2021). ISO 8373:2021(en) Robotics – Vocabulary. Available at: https://www.iso.org/obp/ui/#iso:std:iso:8373:ed-3:v1:en (Accessed: 24 July 2024).
Jonell, P., Mendelson, J., Storskog, T., Hagman, G., Leite, I., Kucherenko, T., Mikheeva, O., Akenine, U., Jelic, V., Solomon, A., Beskow, J., Gustafson, J., Kivipelto, M., and Kjellström, H. (2017) Machine Learning and Social Robotics for Detecting Early Signs of Dementia. Available at: https://people.kth.se/~hedvig/EACare/eacare_positionpaper_2017.pdf (Accessed: 17 July 2024).
Jozuka, E. (2016). ‘The Sad Story of Eric, the UK’s First Robot Who Was Loved Then Forsaken,’ [online] www.vice.com. Available at: https://www.vice.com/en/article/pgkkpm/the-sad-story-of-eric-the-uks-first-robot-who-was-loved-then-forsaken (Accessed: 21 July 2024).
Kalegina, A., Schroeder, G., Allchin, A., Berlin, K., and Cakmak, M. (2018) ‘Characterizing the Design Space of Rendered Robot Faces’, Human-Centered Robotics Lab [Preprint]. Available at: https://hcrlab.cs.washington.edu/assets/pdfs/2018/kalegina2018hri.pdf (Accessed: 16 July 2024).
Kalotra, S. (2023) AI-Powered Social Robots: Enhancing Human-Robot Interaction. Available at: https://www.signitysolutions.com/tech-insights/ai-powered-social-robots (Accessed: 25 July 2024).
Khurana, D., Koli, A., Khatter, K. and Singh, S. (2022). Natural Language processing: State of the art, Current Trends and Challenges. Multimedia Tools and Applications, 82(3), pp.3713–3744. Available at: doi:https://doi.org/10.1007/s11042-022-13428-4 (Accessed: July 2024).
Knox, B., Stone, P., and Breazeal, C. (2013) ‘Training a Robot via Human Feedback: A Case Study. In Proceedings of the International Conference on Social Robotics (ICSR).’, MIT Media Lab. Available at: https://www.media.mit.edu/publications/training-a-robot-via-human-feedback-a-case-study-in-proceedings-of-the-international-conference-on-social-robotics-icsr/ (Accessed: 20 July 2024).
Koglbauer, R. (2018). Education Systems and Migrant Students. Retrieved from Oxford Academic.
Korn, O., Akalin, N., and Gouveia, R. (2021) ‘Understanding Cultural Preferences for Social Robots’, ACM Transactions on HRI, 10(2), 1–19. Available at: https://dl.acm.org/doi/fullHtml/10.1145/3439717 (Accessed: 15 July 2024).
LeBouthillier, A. (1999). ‘W. Grey Walter and his Turtle Robots,’ [online] home.csulb.edu. Available at: https://home.csulb.edu/~wmartinz/content/w-grey-walter-and-his-turtle-robots.html (Accessed: 21 July 2024).
Lim, V., Rooksby, M., and Cross, E.S. (2020). ‘Social Robots on a Global Stage: Establishing a Role for Culture During Human–Robot Interaction,’ International Journal of Social Robotics, 13(1), 1307-1333. Available at: https://link.springer.com/article/10.1007/s12369-020-00710-4 (Accessed: 21 July 2024).
Loughborough University (2022) ‘Meet Orbit, the Interactive Robot That Looks to Help Children With Autism Spectrum Disorders Develop Social Skills,’ Loughborough University, 20 September. Available at: https://www.lboro.ac.uk/news-events/news/2022/september/orbit-robot-student-design-project/ (Accessed: 25 July 2024).
Louie, B., Björling, E.A., Kuo, A.C., and Alves-Oliveira, P. (2022) ‘Designing for culturally responsive social robots: An application of a participatory framework’, Frontiers in Robotics and AI, 9. Available at: https://doi.org/10.3389/frobt.2022.983408 (Accessed: 21 July 2024).
Lutz, C. (2019) ‘The privacy implications of social robots: Scoping review and expert interviews,’ Special Issue: Mobile Media Beyond Mobile Phones, 7(3). Available at: https://journals.sagepub.com/doi/abs/10.1177/2050157919843961 (Accessed: 28 July 2024).
Lutz, C. (2019) The key challenges of social robots. Available at: https://www.hiig.de/en/the-key-challenges-of-social-robots/ (Accessed: 17 July 2024).
Mahdi, H., Akgun, S.A., Saleh, S., and Dautenhahn, K. (2022). ‘A Survey on the Design and Evolution of Social Robots – Past, Present and Future,’ Robotics and Autonomous Systems, 156, 104193. Available at: https://doi.org/10.1016/j.robot.2022.104193 (Accessed: 28 July 2024).
Michaud, F. (ed.) (2021) Current Robotics Reports. Available at: https://link.springer.com/journal/43154 (Accessed: 31 July 2024).
Miller, J. (2022). ‘Secure cybernetworks and facial recognition in care robots.’ Arizona University.
Mori, M. (2012) ‘The Uncanny Valley: The Original Essay by Masahiro Mori’, IEEE Spectrum. Available at: https://spectrum.ieee.org/the-uncanny-valley (Accessed: 16 July 2024).
Nolfi, S. (2021) Behavioral and Cognitive Robotics: An Adaptive Perspective. Available at: https://www.researchgate.net/publication/351093674_Behavioral_and_Cognitive_Robotics_An_Adaptive_Perspective (Accessed: 28 July 2024).
Nolfi, S. (2021) The machina speculatrix of William Grey Walter (1953). Available at: https://www.researchgate.net/figure/The-machina-speculatrix-of-William-Grey-Walter-1953_fig3_351093674 (Accessed: 27 July 2024)
Nussey, S. (2021). ‘SoftBank Claims Pepper the Robot Still Alive despite Sales Dive,’ [online] Asia Financial. Available at: https://www.asiafinancial.com/softbank-claims-pepper-the-robot-still-alive-despite-sales-dive#:~:text=SoftBank%20propped%20up%20Pepper (Accessed: 26 July 2024).
O’Shea, K. and Nash, R. (2015). An Introduction to Convolutional Neural Networks. [online] Available at: https://arxiv.org/pdf/1511.08458 (Accessed: 28 July 2024).
Obaigbena, A., et al. (2024) ‘AI and HRI: A review of recent advances and challenges’, GSC Advanced Research and Reviews, 18(2), 321–330. Available at https://gsconlinepress.com/journals/gscarr/sites/default/files/GSCARR-2024-0070.pdf (Accessed: 15 July 2024).
OECD (2021). The Road to Integration – Education and Migration. Retrieved from European Website on Integration.
Onyeulo, E.B. and Gandhi, V. (2020). ‘What Makes a Social Robot Good at Interacting with Humans?’ Information, 11(1), 43. Available at: https://www.mdpi.com/2078-2489/11/1/43 (Accessed: 11 July 2024).
Pandey, A.K. and Gelin, R. (2018), ‘A Mass-Produced Sociable Humanoid Robot: Pepper: The First Machine of Its Kind,’ IEEE Robotics & Automation Magazine, 25(3), 40-48. Available at: https://doi.org/10.1109/MRA.2018.2833157 (Accessed: 18 July 2024).
Pandit Vishwakarma, L., Kr Singh, R., Mishra, R., Demirkol, D., and Daim, T. (2024) ‘The adoption of social robots in service operations: A comprehensive review’, Technology in Society, 76(1), 102441. Available at: https://www.sciencedirect.com/science/article/abs/pii/S0160791X23002464#:~:text=Adoption%20of%20social%20robots%20in%20service%20operations%20is%20the%20focus,%2C%20hotel%2C%20and%20hospitality%20industries (Accessed: 19 July 2024).
Pulido, J. (2024). ‘The Evolution of Assistive Social Robots,’ [online] Inrobics. Available at: https://inrobics.com/en/the-evolution-of-assistive-social-robots/ (Accessed: 21 July 2024).
Recchiuto, C. and Sgorbissa, A. (2022) Diversity-aware social robots meet people: beyond context-aware embodied AI. Available at: https://arxiv.org/pdf/2207.05372 (Accessed: 28 July 2024).
Reeves, B., Hancock, J., and Liu, X. (2020) ‘Social robots are like real people: First impressions, attributes, and stereotyping of social robots.’, Technology, Mind, and Behavior, 1(1). Available at: https://tmb.apaopen.org/pub/mm5qdu5l/release/1 (Accessed: 16 July 2024).
Reilly, K. (2011). ‘From Automata to Automation: The Birth of the Robot in R.U.R. (Rossum’s Universal Robots).’ in Automata and Mimesis on the Stage of Theatre History. Palgrave Macmillan, London. https://doi.org/10.1057/9780230347540_6 (Accessed: July 2024).
Revfine.com (2023) Robots in the Hospitality Industry. Available at: https://www.revfine.com/robots-hospitality-industry/ (Accessed: 20 July 2024).
Richards Family Robot Archive (2021) Eric Robot 1928. Available at: http://www.richardsrobots.com/eric-robot.html (Accessed: 26 July 2024)
Rothemund, P., Kirkwood, D., and Zhu, J. (2021). ‘Soft robotics: Challenges and opportunities.’ Soft Robotics, 8(4), 508-518.
Serholt, S. and Barendregt, W. (2014). ‘Students’ perceptions of educational robots.’ Workshop on Educational Robots, Sweden.
Sharkey, A. and Sharkey, N. (2020) ‘We need to talk about deception in social robotics!’, Ethics and Information Technology, 23(3), 309–316. Available at: https://link.springer.com/article/10.1007/s10676-020-09573-9 (Accessed: 19 July 2024).
Sheridan, T.B. (2016) ‘Human–Robot Interaction’, Human Factors: The Journal of the Human Factors and Ergonomics Society, 58(4), 525–532. Available at: https://journals.sagepub.com/doi/pdf/10.1177/0018720816644364 (Accessed: 20 July 2024).
Sokolov, T. and Kuperman, O. (2024) ‘Navigating the Ethics of Social Robots’, Weizmann Institute of Science Education, 8 May. Available at: https://davidson.weizmann.ac.il/en/online/firefly/navigating-ethics-social-robots#:~:text=Social%20robots%20have%20the%20potential (Accessed: 28 July 2024).
Song, Y. and Luximon, Y. (2024) ‘When Trustworthiness Meets Face: Facial Design for Social Robots’, Sensors, 24(13), 4215. Available at: https://www.mdpi.com/1424-8220/24/13/4215 (Accessed: 16 July 2024).
Soori, M., Arezoo, B., and Dastres, R. (2023). ‘Artificial Intelligence, Machine Learning and Deep Learning in Advanced Robotics, a Review,’ Cognitive Robotics, 3(1), 54-70. Available at: https://www.sciencedirect.com/science/article/pii/S2667241323000113 (Accessed: 18 July 2024).
Spezialetti, M., Placidi, G., and Rossi, S. (2020). Emotion Recognition for Human-Robot Interaction: Recent Advances and Future Perspectives. Frontiers in Robotics and AI, 7. doi:https://doi.org/10.3389/frobt.2020.532279 (Accessed: July 2024).
SpringerLink (2021). Interactive Robotics: Legal, Ethical, Social and Economic Aspects. Retrieved from SpringerLink
Standard Bots (2024) What are teleoperated robots? Definition with examples. Available at: https://standardbots.com/blog/teleoperated-robots#:~:text=Some%20of%20the%20most%20well,operate%20autonomously%20to%20a%20degree (Accessed: 20 July 2024).
Stange, S., Hassan, T., Schröder, F., Konkol, J., and Kopp, S. (2022) ‘Self-Explaining Social Robots: An Explainable Behavior Generation Architecture for HRI’, Frontiers in Artificial Intelligence, 5. Available at: https://www.frontiersin.org/journals/artificial-intelligence/articles/10.3389/frai.2022.866920/full (Accessed: 27 July 2024).
Subramanian, R. (2017) ‘Emergent AI, Social Robots and the Law: Security, Privacy and Policy Issues,’ Journal of International Technology and Information Management, 26(3). Available at: https://scholarworks.lib.csusb.edu/jitim/vol26/iss3/4/ (Accessed: 28 July 2024).
Tarakli, I., Angelopoulos, G., Hellou, M., Vindolet, C., Abramovic, B., Limongelli, R., Lacroix, D., Bertolini, A., Rossi, S., Di Nuovo, A., Cangelosi, A. and Cheng, G. (2023) Proceeding of the 1st Workshop on Social Robots Personalisation At the crossroads between engineering and humanities (CONCATENATE), arXiv.org. Available at: https://arxiv.org/abs/2307.12777 (Accessed: 26 July 2024).
Thalmann, N.M. (2022). ‘Social Robots: Their History and What They Can Do for Us.’ in Werthner, H., Prem, E., Lee, E.A., and Ghezzi, C. (eds) Perspectives on Digital Humanism. Springer, Cham. Available at: https://doi.org/10.1007/978-3-030-86144-5_2 (Accessed: 15 July 2024).
Tolle, J., Piazza, A., Kaiser, C., and Schallner, R. (2023) ‘Decision Support in Tourism through Social Robots: Design and Evaluation of a Conversation-Based Recommendation Approach Based on Tourist Segments’, RecTour2023, 3568. Available at: https://ceur-ws.org/Vol-3568/paper6.pdf (Accessed: 25 July 2024).
Torresen, J. (2018). ‘A review of ethical perspectives on robots.’ Journal of AI Research, 61, 1-16.
Torres-Guzman, M. (2017). Academic Performance and Language Barriers. Retrieved from Academia.edu.
Turkle, S. (2017) ‘Why these friendly robots can’t be good friends to our kids’, The Washington Post, 7 December. Available at: https://www.washingtonpost.com/outlook/why-these-friendly-robots-cant-be-good-friends-to-our-kids/2017/12/07/bce1eaea-d54f-11e7-b62d-d9345ced896d_story.html (Accessed: 25 July 2024).
Vulpe, A. (2015) ‘IoT Security Approaches in Social Robots for Ambient Assisted Living Scenarios’, IEEE Xplore. Available at: https://ieeexplore.ieee.org/Xplore/home.jsp (Accessed: 28 July 2024)
Wakefield, J. (2021). ‘RIP Pepper robot? SoftBank ‘pauses’ Production.’ BBC News. [online] 29 Jun. Available at: https://www.bbc.com/news/technology-57651405 (Accessed: 26 July 2024).
Wang, J. and Liu, H. (2024) ‘Application of social entertainment robots based on neural network’, Entertainment Computing, 56, 100765. Available at: https://www.sciencedirect.com/science/article/abs/pii/S1875952124001526 (Accessed: 20 July 2024).
Zhenhua, Z. and Feng, G. (2024) ‘Application of social entertainment robots based on machine learning algorithms and the Internet of Things in collaborative art performances’, Entertainment Computing (Forthcoming in 2025), 52, 100784. Available at: https://doi.org/10.1016/j.entcom.2024.100784 (Accessed: 21 July 2024).