Introduction
The implementation of artificial intelligence (AI) into the finance sector has led to a profound transformation, reshaping how financial institutions operate and conduct risk management, applications of AI in personal banking services, along with various other investment opportunities, as well as its notable impact on labor market automation.
The integration of AI into various industries has ushered a new era of technical advancements in the financial sector of personal banking. AI technologies have had a significant impact on personal banking due to their ability to stimulate human intellect. By enhancing convenience and ease, effectively identifying and resolving financial fraud, and promoting hyper-personalization of financial decision making, AI algorithms have modernized the client experience significantly. However, the effects of AI on personal banking are multifaceted, with AI’s inadvertent bias posing challenges due to ethical and regulatory considerations along with safety concerns when handling sensitive customer data.
Labor market automation concerns are growing as digitization and AI become more pervasive, which government leaders and business executives want more data on work tasks and automation processes. Narrow AI can replace humans in some routine physical and cognitive occupations, increasing productivity while creating jobs across industries. But high-level cognitive demand tasks must remain uniquely human. The AI’s, like ANN and SVM help in predicting the markets rise and fall. These AI’s use materials from the internet and compile it to form its predictions. It backtests the models and gives us the best option. It does this by analyzing data on the internet.
In the last 5-10 years, there’s been a significant increase in the use of AI in investments. AI has been able to increase the accuracy and effectiveness in which investors are able to execute trades. In such a short amount of time AI has been able to revolutionize the strategies, techniques, and platforms that investors use in order to trade.
AI’s Impact on Personal Banking (Aadya Kedia)
Enhancing customer interactions
Firstly, one of the most significant impacts of AI on personal banking is its transformative effect on customer-centric interactions that guide consumers into making informed investment decisions. Specifically, chatbots and virtual assistants can engage with customers in a natural language, providing instant responses to queries and assisting with various consumer support tasks such as balance inquiries, transaction history checks, and fund transfers. Moreover, the implementation of AI in the banking sector builds a sense of consumer confidence by ensuring that the transactions are free of biased interference by humans. The round-the-clock service by AI minimizes the need for customers to visit physical branches or wait on hold for customer service representatives, enhancing customer satisfaction (Artificial Intelligence, Machine Learning and big data in Finance 2021).
AI gives better experience beyond the customer expectation | |||
AI improves the sense of safety during transactions | Disagree Neutral Agree Strongly agree | Total | |
Disagree | 3 10 27 4 | 44 | |
Neutral | 1 18 22 6 | 47 | |
Agree | 1 5 20 2 | 28 | |
Strongly agree |
0 6 18 12 | 36 | |
Total | 5 39 87 24 | 155 |
Figure 1: Chart presenting the results from a survey comparing the effect of AI on consumer satisfaction in terms of safety and experience (Cegiela et al., 2022).
According to Table 1, 74.0% of the respondents ‘Agreed’ or ‘Strongly Agreed’ that AI enhances consumers’ banking experiences beyond their expectations. However, only 41.3% of respondents ‘Agreed’ or ‘Strongly Agreed’ that AI improves their sense of safety while making business transactions. Thus, only 7.7% of respondents ‘Strongly Agreed’ that AI improved their overall banking experience, with a majority of consumers still wary of trusting banking sites. In order for AI to have a significantly positive impact on personal banking experiences, sites must enforce safety considerations, for instance by reinforcing privacy policies (Cegiela et al., 2022).
Ensuring customer satisfaction
Moreover, AI’s impact on personal banking extends beyond customer interactions to back-end operations. Tasks such as document verification, fraud detection, and credit assessments are all time-consuming, labor-intensive tasks involved in personal banking. The automation of many of these tasks by AI has led to significant improvements in operational efficiency and financial safety for customers. A key example of AI working to prevent cases of money laundering is in China, where using surveillance cameras in banking is a norm. The devices, equipped with latest face recognition and data analysis technology, are used to monitor the behavior of customers and employees when carrying out any financial transactions (Artificial Intelligence, Machine Learning and big data in Finance 2021).
Furthermore, another example of AI recognizing fraudulent bank transactions is through the use of machine learning. The function of the machine learning algorithm involves identifying a particular transaction as either real or fake, based on a database of historical data of previous transactions.
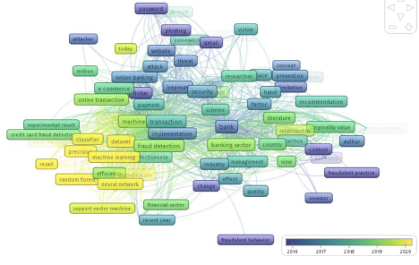
Figure 2: Map based on statistics of average number of publications in abstract and citation database, Scopus. (Artificial Intelligence, Machine Learning and big data in Finance 2021)
The map above presents an increased frequency of terms such as fraud detection and machine learning, suggesting an increase in concern of fraud by financial institutions and regulatory bodies worldwide. Likewise, according to the American Bankers Association, in 2018, the finance industry incurred about $2.8 billion in fraud losses, a 27% increase from 2016, indicating that financial losses due to money laundering is on the rise. The development of AI as technology progresses can hopefully enable features, such as using AI to analyze large amounts of data to identify specious activities and to track user activities in physical bank stores, to be implemented in the personal banking sector. By tackling fraud in a rapid and accurate manner, banks can improve their trust and compliance with their customers and partners (Cegiela et al., 2022).
Alongside working to ensure financial safety for customers, AI also allows for enhanced financial decision-making. AI’s impact on personal banking goes by customer service and operational enhancements, extending to the very core of financial decision-making. The integration of AI algorithms into investment advisory services have made sophisticated financial planning tools much more accessible. Robo-advisors, powered by AI, offer automated investment recommendations based on customers’ profiles, financial goals, and market trends. This empowers individuals with a lack of access to professional financial advisory in the past to make informed investment decisions (Cegiela et al., 2022).
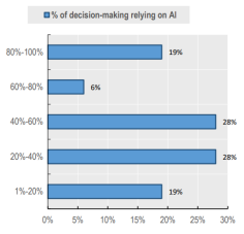
Figure 3: Graph presenting the extent of AI’s role of decision-making. Data based on industrial research by Barclay’s as of 2018 (Artificial Intelligence, Machine Learning and big data in Finance 2021).
A key conclusion we can draw from data from this graph, is that 56% of financial institutions, which is the greater majority, stated that about 20% to 60% of their decision making relied on AI algorithms to support key financial decisions for customers. However, there is also a large spread of data, with 19% of financial institutions relying on 1% to 20% of AI for decision making, while an equal 19% stated that they relied on AI algorithms 80%-100% of the time. This may suggest that due to the various benefits and challenges of using AI in personal banking, different firms are at different stages when incorporating AI in decision making (Artificial Intelligence, Machine Learning and big data in Finance, 2021).
Personalized banking services
Moreover, financial data analysis enhanced by AI has paved the way for hyper- personalized banking experiences. By analyzing customers’ transaction patterns, spending habits, and financial goals, AI algorithms can offer tailored recommendations for saving, investing, and budgeting. This level of
personalization not only strengthens customer relationships but also aids customers in achieving their financial objectives. For instance, an AI-driven method that focuses on ‘buying moments’ frequently targets particular acquisition pools, micro-geographies, and client categories according to life stage, financial wallet, and potential for both short- and long-term value (Cegiela et al., 2022). However, findings from a 2021 survey conducted by NTT Data, suggest that 83% of financial institutions claim that AI can differentiate offerings and increase customer satisfaction. Yet, only 16% of financial institutions actually implement AI and data to provide personalized services, indicating that AI’s significant impact on personalized banking is yet to hit (Decision intelligence – home|ntt data 2020).
Ethical and Regulatory Challenges
Although there are several advantages of AI in personal banking, it’s not without its challenges. Due to the potential bias caused by AI algorithms, ethical issues must be taken into account. These algorithms have the potential to perpetuate current social, economic, and demographic inequities when developed through biased algorithms. Biased data can result in unjust lending practices in personal banks, possibly denying people or communities access to financial services. Banks must strictly ensure that their AI systems are trained on a variety of representative datasets in order to solve this issue. They also must conduct rigorous testing to find and correct biases (Browne, 2023).
In a specific case, the ethical component of bias in banking has been highlighted by the European Banking Authority (EBA) as an issue that calls for a suitable regulatory framework. Major corporations such as Microsoft and Salesforce, have employed ethics experts to monitor and prevent bias against those of a certain race, gender, or other undesirable characteristic in their algorithms. Furthermore, a forecast by Gartner, the consulting company, states that by 2023, at least 75% of large firms will have recruited AI professionals to lower reputational risks by unintended bias (Decision intelligence – home|ntt data 2020).
Regulatory issues also impact the application of AI in personal banking. Regulatory frameworks often struggle to keep up with the rapid evolution of AI algorithms. Defining liability, accountability and transparency in the case of AI-related malfunctions is difficult due to the dynamic nature of AI. Regulators must balance protecting customer rights with promoting innovation. To ensure that AI technology used in personal banking uphold ethical norms and comply with current financial regulations, robust regulatory frameworks are required (Huang & You, 2022).
Security and Privacy Concerns
Although AI has undoubtedly enhanced operational effectiveness and consumer experiences in personal banking, it has also resulted in new security and privacy concerns. Customers may be more at risk for data breaches and cyberattacks due to the increased dependence on online platforms and data exchange. Users seeking to exploit the flaws in AI algorithms, may target investment websites dealing with sensitive financial data (Browne, 2023).
Moreover, access to a vast amount of consumer data is necessary for the use of AI in fraud detection and prevention. Customers may be reluctant to share private financial information, due to the concern of its misuse or access by unauthorized parties, thus gathering and analyzing such data creates privacy concerns. Banks must implement strong cybersecurity measures to protect client data to ensure that the financial information of customers is secure (Huang & You, 2022).
AI and Labor Market Automation (Efe Gürelli)
AI can enhance the efficiency of your marketing initiatives by automating repetitive tasks such as contacting prospects or analyzing data. AI also can assist in creating deeper customer
profiles so you can target messages directly to them. Though initial concerns of automation will likely lead to jobless apocalypse may persist, most scholars agree that automation will reshape work rather than simply replace it.
How will AI impact the job market?
According to a recent report by the World Economic Forum, AI may replace 85 million jobs by 2025 while also creating 97 million new ones – depending on its adoption rate and speed of integration into company operations. The exact impact will depend on how widespread adoption is of AI technology into operations. Jobs that involve repetitive tasks that can be automated are at greatest risk from AI; those requiring creativity, empathy and social interaction may be less susceptible. That doesn’t mean AI won’t impact them at all – rather it may simply reshape them into different types of positions
People who are employed as barbers, plumbers, dishwashers and nannies may lose some of their traditional functions but according to a report may find new employment in areas like data analysis or software engineering. Accountants and bankers will see themselves transformed into roles such as customer service representatives or consultants. Though AI may threaten job losses in certain industries, workers in sectors more exposed to AI tend to believe it will enhance rather than diminish their careers. Professional services providers, finance, insurance, real estate and government workers tend to hold the highest confidence that AI will enhance their work experience and many believe their skills will remain valued long after AI becomes mainstream.
How will AI impact the economy?
Some fear AI could cause widespread job losses and economic recession, while others believe it will increase productivity, create new jobs and transform existing work rather than simply replacing workers. The impact of any new technology depends on both micro and macro factors; productivity
effect for AI depends on its output per worker increase rate, cost per task generated and its ability to reduce displacement effects.Goldman Sachs estimates that generative AI could boost global output by 1.5 percentage points annually within ten years after widespread adoption, comparable to its incremental contribution to growth through other general-purpose technologies throughout history. However, the report warns of its uneven effects: two-thirds of occupations can automate some part of their workloads; even in industries affected by AI technology there remains uncertainty as to which jobs may be lost and which will remain. Workers able to complement AI with complementary tasks will see higher compensation while those whose duties are replaced will suffer financially as AI replaces their responsibilities with AI services.
How will AI impact the workforce?
AI automation and its potential effects on jobs is of great concern, according to estimates by some researchers. Some estimates indicate that AI could disrupt employment prospects for large numbers of workers by replacing them with machines; other experts are more optimistic regarding human employment prospects.
The MIT Center for Science, Technology, and Society (CSTI) has concluded that AI is more likely to transform work rather than displace jobs. Their analysis of its potential effects cited a variety of studies showing job loss due to automation would likely be offset by other positive forces – including job creation for data scientists and engineers who specialize in AI development; as well as new hybrid jobs that combine human with machine skills like managers who supervise automated systems while training them to perform new tasks.
The Brookings Institution predicts that “new automation,” with more advanced robotics and AI, will broaden the range of tasks automatable within several decades to include those held by college graduates, professionals, vehicle drivers, retail workers and retail workers themselves – becoming much more disruptive to workers than previous forms of automation.
How will AI impact the workplace?
As much as many fear AI will displace workers quickly, experts point out that its actual impact will likely be far less drastic. While certain jobs may disappear completely due to AI adoption, others will arise and flourish alongside it. As AI advances across sectors and countries alike, businesses should understand its impact on operations as well as workforces before adopting such technology themselves.
One way artificial intelligence is having an effect on work is through its impact on productivity. AI helps companies reduce operational costs, which they then pass along as lower prices to consumers. Furthermore, this productivity increase creates new job opportunities in fields like engineering, programming and robotics which means higher wages overall for workers in these fields.
Other fields of work are already moving towards partial automation as machines complement human labor. For instance, doctors who once read medical scans could become AI powered assistants that help identify symptoms and prescribe treatments; warehouse workers who once lifted and stacked items may shift into monitoring and troubleshooting automated systems instead.
At times, human skills will always be required. These include critical thinking, creative problem solving and communication therefore workers in these fields should make investments to develop these abilities while remaining informed on how AI is impacting the workplace so that they can adapt accordingly.
AI & Investments (Uche Nwokocha)
Since the recent advancements of Artificial Intelligence, there have been changes implemented into the techniques used for stock investing. With the help of AI, investors are now able to make more calculated decisions and manage their risk more effectively without solely relying on instinct and human analysis to make transitions.
The use of AI algorithms in stock analysis has already been revolutionizing the approaches of stock trading in many investment firms.
AI has been able to enhance the way investors analyze data. AI analysis is able to process higher amounts of financial data more effectively than humans. Without AI, stock investors can often fail to accurately interpret the high amounts of information and market data that is available to them, and because of this investors run the risk of missing potential opportunities due to incorrect judgments. With AI, investors can analyze companies, manage risk, and examine market trends more accurately than ever before.
Another impact that AI has had on stock investing is in increasing the amount of access investors have to advance investment strategies. Before, more progressive investment strategies were only accessible to institutional investors and hedge fund traders.
Besides this, AI integrated trading platforms have been able to increase the access of advanced trading tools to retail investors. These platforms have been able to use these intricate AI algorithms to customize and personalize investment portfolios for risk management and market conditions. Advanced AI strategies have been able to increase the amount of opportunities for investors to benefit from in the stock market.
In conclusion, in leveraging both AI and human effort investors can enhance their risk tolerance,market tolerance, and investment strategies to optimize their trading outcomes.
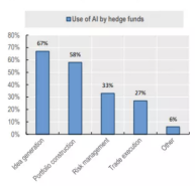
Figure 4: The Usage of Artificial Intelligence by Hedge Funds
This graph represents the percentage of hedge funds that use AI to assist them in market analysis and investing. 67% of hedge funds have used AI to aid in idea generation, Portfolio construction follows with 58%, Risk management and Market analysis with 33%, 27% in Trade execution, and lastly 6% for other usages. With this graph we can visually analyze the impact that AI has had on investments and stock trading in a larger perspective.
AI and Risk Management (Aanjaney Singh J.)
AI also has applications in risk management, with regard to both market risk and credit risk Market risk refers to the likelihood of loss resulting from aggregate market fluctuation, and credit risk is the risk of a counterparty not fulfilling its contractual obligations, which results in a loss in value. Although AI has broader uses in risk management, these two categories are the most important in asset management.
So how does AI help in risk management in different topics?
Let’s see:
- Market Risk: The AI must predict the risk factors that affect the investment portfolio. AI can play a role in this area in three ways:
(a) making use of qualitative data for risk modeling,
(b) validating and backtesting risk models,
(c) producing more accurate forecasts of aggregate financial or economic variables.
AI uses textual and image data sources, textual data sources, including news articles, online posts, financial contracts, central bank minutes and statements, and social media, which can contain valuable information for managing market risk. The AI can use satellites to check Retail stores, which have more cars, which equals to the customer and check the growth of the companies. The textual information has helped AI track the loss and profit of companies, leading to forecasts regarding which ones will prosper and which ones will crash, interest rates, and other macroeconomic outcomes. These methods can also be implemented by using the information from corporate disclosures with the aim of determining firms’ systematic risk profiles. But to date, trustable implementations and evidence in this area are scarce.
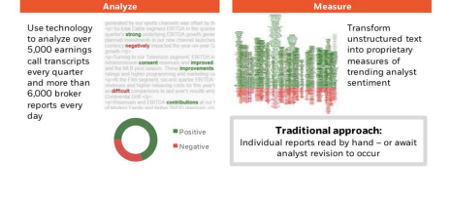
Figure 5
Backtesting
AI can also help risk managers validate and backtest risk models. Regulators and financial supervisory institutions emphasize this important part of model risk management. Unsupervised AI approaches can detect anomalies in risk model output by evaluating all projections generated by the model and automatically identifying any irregularities. Depending on the exposure of the assets in a portfolio to the underlying risk factors, various financial or economic variables can affect its performance. Therefore, modeling future trends in these factors, especially macroeconomic variables, is important.
ANN’s (Artificial Neural Network’s)
It involves computations and mathematics, which simulate the human–brain processes. Many of the recently achieved advancements are related to the artificial intelligence research area such as image and voice recognition, robotics, and using ANNs.
How do ANN’s help?
They can be used to devise systematic risk factors. These models can capture nonlinearities and interactions of covariates, including firm characteristics and macroeconomic variables. ANN’s can be used in non-diversifiable and diversifiable risks. ANN’s are able to capture the non textual/photo information and interpret the messages, which gives them an advantage over the traditional AI’s. ANN’s and SVM’s have been able to predict crises, bank crises and recessions earlier. But crises are rare financial events, so in the absence of a sufficient number of such events in the sample, one could question the ability of AI models to accurately predict future crises.
What are SVM’s?
A support vector machine (SVM) is a supervised machine learning model that uses classification algorithms for two-group classification problems. After giving an SVM model sets of labeled training data for each category, they’re able to categorize new text.
- Credit Risk: Credit risk modeling is one of the first areas of finance to consider the application of AI techniques. To ensure that the failure of any counterparty to meet its obligations does not have a negative effect on the portfolio beyond specific limits. The asset managers monitor the credit risk of the entire portfolio and individual positions and transactions. This involves modeling the risk associated with institutions issuing financial products, including equities, bonds, swaps, and options. An extensive range of approaches exists for modeling solvency or bankruptcy risk. Multivariate discriminant analysis, logit, and probit models are among the most common traditional methods used. In this field ANN’s and SVM’s again can play a crucial role, and help. The popularity of ANNs stems largely from their higher success in forecasting bankruptcy and determining credit ratings compared with traditional techniques. More-recent studies, however, advocate the use of SVMs because they yield slightly more accurate bankruptcy forecasts than ANNs do. But every AI has its pros and cons, an ensemble technique that uses various approaches separately and then combines the resulting predictions should be considered for achieving the best performance.
What could go wrong?
Although AI has many times correctly predicted crashes in the market and which companies could overperform the market, it has its own shortcomings. Understanding and explaining the inferences made by most AI models is difficult, if not impossible. As the complexity of the task or the algorithm grows, opacity can render human supervision ineffective, thereby becoming an even more significant problem. This issue might have repercussions for asset managers in three ways. First, the difficulty in predicting how AI models will respond to major surprises that could lead to systematic crashes. Algorithmic crashes are not specific to AI systems and may arise from even simple widespread quantitative approaches, such as value investing. What makes AI different, however, is that its opacity may prevent such risks from being properly modeled and monitored. Second, AI can make wrong decisions based on incorrect inferences that have captured spurious or irrelevant patterns in the data. For example, ANNs that are trained to pick stocks with high expected returns might select illiquid, distressed stocks. Third, attributing investment performance can become more challenging when using AI models. For example, the widely used Barra Risk Factor Analysis, based on linear factor models, might not suit AI-based strategies that capture indirect connections between characteristics and returns.Data quality and sufficiency can be other major sources of concern. Like other empirical models, AI models rely on the integrity and availability of data.
Conclusion
Overall, it is evident that AI is having a major effect on the world of investmenting. AI analysis has proven itself more accurate and efficient than manual human analysis. Investors have been able to take advantage of the strengths of Artificial Intelligence and apply it to areas like portfolio creation, market analysis, risk management, and investing strategies. AI has increasingly helped investors with maximizing their outcomes and rewards on their trades, with it already having such a big and impactful effect on investing, the possibilities for AI in the future are limitless.
References
Acemoglu, D., & Restrepo, P. (n.d.). Automation and new tasks: How technology displaces and Reinstates Labor. Journal of Economic Perspectives. https://www.aeaweb.org/articles?id=10.1257%2Fjep.33.2.3
Artificial Intelligence and machine learning in Asset Management. (n.d.). https://www.blackrock.com/corporate/literature/whitepaper/viewpoint-artificial-intelligence machine-learning-asset-management-october-2019.pdf
Artificial Intelligence, Machine Learning and big data in Finance. OECD.org. (2021, August 11). https://www.oecd.org/finance/artificial-intelligence-machine-learning-big-data-in-finance.htm
Cegiela, R., Panowicz, M., Strauss, M., Strzelczyk, M., & Widwitz, M. (2022, October). Just for you: The route to hyper-personalization in banking – BCG. BCG.com. https://media-publications.bcg.com/Just-For-You-The-Route-to-Hyper-personalization-in-Ban king.pdf
Decision intelligence – home|ntt data. NTT Data. (2020). https://www.nttdata.com/global/en/-/media/nttdataglobal/1_files/services/data-and-intellig ence/decision_intelligence.pdf?la=en&hash=C1F205ECC2466211A5212CE78F18F65F23220 31
Huang, A., & You, H. (2022, October 4). Artificial Intelligence in financial decision making. SSRN. https://deliverypdf.ssrn.com/delivery.php?ID=5540721200671141030970760900820250981 0201908507904901608502500112712300802709711701812212205301210405605509502 5069096125024090009086053083017026085071091122087119062017052093123072070 100002002094014018114125029000003025089118026095100123111079004078&EXT=pd f&INDEX=TRUE
Ligon, M. (2023, September 12). Council post: How artificial intelligence is revolutionizing stock investing. Forbes. https://www.forbes.com/sites/forbesbusinesscouncil/2023/07/17/how-artificial-intelligence -is-revolutionizing-stock-investing/?sh=48366b976485
Ryan Browne, M. S. (2023, June 23). A.I. has a discrimination problem. in banking, the consequences can be severe. CNBC. https://www.cnbc.com/2023/06/23/ai-has-a-discrimination-problem-in-banking-that-can-be devastating.html
Thomas, M. (2021). How AI trading technology is making stock market investors smarter. Built-In. Web.