Abstract
The use of artificial intelligence in cancer imaging via radiology has the potential to set unprecedented benchmarks for innovation, productivity, and accuracy in the medical field. Deep learning artificial intelligence (AI) algorithms, which are increasingly used in conjunction with various imaging techniques, are particularly effective in assisting radiologists in identifying and examining cancerous cells in mammograms, MRIs, CT scans, and PET scans. The chance of making a mistake is reduced due to the suggestions for a second opinion, as well as unparalleled accuracy. AI can identify suspicious areas and even predict whether the tumour is likely to be benign or malignant, while at the same time providing doctors with a detailed treatment plan. This technological advancement makes it possible to carry out the analysis process much more effectively. AI will facilitate faster diagnosis of cancer and other interventions required in radiotherapy. This article explores the most recent advances in cancer scanning technology, which have been made possible by AI. In this report, we assess the influence of AI on the calibre and sufficiency of analysis. We offer insights into potential future applications in particular medical disciplines, financial advantages, and particular examples. We aim to demonstrate that AI is a crucial instrument for improving the efficiency of detecting and treating tumours. We hope that our paper can serve as a tool for researchers, clinicians, and policymakers in understanding the potential of AI in breast cancer care.
1. Introduction
Every year, breast cancer, which ranks as the most frequent cancer diagnosis among women, accounts for approximately one-tenth of all new cancer cases (Menon et al., 2024). It also remains the second leading cause of cancer-related deaths in women globally. According to Menon et al., numerous factors increase the likelihood of developing breast cancer, including gender, familial background, genetic mutations, exposure to radiation, obesity, and many others. The vast majority of breast cancers are sporadic; fewer than 10% of patients have a detectable genetic mutation. Mammography is often used as a screening tool for breast cancer (Menon et al,. 2024). The prognosis for people with this serious illness is improving thanks to ongoing studies and a deeper understanding (Mayo Clinic, 2024). The use of artificial intelligence (AI) has soared, with applications such as AI-enhanced mammography for breast cancer detection, which can help radiologists detect early signs of breast cancer (Menon et al., 2024). When implementing these strategies, it is important to consider various factors, such as social concerns, legal and ethical considerations, cost, and accessibility. Mammograms are a vital part of the healthcare system, as they facilitate the identification of breast cancer, and significantly affect society due to the rise in breast cancer cases. Artificial intelligence is on the verge of revolutionising the healthcare industry due to its unprecedented potential.
2. The Software of AI and Room for Improvements
Before we begin dissecting this topic, it is important to set the boundaries of the subject matter we will analyse. Kumar and Alen define AI as the use of computing systems to imitate critical thinking compared to the human mind (Kumar & Alen, 2021). They determine the scope of how AI can work cohesively with physicians and radiologists to benefit health systems and patient care alike. Computing systems and AI currently implemented in cancer screening utilise large data sets of information on the respective area that is suspected to have cancerous growth to make judgements from X-rays and scans to diagnose the patient. Since the technology is still in its early stages, issues such as image preprocessing, data management, and other factors need to be enhanced, thus a physician must look over the results and review them before updating the patient or moving toward preventative, if applicable, action (Kumar & Alen, 2021).
The benefits of such a system revolve around adapting and integrating AI in medical screening, cancer screening, and diagnosis in particular. This can result in improved efficiency in the workspace, fewer false positives or negatives via improved accuracy, and quick and reliable updates. Improved efficiency stems from work done in tandem between the radiologists and the AI model. This new system allows for cases to be completed in faster times, thus radiologists can take more cases throughout their working hours, ultimately increasing the chances of seeing patients and acting on cancer cases while the cancer is still benign and preventable (Kumar & Alen, 2021). The decrease in the number of false positives and negatives comes from the role AI plays in acting as a second opinion in the duo between radiologists and AI. Everyone makes mistakes and having AI provide a different, potentially more accurate, opinion could open up factors that radiologists had forgotten to consider, thus reducing the amount of false positives and negatives. Finally, the faster updates and diagnosis time between the physician and patient is a downstream effect that will be an addition to improved efficiency and effectiveness of AI in the workplace. AI’s accuracy cannot only provide an accurate reading, but due to AI’s powerful computing abilities, it can also provide a diagnosis in a matter of seconds. This factor, graded by the radiologist for confirmation, in total should reduce wait times for patients to receive their diagnoses. Overall, this new AI system boasts a fast, efficient, and accurate way to screen and diagnose patients suspicious of the formation of cancer (Kumar & Alen, 2021).
2.1 Personal Interpretation of Future Challenges
The main challenges that this new technology faces are bias and economic disparity, false positives and negatives, and the “black box” conundrum. The bias and economic disparity are not new concepts when it comes to the integration of AI in all facets of life. In the case of medicine, questions would arise such as: “how much would this technology cost to integrate?” and “how can we ensure that all health systems have some form of this technology when it comes into fruition?” These are questions developers and medical administrators need to consider, and solutions to these problems will ensure an equitable and equal medical system for all. Another main challenge to be faced is false positives and negatives. Although touched on previously, false positives and negatives are a double-edged sword in this stage. Since this AI technology is still in its infancy, its readings will not always be perfect. This is why the radiologist and the AI are meant to collaborate with other, but this is an issue that will be discussed later in this paper, where we explore the ways that developers can fix this system internally, either in the database or code. Finally, an interesting issue with AI that mass media has not been highlighting as often would be the black box conundrum. This is a case in which AI learns an aspect of the field or the system that the developers nor the beneficiaries are aware of. This proves to be a problem because we have no control over how dangerous this newly learnt fact is. This is another aspect that will be discussed in the following sections, as well as how developers and software engineers alike could tackle this dilemma.
2.2 Current Coding System in AI for Cancer Screening
Like any learning machine, the computing system needs to be taught or fed knowledge or, in the case of AI, data to formulate a response to the problems submitted by the user. The AI technologies mentioned have to deal with thousands of patients, if not millions if the model is commonly used and universal among various health systems. In the article, “Medical Diagnostic Systems Using Artificial Intelligence Algorithms”, the authors note how the process of advancing an AI model with ML is done and what steps take place. The system begins when the data is collected based on specific needs and the variables necessary to create a proper diagnosis. The data is then prepared by transforming this raw data to usable data to develop a sufficient diagnosis; this is known as data cleaning. After this a user will choose the right and most effective model to filter and answer the question using the preprocessed data. When the user has made their decision, the model is then trained using ML to ensure and test model accuracy. The model is then heavily criticised and modified before release. Right before release the model is then given parameters on learning rate, distribution, initial values, performance, etc. to guarantee it meets regulatory standards. Finally, upon release, the model can and will efficiently make predictions based on all the data and parameters given in the previous steps.
CNNs, or Convolutional Neural Networks, are a type of neural network that is specifically wired for image processing. A recent study defines CNNs as “a type of artificial neural network that is particularly effective at analysing image data”, which can be used to detect tumours and mysterious objects from scans submitted to the model (Menon et al., 2024). It is clear that both ML and CNNs are some of the most significant parts of the overall machine that must work cohesively to give an accurate diagnosis. This raises questions about who is running this model, and how would this affect workflow. Well, to answer the workflow issue, the current system is only allowed to run under strict regulatory compliance standards, which hold the technology and the developing firm accountable in the case of any breaches in security or other violations. A recent study notes how an MP’s (Medical Physicist) job has become increasingly more difficult as they are tasked with collaborating with various specialists (IT experts, data scientists, regulatory and ethics experts, etc.) to make sure the system runs according to plan (Menon et al., 2024). While the current system of coding and software is a feat within itself, it is still not perfect and carries a set of commonly discussed drawbacks along with it.
2.3 Limitations of the Current Coding System
Considering limitations is a necessity when it comes to formulating a plan to expand or enhance the current AI-integrated medical systems in place. By displaying the limitations the current model faces, we hope to progress efforts to improve the technology as a whole. Incomplete or unbalanced data sets in software can lead to larger, more complex issues to solve later on down the road, such as a biased AI. A recent study explains that due to political intervention, ethical AI has become a reality for most mainstream models. However, they noted that this is not an excuse to stop being vigilant and suspect the harmful potential it has in the wrong hands (Albahri et al., 2023). By far the most interesting dilemmas this new technology faces are in the realm of model interpretability and the black box conundrum. Referencing another recent study, deep learning models or models that can be hard to decipher, like the black box model, can potentially erode the physician-patient trust due to the lack of information physicians yield when interpreting these models, thus violating a patient’s right to autonomy and informed consent (Sandeep, 2021). Problems such as these end up becoming legal and regulatory issues due to the number of patient violations a physician is technically making in the scenario in which said models are used.
This is why software developers have opted to create models with a focus on data stewardship, which is when a computing system over-reports incidental findings (Shearer et al., 2022). This simplifies the algorithm to dodge any regulatory violations. It is important to note, however, that, although models can adapt to challenges in AI’s regulation, the process of passing laws to combat any possible transgressions made by the AI is typically slow and has to be vetted through a tedious process. Finally, even if all the challenges are hurdled and a perfect model is produced, it still must integrate with the current medical systems to not disturb the strenuous administrations of hospitals. A 2023 study by Pouyan Esmaeilzadeh found that broad-scale integration would face underlying issues and concerns, such as securing patient trust, keeping significant regulatory standards, and integration into intricate health systems (Esmaeilzadeh, 2023). While they note how, with concentration and determination, the idea of AI in the workplace can be successful, without the cooperation and trust of physicians and administrators, there will be no one to advance the technology within their respective networks.
2.4 Future Directions
Looking ahead, once the technology leaps over the hurdles it currently faces and is given time to adjust and become a part of the health system, AI will have the ability to provide personalised care for the patients that it screens and diagnoses. Continuing with the direction of cancer care and prevention, AI could potentially provide insights on dietary restrictions and plans, as well as lifestyle changes that could decrease overall risk. The AI would enact this by making use of statistical methods, as well as utilising strategies for analysis and integration. However, do not mistake this future opportunity for an easy feat. On the contrary, it would require massive amounts of data storage, aggregation, an accessible system model, and ultimately the ability to integrate with the current or updating system of the time. Although promising, the cost and time required to develop may mean that this feature won’t be seen for a while. Kasula writes on how health systems can exploit AI to research and discover a patient’s biomarkers, which can add to the personalised aspect of care and treatment, all of which AI brings to the table. From predicting the outcomes of treatment to maximising an efficient healthcare delivery, AI can promise quality care reliably and quickly, meaning the capacity to help others in need is greatly expanded. However, like the feature prior, this implementation would likely be costly and the consumer on the other side of the bill would be the one mostly feeling the brunt of this cost.
Not all of the problems mentioned need to be tackled by one health system or country. The development of AI potentially seeks to bolster international collaboration, as this technology has the potential to help all around the globe. A 2020 study revealed that countries such as China and India have already begun to emerge in the sphere of AI development along with the US and Europe, so it would be for everybody’s benefit if research was pooled and technology was shared between nations (Pianykh et al., 2020) This feature also can help direct the international legality of AI and set the boundaries/guidelines for the technology to abide by as it develops in other spaces.
Finally, a model that always learns from previous cases and new data will prove to be the strongest characteristic that an AI model can boast because it should hypothetically be low maintenance aside from some software checks and organisation. The authors of the “Radiology Vol. 297″ textbook, Pianykh et al. (2020), and others believe that during its development, it is the radiologists’ responsibility to constantly update and question the AI model with data as the problem currently is not AI taking over humans’ roles in professions, but in allowing low-quality AI to serve in critical systems, such as hospitals.
3. Legality While Implementing AI in Breast Cancer Screening
The legal and ethical effects of involving AI in breast cancer screening are still very much unresolved. Many radiologists and medical students are anticipating a transformation in the radiology industry with AI, though a lot of research and modifications are still needed (Högberg et al., 2023). It is imperative to implement well-founded policies to avoid catastrophic outcomes and facilitate successful integration into healthcare.
3.1 Responsibility
A major consideration of employing AI in breast cancer screening is responsibility: what would happen if AI were to make mistakes or cause harm? There are already some lawsuits present due to mistakes caused by AI and it is unclear who is to blame. AI allows institutions to conduct a significantly higher rate of mammography imaging (Mezrich, 2022). Contrary to radiologists, AI is tireless and operates at an astonishingly fast pace. Despite the speed and accuracy of AI, it can have flaws. The AI systems are not perfected; mistakes are unavoidable. Since AI analyses a considerable number of cases per day, even a small percentage of errors can make a significant difference in the long run (Mezrich, 2022). AI techniques are continuously improved and perfected, and they are also becoming much more accepted and popular in society. Several liability issues remain unsettled, and yet they need time to be perfected, though the radiologists currently seem to carry the final responsibility (Hsieh, 2024). Establishing a national programme to compensate victims of AI’s inaccuracy could solve the current responsibility and legal conundrum. Such programmes already exist to protect patients who have suffered adverse effects from vaccines. A proposal could be developed to protect patients who suffer harm due to AI malfunctions (Hsieh, 2024).
3.2 Perceptions About AI
A survey in Sweden explored the point of view of radiologists on the implications of AI in mammography screening (Högberg et al. 2023). They investigated radiologists’ insights on the advantages and uncertainties of using AI in their field. The results showed that approximately 80% of breast radiologists were in favour of using AI in their practice. Roughly 20% of the participants were mainly unsure or unfavourable of making use of AI in breast cancer screening. Some specified that their perceptions are based on the fact that AI detects a substantial number of false positives due to its accuracy in detecting calcifications (which are not always cancerous). This study revealed diverse opinions on integrating AI in breast cancer screening. A considerable number of respondents also worried that AI could decrease radiologists’ skills and knowledge in evaluating mammograms (Högberg et al., 2023).
3.3 Transformation of Standard of Care
Over the years, AI has significantly evolved. Until now, the use of AI in breast cancer screening has been optional (Hsieh,2024). These systems are still researched and monitored closely, and studies have stated that AI could enhance breast cancer screening (Conner, 2024). AI algorithms also can identify early minimal signs of breast cancer, which may be unnoticed by the human eye (Breast Cancer Research Foundation, 2024). With further developments in AI systems, use of AI could become the new standard of care, and radiologists who decide not to make use of AI may be subject to accusations of performing below the standard of care (Hsieh, 2024).
3.4 Confidentiality
The use of AI in breast cancer screening can pose confidentiality risks. Data breaches are serious and can lead to a loss of trust from the people they affect. In the United States, the HIPAA (Health Insurance Portability and Accountability Act) regulation is in place to provide privacy protection in healthcare (US Department of Health and Human Services, 2022). The algorithms used in AI frequently need to obtain a lot of the patient’s data, and may use it at different moments, which presents elevated privacy risks (Farhud et al., 2021). Supposing that this data is not correctly protected, a wrongful institution or individual could obtain it. This could cause privacy breaches and can lead to severe legal issues.
AI’s growth has outpaced the development of its legal framework. While the technological and legal aspects are perfected, patients could be given the choice of whether they want standard radiology care or to have AI read and assess their mammograms. This could be done by signing consent forms. Once these devices are fully developed, they will reduce the workload of radiologists by analysing many more mammograms quickly and efficiently while avoiding false positives. This will allow radiologists to focus on other tasks that AI isn’t yet capable of accomplishing.
4. Equity in AI Screening
Compared to HICs (high-income countries), LMICs (lower-middle-income countries) are at a disadvantage when it comes to access to healthcare, AI included. The lack of consideration of infrastructure and human resources necessary to implement these AI technologies is the recurrent problem that is preventing AI from being successfully integrated into underdeveloped healthcare systems. Factors such as the cost of implementation and the needs for maintenance and ongoing support, trained personnel to use AI technologies, and technological support to allow for the integration of the developed AI technologies must be considered.
To ensure equity in AI screening, AI technologies developed in HICs must be effectively applied in LMICs, such that AI technologies which are primarily designed in HICs are implemented in LMICs. However, this is prevented as the divide between the location of development and the location of the implementation of AI in global oncology is a prominent issue. To solve this, greater attention to co-design, which refers to the involvement of end users in the design of AI technologies, is required. To ensure a more equitable distribution of benefits within LMICs, research should extend beyond specific partner institutions, engaging additional stakeholders from relevant government and nongovernmental organisations to evaluate and implement technologies.
Access to medical imaging, such as mammography, ultrasound, and Magnetic Resonance Imaging (MRI), is another area where inequalities exist. Some patients face long wait times for imaging services or are unable to access the services at all due to geographic or financial constraints. Such delays in diagnosis and treatment significantly impact health outcomes for breast cancer patients. Furthermore, there are also racial and ethnic disparities in access to medical imaging, with marginalised and low-income populations and ethnic minorities not receiving sufficient information for timely preventative care or the treatment they need, leading to severe health complications. Studies have shown that in particular, African-American and Hispanic patients are less likely to receive certain imaging procedures compared to their White counterparts. This may be due to implicit biases among healthcare providers or structural barriers within the healthcare system.
The strategies suggested to solve this issue are as follows:
- Ensuring cultural competency: Radiologists can partake in ongoing cultural education and training, which involves learning about the language, customs, traditions, and beliefs of the patient population they serve.
- Advocating for policy change: In the field of breast cancer, governments, and healthcare systems globally have taken proactive measures to ensure equal access to screening services, aiming to remove barriers and enhance healthcare infrastructure for underserved populations.
- Implementation of telemedicine: Telemedicine refers to the provision of remote clinical services via real-time two-way communication between the patient and the healthcare provider. By enabling remote consultations, facilitating second opinions, and extending specialised knowledge to remote areas, telemedicine can facilitate the minimising of disparities in AI for breast cancer. This dual approach of leveraging teleradiology to provide access to specialised expertise, while simultaneously empowering local professionals, can lead to more sustainable and effective healthcare delivery in underserved regions. Timely interpretations for several radiological examinations can be achieved – like mammography for breast cancer screening – even in areas where radiologists are not available on-site. This enables healthcare providers to make faster and more accurate diagnoses.
5. Understanding the Financial and Medical Benefits of AI Models in Cancer Cases
5.1 How AI Modeling in Lung Cancer Decreases Estimated Cost per Patient
AI cancer screening is a cost-effective system that must be added to every hospital that can afford it. In this case study, the scientists look into the average cost of having AI-assisted cancer imaging in lung cancer cases. This case study mainly looked at patients aged 55-80 who had smoked for more than 20 years (Ziegelmayer et al., 2022). This specific data set was chosen due to their enhanced risk of lung cancer or similar disease. AI models in lung cancer screening are a major benefit in classification and preventative care in a study by Ziegelmayer et al. (2022).
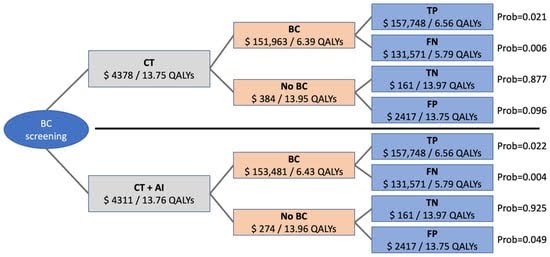
(Ziegelmayer, Graf, Makowski, 2022)
AI cancer screening models such as these have been found to decrease the need for additional treatment before diagnosis (Ziegelmayer et al., 2022). Not only does it not cost much to the patient and hospital, it can actually save millions of dollars every year. The cost for an AI cancer screening is around $1,240 USD per patient. While this is a steep cost, the potential cost for a false positive or false negative averages around $60,000. In this specific case study, the $1,240 matches the amount for a CT scan at this specific hospital. A CT scan would likely be the next step if the hospital found the need to provide additional screening based on both AI and doctor-based analysis.
5.2 AI Models in Colon Cancer in US and UK Based Hospitals
These AI cancer screenings have also been found to be extremely financially beneficial to hospitals. Their added success in preventative cancer treatment will increase hospital attendance ratings and will also likely help the hospital become recognised for its work (Areia and Mori, 2022). A study investigating AI cancer screenings in colon cancer, specifically AI pattern recognition in colonoscopy imaging, found that the AI screening led to an 8% decrease in colonoscopies and a 6% decrease in mortality rates. This hospital recorded a prevention of 7,194 colorectal cancer cases and over 2,000 related deaths. It also financially saved the hospital, patients, and related parties almost $290 million USD annually. It is expected that the effects of AI cancer screening on amount of money, deaths, and cases will increase due to rising population, access to care, and added risk to the population (Areia and Mori, 2022). It is also expected that the cost of an AI cancer screening will decrease, due to the effectiveness of AI models increasing with more data sets being studied (Areia and Mori, 2022).
6. Conclusion
AI still has a long way to go before achieving perfection. Its privacy concerns, as well as its potential for catastrophic outcomes, make it difficult for widespread adoption. However, this technological revolution has improved people’s lives. Its comprehensive detection and precise diagnoses of cancer mean that radiologists and physicians can prevent more cases of cancer and ensure transparent updates. Efficiency, effectiveness, and quality are just a few of the many adjectives used to describe the revolutionary effects of AI on the medical field. As future medical professionals, it is our job to guide AI so that technology can aid humanity and work in cohesion rather than competition.
Bibliography
Areia, M., Mori, Y., Correale, L., Repici, A, & Bretthauer, M. (2022) Cost-effectiveness of artificial intelligence for screening colonoscopy: a modeling study. Lancet Digital Health. https://pubmed.ncbi.nlm.nih.gov/35430151/
Breast Cancer Research Foundation. (2024). Can AI and Machine Learning revolutionise the Mammogram? Breast Cancer Research Foundation. https://www.bcrf.org/blog/ai-breast-cancer-detection-screening/#:~:text=AI%2Dpowered%20software%20can%20automate,undetectable%20in%20its%20early%20stages
Conner, K. (2024). Using AI to Detect Breast Cancer: What We Know. Breast Cancer Org. https://www.breastcancer.org/screening-testing/artificial-intelligence
Daniel, D. (2019). Precision Medicine in Cancer Therapy. Springer Link.
Farhud, D., & Zokaie, S. (2021). Ethical Issues of Artificial Intelligence in Medicine and Healthcare. National Library of Medicine. https://www.ncbi.nlm.nih.gov/pmc/articles/PMC8826344/
Högberg, C., Larsson, S., & Lång, K. (2023). Anticipating Artificial Intelligence in Mammography Screening: Views of Swedish Breast Radiologists. National Library of Medicine. https://www.ncbi.nlm.nih.gov/pmc/articles/PMC10230899/
Hsieh, P. (2024). Who Pays The Bill When Medical Artificial Intelligence Harms Patients? Forbes. https://www.forbes.com/sites/paulhsieh/2024/03/28/who-pays-the-bill-when-medical-artificial-intelligence-harms-patients/
Istasy, P., Lee, W. S., Iansavichene, A., Upshur, R., Gyawali, B., Burkell, J., Sadikovic, B., Lazo-Langner, A., & Chin-Yee, B. (2022). The Impact of Artificial Intelligence on Health Equity in Oncology: Scoping Review. National Library of Medicine. https://doi.org/10.2196/39748
Lamb, L., Lehman, C., Gastounioti, A., Conant, E., & Bahl, M. (2022). Artificial Intelligence (AI) for Screening Mammography, From the AJR Special Series on AI Applications. American Journal of Roentgenology, 219 (3). https://ajronline.org/doi/full/10.2214/AJR.21.27071
Mayo Clinic (2024). Breast Cancer. https://www.mayoclinic.org/diseases-conditions/breast-cancer/symptoms-causes/syc-20352470
Menon, G., Alkabban, F. M., & Ferguson, T. (2024) Breast Cancer. National Library of Medicine https://www.ncbi.nlm.nih.gov/books/NBK482286/
Mezrich, J. (2022). Is Artificial Intelligence (AI) a Pipe Dream? Why Legal Issues Present Significant Hurdles to AI Autonomy. American Journal of Roentgenology, 219 (1). https://www.ajronline.org/doi/10.2214/AJR.21.27224
Murdoch, B. (2021). Privacy and Artificial Intelligence: Challenges for Protecting Health Information in a New Era. BMC Medical Ethics. https://bmcmedethics.biomedcentral.com/articles/10.1186/s12910-021-00687-3#
News Medical Life Sciences. (2023). What is Telemedicine? https://www.news-medical.net/health/What-is-Telemedicine.aspx
Pesapane, F., Tantrige, P., Rotili, A., Nicosia, L., Penco, S., Bozzini, A. C., Raimondi, S., Corso, G., Grasso, R., Pravettoni, G., Gandini, S., & Cassano, E. (2023). Disparities in Breast Cancer Diagnostics: How Radiologists Can Level the Inequalities. National Library of Medicine. https://doi.org/10.3390/cancers16010130
Shah, C., Nachand, D., Wald, C., & Chen, P. H., (2023). Keeping Patient Data Secure in the Age of Radiology Artificial Intelligence: Cybersecurity Considerations and Future Directions. Journal of the American College of Radiology. 20 (9), 828-835. https://www.sciencedirect.com/science/article/abs/pii/S1546144023005239#:~:text=AI%20implementation%20configurations%20and%20development,workflow%2C%20including%20beyond%20image%20interpretation
US Department of Health and Human Services (2022). Summary of the HIPAA Privacy Rule. US Department of Health and Human Services. https://www.hhs.gov/hipaa/for-professionals/privacy/laws-regulations/index.html
World Health Organisation. (2024). Breast Cancer. https://www.who.int/news-room/fact-sheets/detail/breast-cancer#:~:text=Breast%20cancer%20is%20a%20disease,producing%20lobules%20of%20the%20breast
Ziegelmayer, S., Graf, M. Makowski, M. & Gawlitza, J. (2022) Cost Effectiveness of Artificial Intelligence Support in Computed Tomography Based Lung Cancer Screening. Cancers 14(7). pp17-29. https://www.mdpi.com/2072-6694/14/7/1729