Abstract
Autonomous Electric Vehicles (AEVs) are the future that humanity is heading towards. The future of AEVs will be heavily influenced by the user experience, making the technology more accessible to everyone. In this work, we intend to develop guidelines for human-centred design enhancements to AEVs, thereby making them more inclusive to everybody. These guidelines are based on a collection of research papers on human-centred design, human-factor engineering, and real-world self-driving electric vehicle data. Using this foundational understanding, we conducted a hierarchical task analysis (HTA) on how to operate an AEV. The HTA was created with a Tesla vehicle in mind and focuses on subtasks that differ from ordinary Internal Combustion Engine (ICE) vehicles, particularly in the areas of charging and the capabilities of the electronic computer within. To prioritise ergonomic features that improve passenger comfort and safety, we suggest using User-Centred Models which include an Accident Risk Index and Ergonomic Attributes Index using a Fuzzy Approach. They will play a central role in the design and production stages of the development of new EVs and AEVs. Furthermore, we point out areas that still require innovation in battery and power propulsion systems which should inspire businesses and researchers to keep up their research and development. Our research aims to close the gap between the human factors essential for the widespread adoption of autonomous electric vehicles (AEVs) and the technological advancements in these vehicles. In the future, we hope to create a world in which AEVs are not just cars but essential and easily accessible parts of everyone’s daily existence with a focus on efficiency, safety, environmental awareness and inclusivity through human-centred design principles.
Keywords: Autonomous Electric Vehicles, Human Factor Engineering, Human Centred Design, Hierarchical Task Analysis, User Requirements and User-Centred Model Designs
NOTE: Many driving models and characteristics are proposed and examined in our research paper and each is taken into consideration separately. We draw attention to the intricate relationships between these parts and illustrate situations in which changing one of them calls for a thorough evaluation of the vehicle’s overall functionality and performance. Comprehending this intricate concept is essential for creating reliable and strong autonomous driving systems, as the interplay among distinct elements greatly influences the overall dependability and efficiency of the system.
View ‘Human-Centred Design Improvements for Autonomous Self-Driving Vehicles‘ as pdf
1. Introduction
The advent of self-driving cars marks a significant development in transportation technology and portends safer roads, less traffic congestion and more global mobility options. As these vehicles develop and incorporate advanced artificial intelligence (AI) and real-time data sensors, attention should also be paid to making sure that this advanced technology is balanced by a design philosophy with human needs in mind. The academic paper Human-Centred Design Improvements for Autonomous Self-Driving Vehicles explores the critical intersection of technological progress and human needs, looking at how design approaches should be applied to create autonomous cars that prioritise the safety, well-being and comfort of their users and occupants.
2. Research Framework
2.1 Aims and Objectives
Aim:
Investigating and creating methods to improve the safety and user experience of Autonomous Electric Vehicles (AEVs) are the main goal of the research paper Human-Centred Design Improvements for Autonomous Self-Driving Vehicles. This entails comprehending how AEVs can be built and used in a way that prioritises user comforts, wants and needs while maintaining safety regulations and peak performance. We prioritise social inclusion and diversity of users while focusing on commercial viability.
Objectives:
- Creation of HTA: To develop a Hierarchical Task Analysis (HTA) framework focused on a human-centred design approach to improve user experience and safety in autonomous electric vehicles.
- Analysis of User Requirements: To discuss and list the common user requirements for autonomous EVs considering human safety and comfort.
- Proposing User-Centred Design Improvements: To suggest various models aimed at optimising user experience and safety of AEVs that align with the user expectations and requirements.
2.2 Methodology
A foundational understanding of the topic was created by reviewing academic research papers published in various journals on human-centred design, human engineering and real-world EV/AEV experiences and data. Using this information, we developed a hierarchical task analysis (HTA) on how to operate an AEV. The HTA is based on a Tesla, which is the closest commercially available fully autonomous electric vehicle. Based on the HTA, we highlighted significant areas where human-centred design may be enhanced. Using more study papers and publications, we conducted research into the areas that required improvement and proposed possible solutions to the problems.
2.3 Literature Review
Human-Centred Design of Vehicles
Brooks et al., 2018 stressed the significance of using a human-centred design process when creating passenger compartments for vulnerable groups like working mothers or people with visual impairments. Using interviews with these individuals the study enhanced metrics related to user satisfaction underscoring the imperative need for customised design in electric vehicles. The most significant lesson learned from these interviews is that the opinions of general customers are heavily influenced by their experiences with ICE vehicles. Meanwhile, there is no doubt that EVs have provided new opportunities to revisit classical ICE vehicle designs, offering more satisfying experiences for human transportation.
Advanced Sensory Technologies for Vehicle Operation
Sensory technology will play a central role in autonomous driving. In the years since EVs came to the market, sensory technology’s progression almost rivalled that of batteries. Besides the current development focus on autonomous driving sensors outside of the vehicle, we want to point out that the sensory technologies that support in-cabin awareness and technologies, and that which fuse in and out-of-cabin sensors for decision-making, are also important in future EV/AEV sector growth.
Rahmati and Talebpour, 2017 examined how to integrate cutting-edge driver sensing technologies to track driver activity, cognitive load and state in real-time. This approach improves Advanced Driver Assistance Systems (ADAS) and vehicle interfaces, making driving safer and more individualised. Tesla’s Autopilot system is a prime example of how this is done.
Human-Machine Interface (HMI) Design
Besides the experiences of traditional vehicle ergonomic design, the Human-Machine Interface (HMI) could be crucial for EV/AEV experiences in the acceptance of customers, especially in the phase-in stage.
Chen et al., 2020 emphasised how crucial good HMI design is to enable smooth communication between users and driverless cars. The study stressed the significance of providing support for both automated and manual driving modes and suggested creating systems that facilitate seamless transitions between varying degrees of autonomy, thereby augmenting user assurance and safety.
Naturalistic Brake Policy and Shared Control
Many studies and real-life experiences with EV/AEV suggested a divergence of in-cabin human experiences with EV/AEV. For drivers moving from ICE vehicles to EVs, the experiences are overwhelmingly positive. However, for passengers, the results are more mixed. Their main complaints focus on two distinct phases of the riding experience: the initial acceleration and the braking.
Rahmati et al., 2021 discusses incorporating a naturalistic brake policy that adapts to human driving behaviours and can significantly enhance user trust and safety in autonomous vehicles. Studies show that designing vehicle control systems that inform users of the system’s capabilities and limitations, rather than attempting to achieve perfect autonomous navigation, can lead to better user experiences and safety outcomes (Fridman, 2019).
3. Hierarchical Task Analysis (HTA)
Hierarchical task analysis is a method of breaking down a large task (say, making a journey with an EV) into smaller subtasks, allowing for a close-up analysis of what makes an EV journey different from an ICE journey and helping designers and engineers create more functional vehicles per the aforementioned user requirements.
This specific HTA was designed with a Tesla vehicle in mind and specifically highlights subtasks that differ from typical ICE vehicles, particularly in the areas of charging and the capabilities of the electronic computer inside the vehicle. More in-depth decisions relating to car customisation were not included as they do not pertain directly to the typical car journey, but they may be customised as needed.
NOTE: Under Task 2 (during the journey), different colours determine actions that depend on the preselected mode under which the car is being driven, given designations A (manual), B (semi-autonomous), and C (fully autonomous). Note that certain tasks are to be done for both the B and C cases, in which case the subtask is denoted B/C and the box has a gradient.
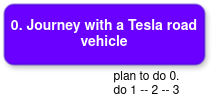
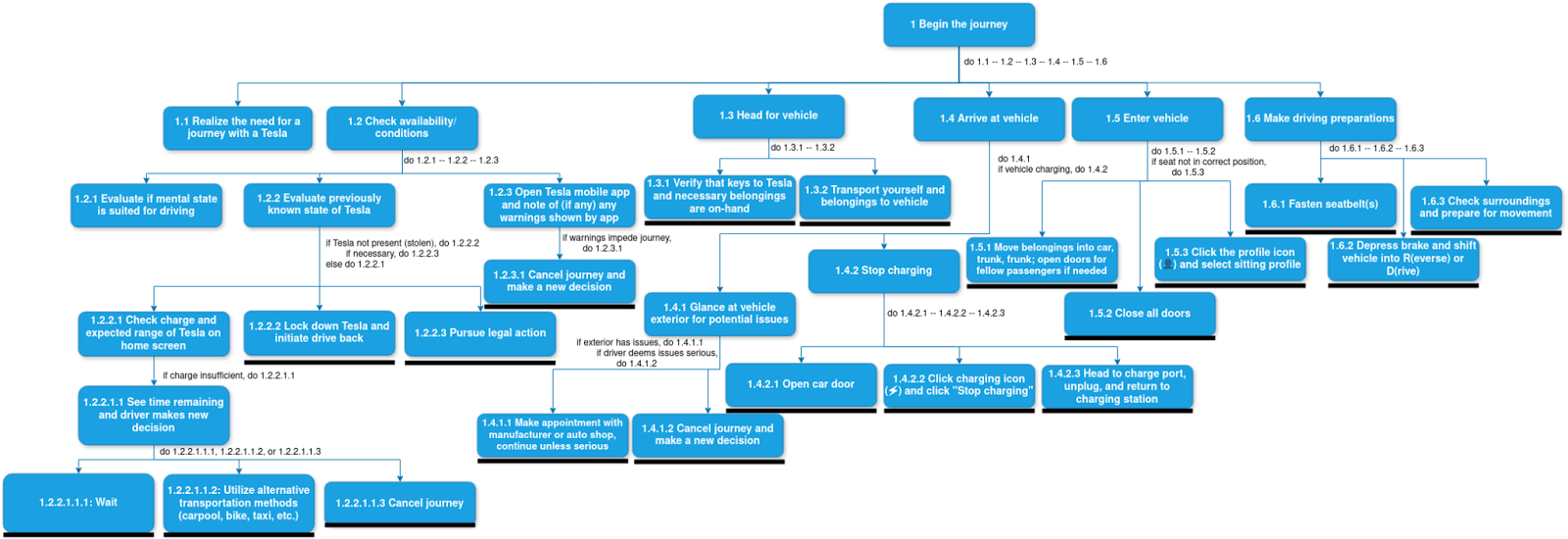
Figure 1A
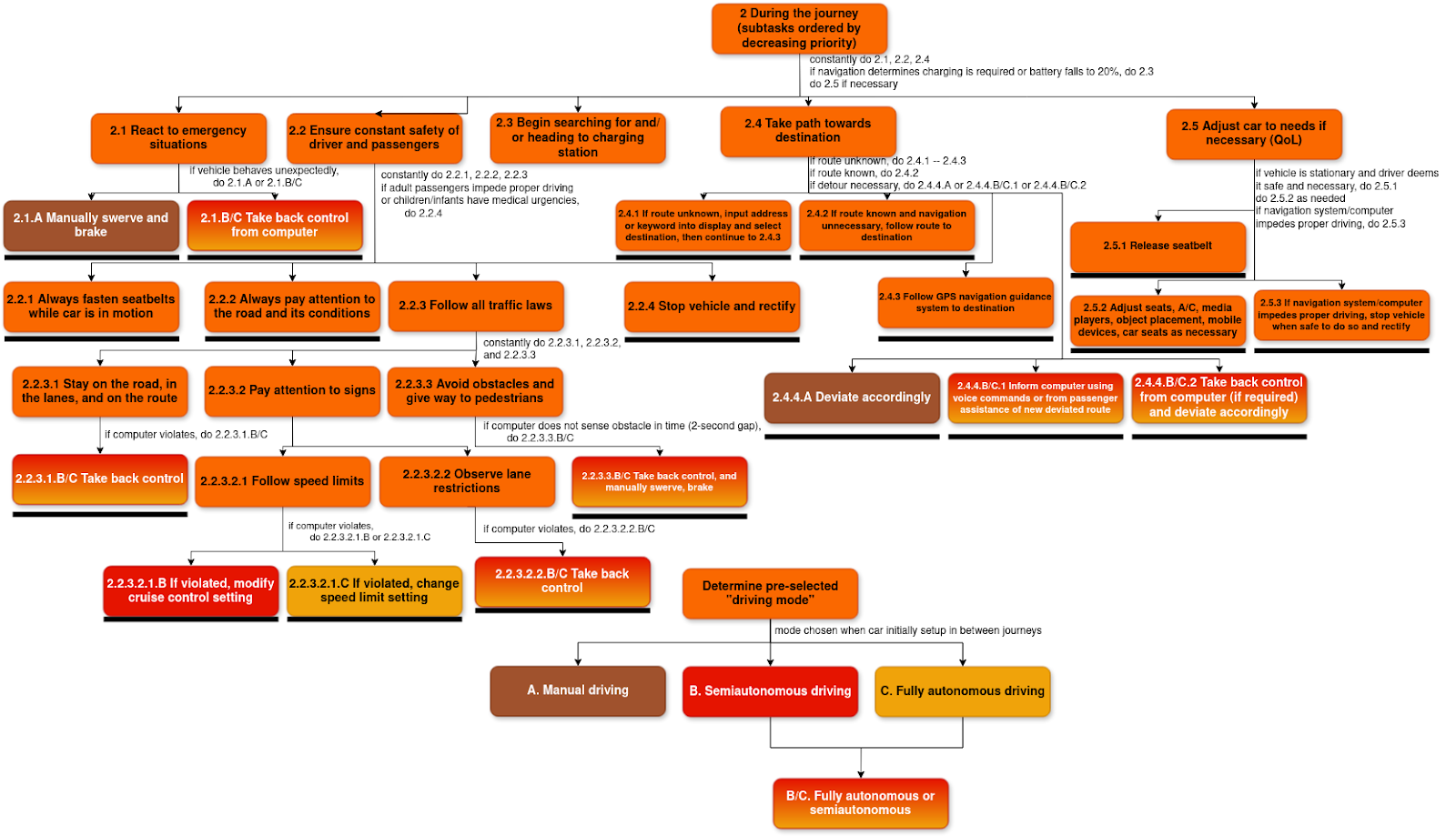
Figure 1B
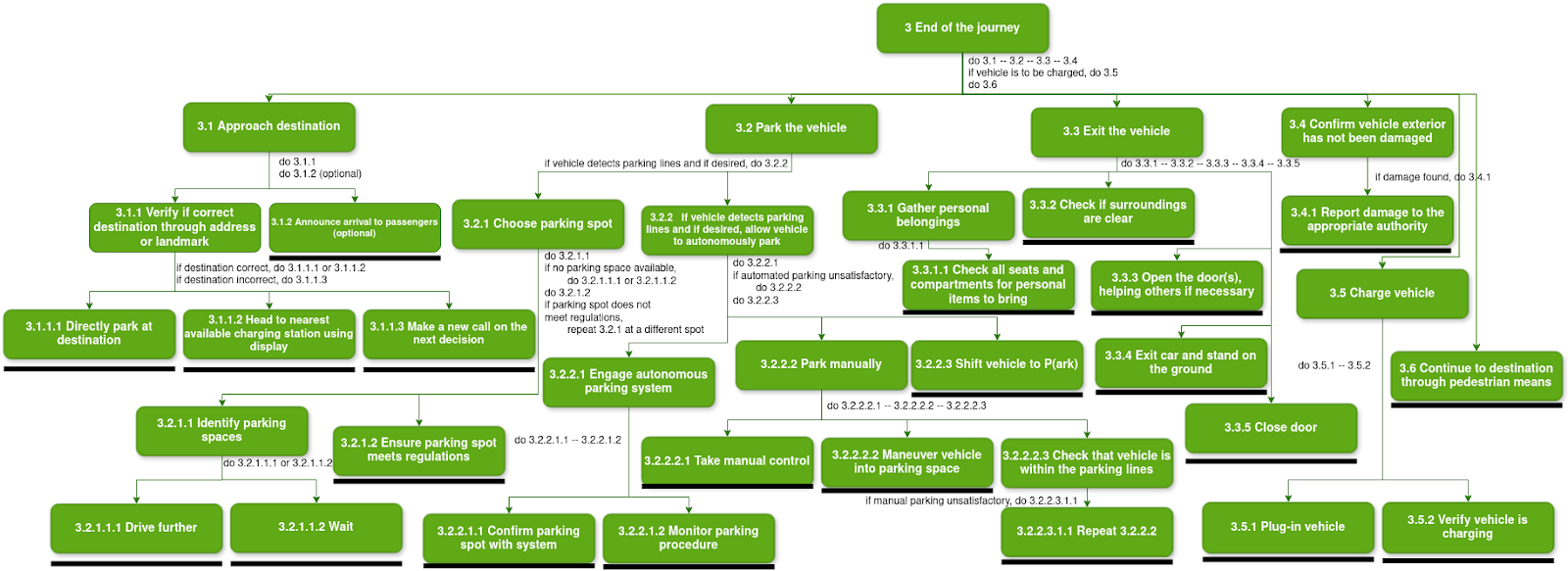
Figure 1C
4. User Requirements
Before discussing the various user requirements in an EV, we discuss the nature of autonomous driving and the technology prevalent in autonomous EVs.
Autonomous driving can be classified into the following five levels:
- Level 1: Basic driver assistance, such as adaptive cruise control, lane-keeping assistance and road sign recognition.
- Level 2: Partial autonomous driving, where the vehicle can control both steering and acceleration, but the driver must remain engaged.
- Level 3: Conditional autonomous driving, where the vehicle can handle all driving tasks in certain conditions, but the driver must be ready to take over at every moment.
- Level 4: High autonomous driving, where the vehicle can handle all driving tasks and monitor the environment in specific conditions or vulnerable areas without human intervention.
- Level 5: Full autonomous driving, where the vehicle can operate independently under all conditions without any human intervention (Park and Kee, 2021) (Dai et al., 2021).
The technology of autonomous driving can be realised in the following ways:
- Sensor suite: EVs have integrated sensors such as LIDAR, radar and full self-driving (FSD) cameras to support autonomous driving features, even allowing for differentiation between real cars (3D) and images of cars (2D). This comprehensive sensor integration enhances the vehicle’s ability to perceive and interpret its surroundings accurately, improving the effectiveness of autonomous driving features and ensuring safer navigation by distinguishing between real-world objects and images.
- Software and algorithms: EVs are using advanced software to perform real-time decision-making, object detection and path planning. Advanced software and algorithms enable the vehicle to make swift, informed decisions, detect and avoid obstacles, and plan optimal routes, enhancing overall driving safety and efficiency.
- Safety and security: EVs have robust cybersecurity measures to protect autonomous systems from hacking and/or unauthorised access. These cybersecurity measures safeguard critical vehicle systems from potential cyber threats, ensuring the integrity and safety of both the vehicle’s operation and the driver’s personal data.
- Regulatory compliance: EVs have to adhere to local and international regulations regarding autonomous driving, including testing and deployment guidelines. Compliance with regulatory standards ensures that the vehicle meets safety and performance benchmarks, facilitates smoother approval for deployment, and builds trust with consumers by adhering to established legal and safety protocols (Dai et al., 2021).
EVs (electric vehicles) are a completely new stage in the automobile market and industry, offering new alternatives to traditional internal combustion engine cars. Firstly, EVs significantly reduce greenhouse gas (CO2) emissions, contributing to a cleaner environment and reducing consumer impact on climate change — as EVs do not produce gas emissions, they do not contribute to air pollution and improve public health efforts.
Secondly, EVs provide economic advantages. Although the initial purchase price of an electric vehicle can be higher, the total cost of ownership is generally lower due to reduced fuel expenses and maintenance costs. Electric motors have fewer moving parts than combustion engines, leading to, on average, fewer repair expenses. Additionally, many governments offer incentives, such as tax credits and rebates, to encourage the adoption of EVs, making them more affordable for consumers.
Moreover, advancements in battery technology are extending the range of EVs, alleviating concerns about limited driving distances. The development of robust charging infrastructure, including fast-charging stations, further improves the facilities and infrastructure needed to support the owning of an electric vehicle. There are two new technologies on the horizon of the charging sector worth mentioning here:
- One is the battery swap system which has been adopted by some EV manufacturers, e.g. NIO, a Chinese EV company whose pilot swapping station can replace a flat battery with a newly charged one in only 15 minutes. Not only does this model shorten the customer waiting time, but it also reduces the initial investment amount since the customer only rents the battery, rather than owning it. On the other hand, the asset investment for the manufacturer will increase by a lot. We forecast this model will be promising for the early stage adoption under strong government incentives support, or support from long-term capital investment.
- The other charging technology is wireless charging, including stationary wireless charging and road-embedded wireless charging systems. For stationary wireless charging systems, universal standards or protocols would be our recommendation for a successful human-centred EV/AEV design. On the side of the road-embedded wireless charging system, it is intrinsically a human-centred EV/AEV design which could significantly improve human experiences in road travel. Several European and Asian countries have constructed pilot road sections using this technology. We believe it could be a game-changer for EV/AEV adoption if it can be widely implemented (Thomas, 2023).
As battery production rises, costs are expected to decrease in parallel, making EVs even more accessible and affordable.
Finally, EVs offer a superior driving experience. They provide instant torque, resulting in quick acceleration and good performance. The quiet operation of electric motors also leads to a more peaceful and quiet driving experience (although there are some concerns that this may impact pedestrian notice of oncoming vehicles). With continuous innovation and investment in the EV sector, the future of transportation will be increasingly electric, and such improvements will bring forth significant benefits to the environment, economy and driving experience.
To realise those targets, there are some requirements for the EV:
4.1 Performance Requirements
Overall, EV/AEV performance overwhelmingly prevails ICE vehicles. The performance expectations with human experiences in mind shall focus on how to reduce transition barriers and alleviate the worries of customers when they move from ICE cars to EV cars and from non-autonomous cars to autonomous cars.
- Range: From a customer point of view, EVs should have sufficient range to meet daily driving needs and alleviate so-called “range anxiety”. The typical target range is around 400 to 600 km per charge, which ensures that EVs can comfortably handle daily commutes and longer trips without frequent recharging, significantly reducing range anxiety and making them practical for a wide range of driving needs.
- Charging time: Fast charging capabilities are essential. Based on customer feedback, optimal EVs should be able to recharge to 80% within 30 minutes using DC fast chargers. Rapid charging reduces downtime at charging stations, allowing for quick top-ups during long journeys and making daily use more convenient by minimising the time spent waiting for a full charge.
- Acceleration and top speed: EVs should be comparable to diesel vehicles in terms of acceleration (e.g. 0-100 km/h under 8 seconds) and have sufficient top speeds for highway driving. Comparable acceleration and top speed ensure that EVs offer performance that is on par with traditional diesel vehicles, providing a dynamic driving experience and making them suitable for both city and highway driving (Ulrich, 2005).
4.2 Battery and Charging Infrastructure
- Battery lifespan: EVs need long-lasting batteries with warranties covering around 130,000-160,000 kilometres. Extended battery life and robust warranties provide peace of mind to owners by ensuring long-term reliability and reducing concerns about battery degradation, ultimately enhancing the vehicle’s value and reducing total ownership costs.
- Battery technology: EV companies need to improve battery technology with higher energy density, increased safety, faster charging and longer range. Advances in battery technology led to greater energy density and safety, shorter charging times, and extended driving range, making EVs more efficient, reliable and user-friendly, thereby enhancing overall driving experience and convenience.
- Charging network: For EVs, availability and diversity of charging stations in a country (charging stations in cities, small towns, at highway service stations) is needed, including fast chargers, to support both urban and long-distance travel. New charging station technology (e.g. NIO’s swap system) can replace a dead battery with a charged battery within only 15 minutes. A well-distributed and varied charging network ensures convenient and accessible recharging options, supports both daily urban use and long-distance journeys, and alleviates range anxiety, making EVs a practical choice for all types of travel. Recent developments have also included the development of electromagnetic induction road charging technology, allowing cars to remain on the power grid underneath roads during transportation.
- Sociability: If you live in a rural area without access to charging stations, an EV company can assist you in installing a charging station at your home, allowing for convenience in driving and charging. This helps the user maintain social circles and allows for better energy choices that would not prevent social interactions due to a lack of charging options. For example, companies such as Tesla offer home charging solutions and provide installation support. Additionally, many local electricians are certified to install EV chargers, ensuring reliable access to charging one’s vehicle. This enhances social inclusion and connection within communities.
- Battery security: EV batteries should not spontaneously combust. Utilising non-combusting batteries enhances safety by reducing the risk of battery fires or explosions, increasing the vehicle’s reliability and providing greater peace of mind for owners (DeLuchi et al., 1989).
4.3 Environmental Impact
- Sustainability: EVs need to use sustainable materials and processes in manufacturing EVs and batteries. Utilising sustainable materials and processes minimises the environmental impact of production, supports resource conservation, and aligns with eco-friendly practices, making the entire vehicle lifecycle more environmentally responsible. From a wider energy budget perspective, making each electric vehicle with more sustainable components is important to reducing the footprint of the entire vehicle’s lifespan.
- Emissions: EVs have, by principle, zero tailpipe emissions. This reduces urban pollution and greenhouse gas emissions. Zero tailpipe emissions contribute to cleaner air in urban areas, reducing harmful pollutants and greenhouse gases, combatting climate change and improving public health.
- Research on urban population limits: Prior understanding and regulation of urban pollution limits by measuring the concentration of particulate matter helps set effective regulations and benchmarks for air quality, ensuring that EVs and other measures contribute to meaningful reductions in pollution and enhance overall environmental health (Hawkins et al., 2012).
4.4 Cost Considerations
- Purchase price: EVs must have competitive pricing with traditional ICE vehicles, considering government incentives and subsidies. Competitive pricing makes EVs more accessible to a broader range of consumers, while government incentives and subsidies further reduce the initial cost, accelerating adoption and making EVs a financially viable option for more buyers.
- Total cost of ownership: Lowering long-term costs is vital — this can be in the form of savings on fuel, maintenance, and potential tax benefits for EVs. Lower long-term costs are achieved through reduced expenditures on fuel and maintenance, as well as possible tax benefits, resulting in substantial savings over the vehicle’s lifespan and making EVs a more economical choice for owners (Patil and Kalkhambkar, 2021).
4.5 Regulatory Compliance
EVs have to adhere to local and international regulations regarding autonomous driving, including testing and deployment guidelines. Compliance with regulatory standards ensures that the vehicle meets safety and performance benchmarks, facilitates smoother approval for deployment, and builds trust with consumers by adhering to established legal and safety protocols.
4.6 Suspensions, Driving Comfort, Interior Design and Connectivity Technology
- EVs use modern designs focusing on aerodynamics and energy efficiency. This design approach enhances vehicle performance by reducing drag, improving range, and contributing to overall energy efficiency (Bhatnagar et al., 2024).
- EVs should use advanced suspension systems, such as air or adaptive suspensions, to optimise weight distribution. As the main weight is located at the bottom of the car (the only place the battery should go), the centre of mass is lowered and the mechanics on the suspension are changed. For this reason, EV ball joints tend to wear down faster, as do the front axles. On a secondary note, these systems also maintain the driving comfort found in ICEs by adjusting the vehicle’s ride height and damping in real-time to accommodate varying road conditions and driving styles. Examples of this in action include the Tesla Model S and the Audi e-tron.
- The design of the suspension for ICE cars tends to remain the same because the distribution of the weight remains the same – it is now settled, for the most part, that the weight should be either in the front of the vehicle, where the engine is located, or in the back of the vehicle, where a more complicated mechanical design is needed. Most traditional car companies make cars with front-wheel drive, primarily for safety reasons (reducing the chance of a flip). This setup means the engine is effectively pulling the rest of the vehicle forward.
- The arrival of the electric vehicle allowed innovative companies to incoporate a rear-wheel drive approach (to accommodate terrain, improve balance, and increase traction). However, the addition of an incredibly heavy battery underneath the car throws the previously established setup out the window. Due to the sheer density and weight of the large traction battery under the car, an upper-lower weight distribution analysis proves to be more useful than a front-back approach. As the heaviest component of the entire vehicle, the lithium-ion battery’s only possible and reasonable location is under the floor. While this improves stability (it isn’t generally safe or sensible to be carrying top-heavy loads), this means that over longer periods of time, the suspension has to deal with a constant downward push by the heavy battery. Given the clearly novel situation, it seems reasonable to advocate for a complete overhaul of the suspension system. It is necessary to strengthen the front of the car for front-mounted electric motors, or pay close attention to the back for rear-mounted electric motors. Tesla’s suspension in particular has led to problems in the running gear and the alignment. Although many different solutions have been tested (air suspension, adaptive automatic suspension, intelligent/real-time adjustment), the fundamental difficulties remain.
- EV/AEV design shall invest more in tyre design, mostly focusing on tyre material research and development. We shall point out that both suspension design and tyre material design not only serve the purpose of riding comfort, but also the long-term customer experience and satisfaction.
4.7 Interior Environment Design and Connectivity Technology
- EV/AEV interior shall focus on environmental design, which includes but is not limited to climate control, ergonomics efficiency, interior material and light design, etc.
- On the design of climate control, the market and industry have concluded, after multiple tried and tested solutions in the past, that at the current stage, heat pump technology should be the choice of solution to provide high- efficient climate control in human-focused EV/AEV design.
- EVs are equipped with spacious interiors and ample cargo space, featuring high-quality materials like Nappa leather and organic, recyclable materials that minimise environmental impact. Infotainment systems in EVs often include premium sound systems such as Bowers & Wilkins or Bang & Olufsen, providing clear and immersive audio experiences. This ensures a luxurious and pleasant driving experience with ample room for passengers and cargo. High-quality materials enhance comfort and luxury, while premium sound systems deliver superior audio quality. The use of sustainable materials also supports environmental stewardship, and the spacious design improves overall travel comfort (Welsh and Pierce., 2018).
- EVs are equipped with advanced infotainment systems, connectivity features, autonomous driving capabilities, and regular software updates, including an app on the mobile phone of the owner for the EV to allow the driver to access the EV easily. For example, the Tesla app can lock the car, control the A/C, plan the next trip, display charging data, and perform other features. These features provide a seamless and intuitive driving experience, allowing for real-time updates, enhanced safety, and convenience through easy access to vehicle controls and information (Bhatnagar et al., 2024).
5. User-Centred Design Improvements
5.1 Car Modelling Based on Driver Safety, Human Factors Engineering and Anthropometrics
Human factors engineering refers to the integration of ergonomic principles into the design and development of devices, equipment, work systems and tasks. It aims to improve the optimal performance of the appliance, prioritise the safety of users, and reduce human errors (Anderson, 2024).
Anthropometrics refers to the “measurement of humans” and is derived from the Ancient Greek words anthro (man) and metron (measure). It focuses on the linear dimensions and functional capabilities, including weight, volume, etc. It takes into consideration the bodily features that vary based on age, population, age, gender, different geographical situations, and biological environments (Bhattacharyya and Saikia, 2024).
5.1.1 Formulation of Accident Risk Index
The Electronic Control Unit (ECU) is the electrical component within vehicles that acts as the “brain” of the vehicle by managing all internal functions. The Advanced Driver Assistance Model (ADAS) refers to the set of technologies that aim to improve vehicle safety by automating, supplementing or replacing the driver’s control over the vehicle. It utilises various devices and sensors for this purpose. ADAS is a creation that offloads the tasks of a traditional ECU. These systems serve to minimise human errors by reducing reliance on humans by employing these computerised systems. Numerous studies conducted suggest that vehicles equipped with ADAS have a lower incidence of accidents (Ahmad et al., 2019; Fleetwood, 2017).
This model detailed by Ahmad et al., 2019 possesses the potential to be utilised in the EV industry. Built on the lines of ADAS, the system involves sensor input data and sends it into an algorithm trained to prioritise tasks based on criticality and importance to driver safety.
Impact weight: Sensors equipped in EVs inform us about the state of the vehicle. However, some pieces of information are more important for safety. For example, the external temperature might be good to know, but the condition of the tires and the presence of obstacles are more crucial to prevent accidents. Impact weight quantifies how significant the information displayed by the sensors is. It is a vector quantity represented by ω.
- Let S represent the vector representing the sensor’s readings for all scenarios. The value is normalised between 0 and 1 which implies minimum risk and maximum risk respectively.
- Then ARI is defined as the vector multiplication of impact weight ω and sensor reading given by the equation ARI=ωS_i.
- The total ARI is given by:
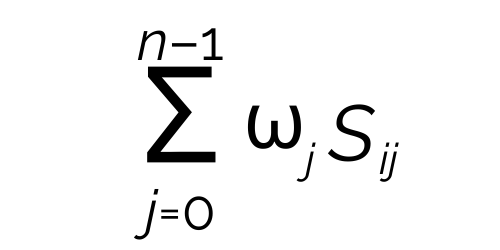
which is the weighted impact sensor reading. This is the aggregate of all the ARIs calculated using all possible
scenarios. If an unsafe state is described as per the sensor, then the necessary actuators take control to ensure safety.
- The value of S for a scenario is calculated as per the following formula:
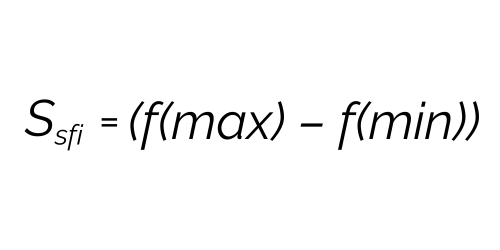
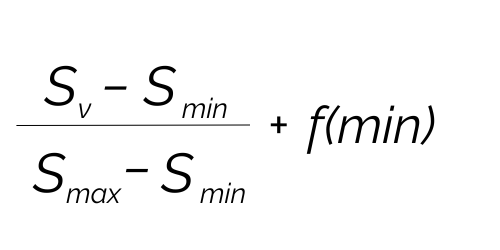
f(max) and f(min) refer to functions set as per the scenarios. The formula can be modelled as per the various scenarios
prevalent. This model takes into account the different scenarios like rainfall, windspeed, blurriness and surface friction.
Flow of operations involving the calculation of ARI and corresponding tasks to be taken:
- The sensor values are measured continuously.
- ARI values are then calculated using the formula.
- If the ARI surpasses a specific threshold, the algorithm discovers contributory circumstances (culprit scenarios) that increase the risk.
- The actuators select and perform corrective steps to mitigate the indicated risks.
- The process is repeated, with continual monitoring and adjustments to ensure safety.
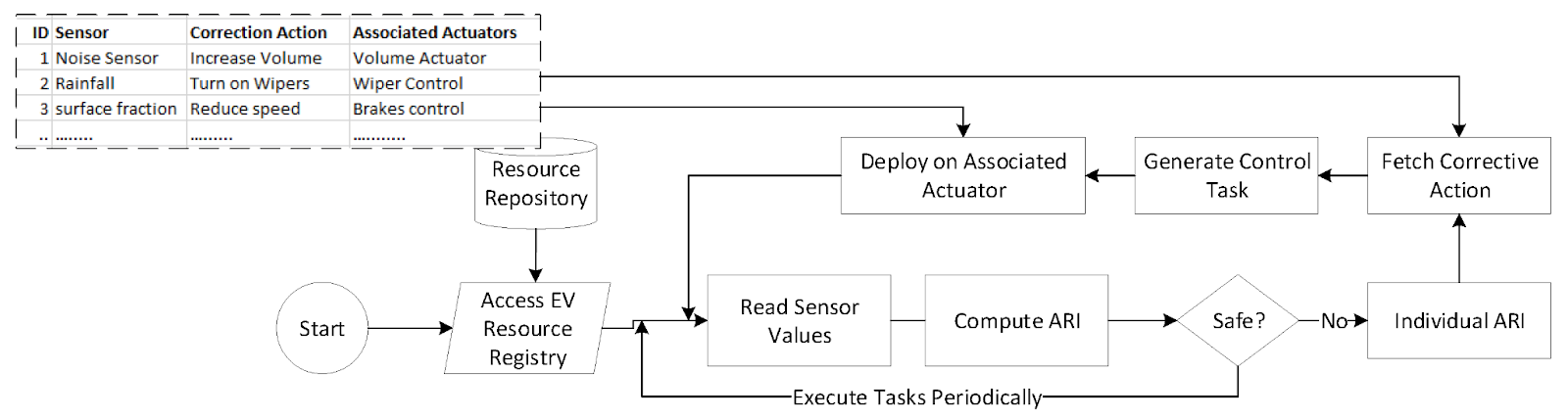
Figure 2: Tasks taken in response to ARI calculation (Ahmad et al., 2019)
5.1.2 Model for Estimating the Ergonomic Potential of Vehicles Using a Fuzzy Approach
Utilisation of this model in Dutta and Rathore, 2020 proposes a method that can be used for building an ergonomic rating index for any vehicle using a mathematical model. It is useful for a potential customer to access a car and compare various models before buying. Few ergonomic and anthropometric considerations are included with the model to provide a framework for building a user-centred vehicle considering the VoC (Voice of the Customer) and focused on human factor engineering.
There are many anthropometric attributes as written in Bhattacharyya and Saikia, 2024, but for this model, prioritising the key ergonomic factors helps in addressing the VoC. It might not be possible to consider all factors for this ergonomic rating index due to the budgetary constraints and time limitations of vehicle manufacturers. Dutta and Rathore, 2020 detail that to achieve prioritisation, relevant factors that contribute to safe and exhaustion-free driving were identified during the literature review.
5.1.2.1 Methodology for Data Collection and Prioritising Car Attributes
For the data collection in the report by Dutta and Rathore, 2020, a survey was conducted with a sample space including car users of different age groups and various backgrounds. The respondents were required to score the significance of each attribute on a scale of 1 to 5, providing insights into customer preferences and priorities. All the data was analysed using SPSS ver.16 and MS Excel software.
The responses given by the responders were used to generate the attribute importance rating by totalling the individual judgement. Fuzzy Logic or Triangular Fuzzy numbers were used to incorporate variability in the responses. Fuzzy Logic is useful to handle the situation of partial truth where there is no definite answer, rather a subjective response and where the truth may be between responses supporting completely true and completely false.
The membership function assists the Fuzzy Logic for this model. Membership function simply refers to a tool which helps us in determining how much of something belongs to a particular category. Usually, the membership function is graphed.
Example to understand Fuzzy Logic and Membership Function better:
Fuzzy Logic: Let us say we are deciding whether we are going to wear a jacket today. It is a bit chilly but not really cold. Conventional thinking would say that it will either be sufficiently cold to wear a jacket or not. However, Fuzzy Logic allows you to describe the weather as “kind of” chilly, implying that things are not quite as black-and-white as they seem.
Membership Function: Consider that we have a thermometer that goes from 0°C (freezing) to 40°C (hot). Suppose we wish to use Fuzzy Logic to define what cold means. To demonstrate that you could use a membership function.
- Below 10°C, it is very cold (high membership)
- Between 10°C and 20°C, it is somewhat chilly (medium membership).
- Above 20°C, it isn’t at all cold (low membership)
The amount of fuzziness of “being cold” can be quantified with this.
The actual formula in the report is explained by the following:
- Let us refer to the respondent’s judgement (i) on an attribute (j) as (w_ij). The attribute importance rating (AIR) was calculated by aggregating individual opinions.
- The aggregate judgement on judgement (j) is represented by triangular fuzzy numbers w_j = (l_(j)m_(j)u_(j))(TFN). TFN is defined by three parameters: l_j (the lower bound of judgements), m_j (the average of judgements) and u_j (the upper bound of judgements). This approach captures uncertainty by establishing a range (from l to u) in which the true value exists, with m being the most likely value.
- The membership function μ_w is reported as given.
- Converting a fuzzy number into a single distinct value is known as defuzzification. The method of graded mean integration representation is applied in this instance. The defuzzified value w_j is calculated via the formula given.
It considers the lower bound l_j and upper bound u_j while giving greater emphasis to the mean value m_j.
5.1.2.2 Computation of Attribute Score
- The AIR is used to determine the Normalised Importance of each Attribute (NIA), with the sum of NIA values of all equal to 1.
- Now for a particular variant of EV, the Ergonomic Score of an Attribute (ESA) is determined based on the degree of fulfilment of that specific attribute by that vehicle. ESA vehicles range from 0 to 5, with 0 meaning no while 5 referring to maximum fulfilment.
- The attributes are then grouped into three main categories:
1. Overall Safety Factor: Includes attributes pertaining to safety features, like airbags, brake assist, etc.
2. Musculoskeletal or Reach Factor: Includes attributes oriented toward operator and accessibility ease, like door control sensors, adjustable steering, etc.
3. Compatible Man-Machine Interface or Comfort Factor: Includes attributes related to comfort and usability, like seat adjustments, A/C efficiency, etc. - The AS which provides an overall evaluation of the AEV can be found with the product of NIA and ESA. This considers the fulfilment of attributes as well as their importance for the comfort and safety of a passenger.
5.1.2.3 Calculation of Factor Score (FS) in % and determining the output score of an EV
- The Factor Rating (FR) for each of the three ergonomic factors is the total of the AS values of all the factor attributes. An analogous concept is the Normalised Importance of Factor (NIF) which is the total of the NIA values for each attribute that makes up the factor.
- Finally using the given formula – which effectively normalises the FR by the NIF and scales it to a percentage – the Factor Score in percent (FS) for each factor is determined.
- Each of the three ergonomic factors receives a factor score (FS in %) for a certain brand and version of an automobile on a scale from 1 to 100, which serves as the model’s input. These inputs are fuzzified using fuzzy rule-based models for function approximation based on these scores. Mamdani FIS has been utilised for this research, and the car’s ergonomic score is the result of the FIS.
Mamdani FIS: It is a Fuzzy Inference System that utilises fuzzy sets of info and through defuzzification, produces a crisp output. It is well known for being widely accepted and intuitive.
Such data can be useful to manufacturers and automobile designers who might utilise this information to improve the ergonomic attributes of a car. This model will keep the automobile designers in check as they will be required to work on getting a competitive ergonomic score that will cause buyers to choose them over other vehicles.
5.2 Move to Modern AI
With the rise of deep learning and then neural networks, the fuzzy approach has been mostly supplanted by an AI approach instead, especially as large neural nets become more and more feasible for use within vehicle computers. As AI has already been implemented in the design of many vehicle subsystems, we are looking forward to the methodology that will help human-focused vehicle design, especially for EVs/AEVs.
5.2.1 Data Collection
The AI design implementation starts with driving data collection. The first pioneer in this field was Nissan. At that time, the energy consumption and range estimation algorithms almost all came from real-world user-driving data. Nowadays, all EV/AEV designs, including more and more ICE vehicle designs, have real-world driving data collection mechanisms integrated into the system in certain ways.
5.2.2 Autonomous Driving System
On the front of autonomous driving, Tesla is the first to try and implement it through its (FSD) program. FSD program initially has ECM equipped with thousands of lines of stack code. The initial stage of the stack system served as a test bed for many years to allow Tesla to collect real-world driving data to train its AI model by empowering neural networks to make decisions about the road as opposed to a system that follows strict rules, an approach that often found the car unable to perform well in the real world’s foreign situations (Brodsky, 2024). Gradually, Tesla merged the trained AI model with stack code in different road conditions until Version 12, when Tesla announced that it was the first to realise an end-to-end AI and computer vision-based autonomous driving system (Level 2). In addition, AI and neural networks can learn over time, rather than the static nature of 300,000 coded lines of C++.
5.2.3 From Fuzzy Logic to Modern AI Modelling
The fuzzy logic method was widely used in traditional ICE vehicle designs but the industry is now quickly moving to modern AI modelling-aided vehicle design. Nascimento et al., 2020 found that most of the current work in AI has moved away from fuzzy logic, with more research being performed in the areas of general AI and machine learning for a large system in AEVs, with fuzzy logic now being primarily supplanted by much more powerful statistical learning algorithms like convolutional neural networks (CNNs) and transformers.
5.3 Future of Power Propulsion Systems in EV
This is responsible for providing the traction force required to move the vehicle by converting electrical energy into mechanical energy. It consists of an energy storage system, the power converter, the propulsion motor, and associated controllers.
5.3.1 Understanding the basic terms and current market trends
Traditionally, silicon (Si) has been the choice of material for these switches used in insulated gate bipolar transistor (IGBT) traction inverters. Metal-oxide-semiconductor field-effect transistors (MOSFETs) made of Si limit the switching frequency to a value less than 100 kHz. These materials require one or two electron volts (eV) of energy to transport their electrons to the valence band to perform the conduction.
Semiconductor Switches: These are on-off controllers controlling the amount of power entering the electric motor which affects the efficiency and speed of the vehicle.
IGBTs and MOSFETs: Simply speaking, they are semiconductor devices used for controlling the flow of electricity and regulation of voltage.
Traction Inverter: Used for converting the direct current (DC) stored in the vehicle’s batteries into alternating current (AC) needed to power the electric motor.
Saturation Velocity: Maximum speed at which charge carriers in semiconductors can operate in high electric field conditions.
Critical Breakdown Electric Field: The dielectric breakdown happens at a threshold electric field strength known as the critical breakdown electrical field.
5.3.2 New technologies coming up in the industry
Any new developments in the field of EVs should prioritise a common focus on energy efficiency and environmental awareness. Several major directions for this development include improvements to the:
- Battery System: Moving from solid-state batteries to air batteries and then to fuel cells, which should be reconsidered to be EVs.
- Power Control System: We primarily discuss the semiconductors used in this system below; GaN and SiC (used in switches in traction inverters) should continue their usage into the development of fuel cells, instead of limiting their benefit to the lithium-ion battery.
- Metal Acquisition: In terms of raw materials, the reduction of rare metals is the primary task; the foremost research agenda should be on attempting to reduce and hopefully eliminate Co, Mn, and Ni from the manufacturing process.
SiC (silicon carbide) and GaN (gallium nitride) have wider band gaps that lead to higher electron mobility with less on-resistance. Carrying more current and switching faster than Si reduces energy losses in conversion. Moreover, a higher band gap allows the devices to operate at higher voltage (AstrodyneTDI). SiC and GaN can handle much higher switching frequencies up to 250 kHz. Higher frequencies mean more power enters the motor, which increases the efficiency of the converter. The usage of SiC and GaN has been increasing due to them offering better performance than Si. SiC and GaN can operate at higher temperatures but require careful thermal heat management to prevent overheating. SiC-based converters are known for their reliability, whereas GaN-based converters are known for their efficiency; however, detailed studies on GaN’s maintenance needs and reliability are needed for its optimal use (Fig 3A).
Currently, IGBTs and MOSFETs are used in present-day commercially available EVs, HEVs and PHEVs traction inverters. Si-based IGBTs and MOSFETs will remain the technology of choice until silicon carbide (SiC) and gallium nitride (GaN) based devices are commercially available at a price comparable to silicon IGBTs (Abro et al., 2023).
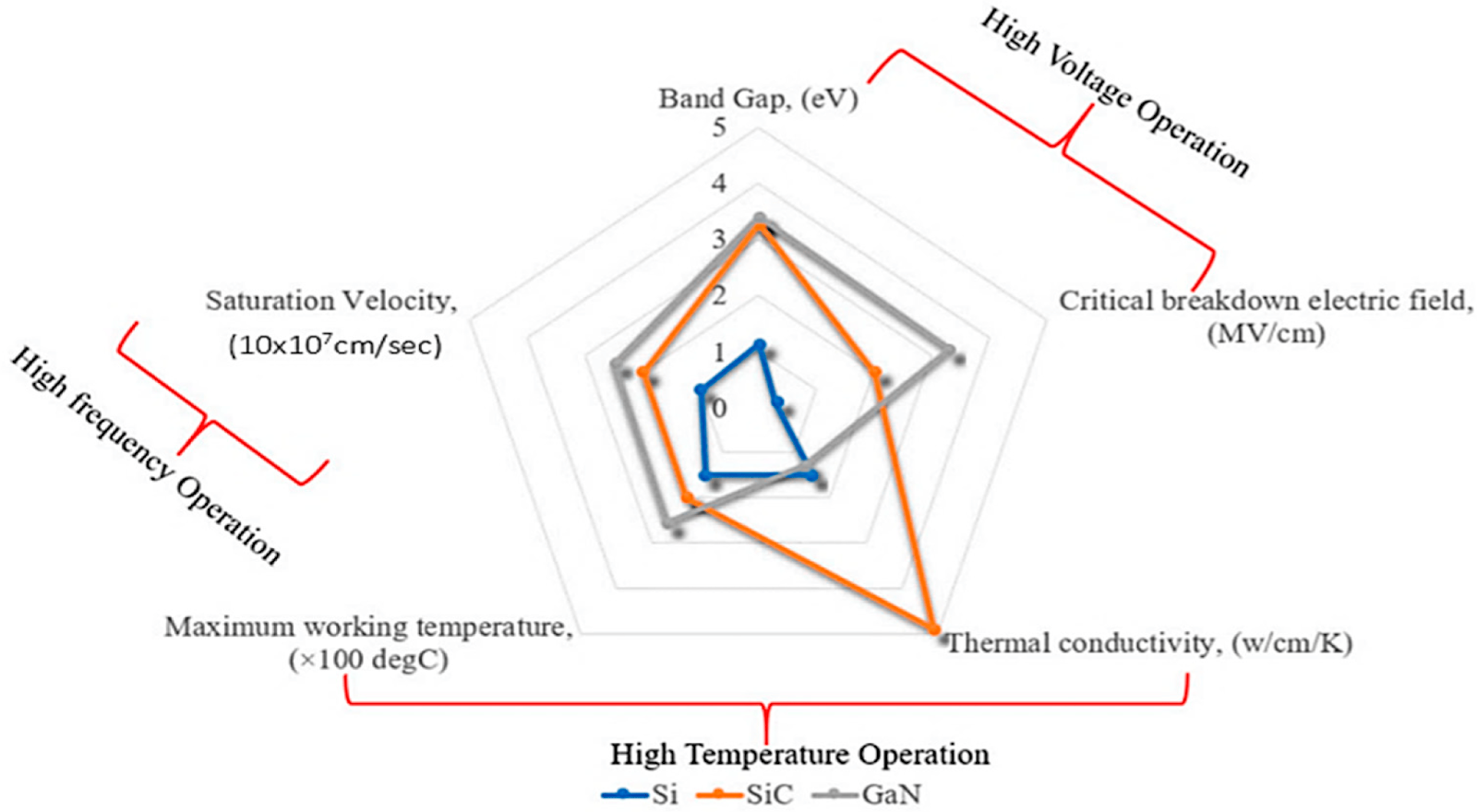
Figure 3A: Comparison between Si, SiC and GaN (Abro et al., 2023)
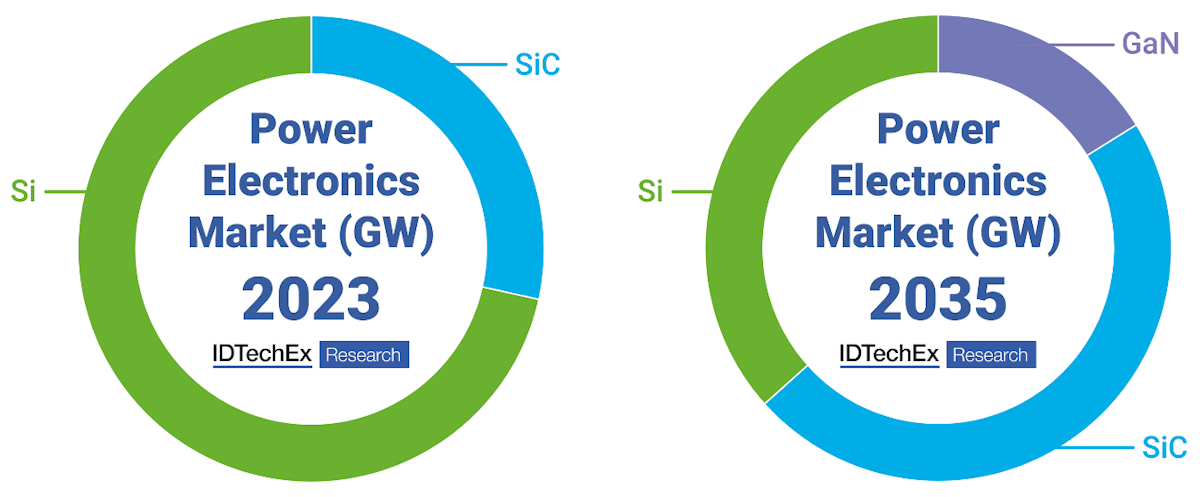
Figure 3B: Expected change in GW demand for Si, SiC, and GaN in the EV power electronics market from 2023-2035 (Li, J. 2024)
5.4 Future in Batteries
Lithium-ion batteries are the most commonly used batteries in an EV. Batteries play an important part in defining the EV’s range, performance and overall efficiency. Lithium-ion batteries have a high energy per unit of mass and volume when compared to other electrical energy storage technologies. They also offer a high power-to-weight ratio, excellent high-temperature performance, a long lifespan, and low self-discharge. The majority of lithium-ion battery components are recyclable, but the expense of material recovery remains a challenge for the business (U.S. Department of Energy). While battery usage safety has improved, they can still pose risks of thermal runaway, leading to fires or explosions under certain conditions.
Thermal Runaway: Thermal runaway refers to a situation where an increase in temperature leads to further increases in temperature, creating a self-accelerating feedback loop.
Example Case Study: On Christmas night 2023, a Tesla Model Y caught fire, causing an interstate highway in Alabama to be closed. A massive 136,000 litres of water was utilised to extinguish the fire. Due to thermal runaway, it was difficult to put out the fire, which is a big problem in EVs (Sharma, 2023b).
5.4.1 Solid-State Batteries
There are an anode and a cathode present, but the battery uses solid electrolytes instead of the liquid or polymer electrolytes found in conventional batteries. The anode is made of Li/V2O5 and is connected to a copper foil that increases the conductivity. The cathode can be made using the same compounds constituting the lithium-ion battery, such as V2O5 or LiMn2O4. There is a separator made of a polymer or ceramic material which also acts as a solid electrolyte. Examples include LiPON, LiBO2, etc. (Kim et al., 2015; Linda, 2022).
Functionality of solid-state batteries: During the discharge phase, lithium ions go from anode to cathode via the solid electrolyte, generating energy. This process is reversible: during charging, ions flow in the opposite direction and store energy in the battery. At the anode, lithium ions lose electrons before travelling to the cathode via the solid electrolyte. These ions mix with electrons at the cathode to form reduced ions, completing the circuit and producing energy.
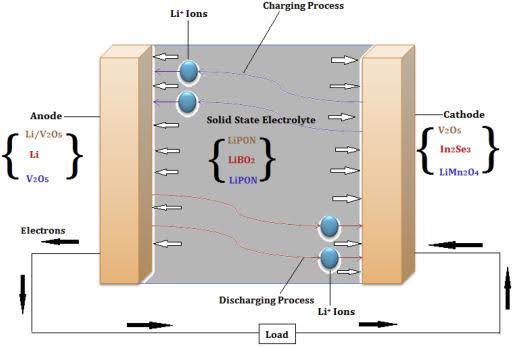
Figure 4: Functionality of solid-state batteries (Kim et al., 2015)
During the charging cycle, Li ions migrate to the anode for the recharging of cells. At the anode, lithium ions lose electrons before travelling to the cathode via the solid electrolyte. These ions mix with electrons at the cathode to form reduced ions, completing the circuit and producing energy. The Li anode may take up Li ions in the material, frequently intercalating between layers (also known as alloying or lithiation), or they can recombine with electrons, reducing to lithium metal that deposits in or on the surfaces, a process known as plating (Kim et al., 2015).
A major challenge in solid-state batteries: If a Li anode is employed, intercalation presents a difficulty, since the additional Li deposited produces metal swelling. The metal plating may be uneven, resulting in the creation of dendrites and spikes that can rupture the separator between the cathode and the anode, causing short circuits and deactivating the battery. A group of researchers at Harvard University created a composite anode material made of micrometre-sized silicon and graphite (Ye et al., 2024). This composite material protects lithium metal in the anode, avoiding dendrite development and allowing for increased energy density. The silicon particles take some lithium, forming a thin layer without crushing the particles, while the remaining lithium plates out uniformly. This technique combines the benefits of both intercalation and plating, perhaps presenting a solution to the limitations experienced by solid-state batteries (Kim et al., 2015; Linda, 2022).
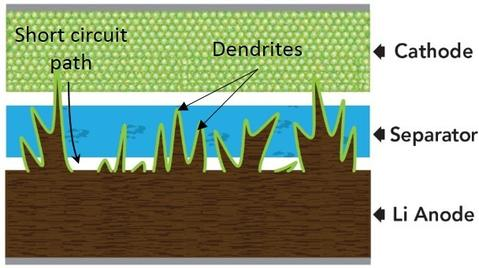
Figure 5: Model of dendrite growths in a lithium battery (MSE Supplies)
Why is it better than Li-ion batteries?
- Concerns over the safety and efficiency of lithium-ion batteries have intensified following a series of recent battery fires, underscoring the need for safer alternatives.
- In comparison to lithium-ion batteries, Solid-State Batteries (SSB) provide greater safety and efficiency since they use a non-flammable solid electrolyte in place of the volatile liquid electrolyte, thereby lowering the possibility of thermal accidents and fires.
- Issues with traditional liquid-state lithium-ion batteries include leakage, flammability of the electrolyte that may produce an explosion, dendritic development, and poor electrochemical window.
- For solid-state batteries, it is crucial to produce solid-state electrolytes with a large electrochemical window, high ionic conductivity, high ionic mobility, and low contact resistance between the electrolyte and electrode (Bates et al., 2022).
- Solid-state electrolytes found in SSBs include inorganic solid electrolytes, organic solid polymer electrolytes, and solid composite electrolytes. The inorganic materials, lithium aluminium titanium phosphate, have high ionic conductivity and thermal stability, but are mechanically fragile. The advantage of organic alternatives, polyethylene oxide and polyvinylidene fluoride, is flexibility, though they have the disadvantage of poorer ionic conductivity.
- Solid composite electrolytes combine the benefits of both inorganic and organic components, increasing mechanical durability and ionic conductivity.
- While composite electrolytes have made great progress, there are still problems in terms of synthesis complexity and material stability. The careful selection of these electrolytes is critical for developing robust and high-performance SSBs.
- Furthermore, while global SSB production capacity is currently less than 2 GWh, it is expected to rise at a compound annual growth rate of more than 118% by 2035, when the potential SSB market size will most likely approach 42 billion euros (Liu, 2023).
5.4.2 Lithium-Air Batteries
This type of battery utilises the oxidation of lithium at the anode and the reduction of oxygen at the cathode to generate electric current. It is an encouraging design because it has the theoretical ability to provide the highest specific energy of any type of battery, surpassing even gasoline in energy density on a weight basis.
Functionality of lithium-air batteries: For a non-aqueous lithium-air battery, there is a Li metal that gets oxidised and releases electrons at the anode. These Li ions combine with electrons and oxygen molecules to form lithium oxide (Li2O) or lithium peroxide (Li2O2). Theoretically, the batteries are rechargeable. Upon application of electricity, the lithium-charged species (stored in the cathode) turns back into the lithium metal and oxygen gas. The electrolyte is a dissolved lithium salt in an aprotic solvent and a porous O2-breathing cathode which is effectively carbon surrounded by O2 (Kribus and Epstein, 2021).
5.4.3 Self-Healing Batteries
Self-healing batteries, as the name suggests, have the capacity to recover from external stress that extends their operational lifetime and durability.
Functionality of Self-Healing Batteries:
There are two major components: Non-Metallic Charge Carriers and Hydrogel Matrices.
Non-Metallic Charge Carriers: Researchers have turned to the usage of ammonium ions to support the self-healing mechanism since they do not react strongly with electrode materials that may potentially damage the battery’s performance over time.
Hydrogel Matrix: Hydrogels like polyvinyl alcohol possess the ability to absorb and retain huge amounts of water lending flexibility and self-healing properties, protecting the battery from physical stress or tension. The inclusion of ammonium salts ensures the dynamic functioning of such batteries in real-life scenarios by providing mechanical sturdiness and adaptability (Guerra, 2024).
The electrodes of this battery incorporate polymers or other materials which can form dynamic covalent bonds. These bonds can break and rebuild under particular conditions, allowing the electrode material to repair itself after being damaged. For example, silicon microparticle anodes with self-healing binders have emerged to boost the stability of high-energy lithium-ion batteries. These binders can repair cracks and keep electrical contact between particles, considerably increasing the battery’s cycle life (Cheng et al., 2022).
Dynamic Covalent Bonds (DCC): Reversible covalent bonds have been recognised as a powerful tool in constructing surface covalent nanostructures at solid-liquid interfaces (Imato and Otsuka, 2018).
5.4.4 Fuel Cell Technology
In the long term, EVs/AEVs based on fuel cell technology will gradually widen applications as research and development endeavours continue. Fuel cell technology will inherit most of the EV/AEV developments we discussed here and provide further revolutionary progress in the transportation industry. The current example is Toyota’s Mirai, which is a hydrogen fuel cell EV.
5.5 Expansion of Terminology Regarding Energy Origin
The terminology of autonomous electric vehicles is frequently limited to purely those vehicles which have a solid lithium-ion traction battery buried at the bottom of the vehicle. However, all hydrogen-based cars and all other cars that have fuel cells should be considered as electric as they all involve the generation of electricity, whereas the internal combustion engine requires burning fuel and oxidation to achieve motion. Fuel cells, in fact, would be able to have a more flexible design once they enter the market as their required weight could potentially be less than even ICE cars.
6. Public Opinion
- Research indicates that drivers are hesitant to adopt self-driving autonomous vehicles (AVs), even with active safety measures like warning systems. The benefits of autonomous vehicles, such as improved safety and fuel efficiency, may not be enough to drive broad use. Raising public awareness can accelerate the adoption and improvement of AV technology. According to a survey by Aldakkhelallah et al., 2023, 22% of respondents would not pay for full automation, while 5% would pay more than $30,000. 33% found the notion very enjoyable. 69% believe that AVs will account for 50% of the market by 2050.
- The majority of respondents supported partial automation (level 4: 36.11%, level 3: 24.07%) over fully autonomous cars (level 5), citing the high prices of new technology and AV usage as barriers to adoption.
- The public views AVs as beneficial for safety, the environment, time efficiency, reduced congestion, comfort and convenience, economic savings, increased productivity, less human error, energy efficiency, and improved traffic systems. The benefits of introducing AVs are widely anticipated to be valuable.
- Public concerns about AVs include system malfunctions, cybersecurity threats, AI decision-making, infrastructure issues, ethical dilemmas, loss of human control, and potential job losses.
- AVs have the potential to transform transportation by improving safety, efficiency, and sustainability. They align with worldwide efforts such as the EU’s prohibition on new ICE car sales by 2035, indicating a seamless integration of AVs and EVs in the coming decade (Aldakkhelallah et al., 2023).
- Barriers, such as the lack of available and current charging stations, may impede the transition to autonomous vehicles. The most common EV charging stations only deliver 2 kW of power, creating impediments to adoption. AEVs rely on advanced sensors and computers, therefore conventional stations may not be sufficient.
- Adequate charging facilities are necessary to enable autonomous vehicles, which have a preferred driving range of 200-400 km according to survey data. Respondents raised concerns about the scarcity of maintenance shops for electric and self-driving vehicles, demonstrating a current lack of confidence in these technologies.
- Collaboration between automakers and municipal planners is vital for assessing the impact of autonomous vehicles on traffic systems and addressing cybersecurity issues. To build trust and understanding in AV decision-making, manufacturers should do rigorous testing and share their findings.
- The survey highlights the need for effective marketing and communication efforts to educate the public about the features and benefits of AVs. Approximately 70% of respondents are ignorant of AV classifications.
- Although battery efficiency has improved, charging time and driving range remain key impediments to EV adoption, with commercial cars averaging roughly 200 miles (~320 km) per charge.
- A survey revealed that self-driving cars require advertisements and education. Automakers should explore motivating consumers to buy AVs to enhance market penetration. Motivations can include tax credits and safety. Marketing should emphasise that AVs are trustworthy and environmentally conscious (with limited CO2 emissions). Public decision-making can be heavily influenced by cost.
- Successful integration of AVs requires tackling cybersecurity threats and ethical decision-making, which have a direct influence on human lives and public acceptance (Alsalman et al., 2021).
7. Limitations
- Inadequate Time for Extensive Research: Conducting comprehensive research within a month can be difficult given the broad breadth of autonomous cars and human-centred design concepts. This restriction has an impact on the scope and depth of the literature review, which may result in understanding gaps in the most recent advancements and complex facets of the subject.
- Restricted Expert Guidance Access: Getting expansive feedback and direction on our study methods, outcomes, and recommendations in just twelve hours of coaching and mentoring sessions has been a significant challenge for us.
- Breadth and Depth Harmony: A balance between breadth and depth is needed to address both the technical and human-centred components of autonomous vehicle design. It was challenging to strike this balance in the allotted time.
- Delivering Originality and Quality: Sustaining the standard of the research and guaranteeing novel contributions to the area might be difficult under time restrictions.
8. Future Work
- The HTA will be expanded to include more concise steps, taking into account smaller subtasks that may demonstrate differences between ICE vehicles and EVs. Greater analysis will be done to determine the possibility of making all Tesla vehicles accessible for all users, including persons with reduced mobility.
- We hope to continue our work in this domain and make more recommendations to engineers and designers in the field to keep human ergonomics in mind in the next generation of autonomous electric vehicles to hit the market.
- Large-scale surveys and research will be conducted to obtain information about user preferences, behaviours, and expectations for AEVs. This empirical research can help lead the creation of more targeted and effective human-centred design principles.
- The integration of emerging technologies, such as AI and machine learning algorithms, with human-centred design principles will be investigated to enhance the AEV experience. This could incorporate adaptive interfaces and personalised safety features.
- Multidisciplinary collaboration among academics in psychology, sociology, ergonomics, and electrical engineering will be encouraged. Such cooperation may result in novel methods to build AEVs that actually suit the demands of all users.
- AEVs’ long-term sustainability and environmental impact will be evaluated, taking into account both their lifecycle and the broader implications on urban settings and ecosystems. This could help to produce greener and more sustainable AEV designs.
9. Conclusion
- In the scientific research paper Human-Centred Design Improvements for Autonomous Self-Driving Vehicles, we focus on enhancing the user experience and safety of autonomous electric vehicles (AEVs). We embarked on an extensive journey to understand the user requirements, propose user-centred design improvements, and investigate the future of power propulsion systems and battery technology.
- The study investigates the relationship between technological improvements and human needs, emphasising the necessity for design methods that prioritise user comfort, safety and inclusivity. Our study encompasses diverse aspects of AEV design and functionality, from HTA to proposing a model for estimating the Ergonomic Potential of vehicles using a Fuzzy Approach and the Driver Assistance Model for Accident Risk Index.
- The paper also highlights the potential and comparison of solid-state batteries, lithium-air batteries, and self-healing batteries as the future of energy storage in electric vehicles. We detailed the future of power propulsion systems wherein SiC and GaN take over traditional Si semi-conductors, thus integrating AEVs with technological advancements.
- As we wrap up this study, we imagine a future in which AEVs smoothly blend safety, comfort and sustainability, while addressing the varying needs of users from all backgrounds. We believe that this work will spur additional research and innovation, paving the road for a transportation landscape that prioritises human needs and experiences. Further, the article emphasises the relevance of public opinion in addressing shortcomings and upsides related with the use of autonomous vehicles. The primary goal of the research is to bridge the knowledge divide between the human factors required for mainstream adoption of AEVs and technological advancements in these vehicles.
10. Acknowledgements
The authors would like to thank our advisor, Liam Obuobie, for guiding and mentoring us during this project and Oxford Scholastica for providing an opportunity to publish this research work. We also thank Ms. Stephanie Lester for organising this programme and allowing young students to contribute to a wide variety of types of academic research.
Bibliography
Abro, G. E. M., Zulkifli, S. A. B. M., Kumar, K., El Ouanjli, N., Asirvadam, V. S., & Mossa, M. A. (2023). “Comprehensive Review of Recent Advancements in Battery Technology, Propulsion, Power Interfaces, and Vehicle Network Systems for Intelligent Autonomous and Connected Electric Vehicles”. Energies, 16 (6). https://doi.org/10.3390/en16062925.
Ahmad, S., Malik, S., Park, D.-H., & Kim, D. (2019). “Design of Lightweight Driver-Assistance System for Safe Driving in Electric Vehicles”. Sensors, 19 (21). https://doi.org/10.3390/s19214761.
Aldakkhelallah A, Alamri AS, Georgiou S, Simic M. (2023). “Public Perception of the Introduction of Autonomous Vehicles”. World Electric Vehicle Journal. 14 (12): 345. https://doi.org/10.3390/wevj14120345.
Alsalman, A., Assi, L. N., Ghotbi, S., Ghahari, S., & Shubbar, A. (2021). “Users, planners, and governments perspectives: A public survey on autonomous vehicles future advancements”. Transportation Engineering, 3, 100044. https://doi.org/10.1016/j.treng.2020.100044.
Anderson, M. (2024, June 30). “Human factors engineering”. Human Factors 101. https://humanfactors101.com/topics/human-factors-engineering/.
AstrodyneTDI (n.d.). “Advantages of wide bandgap semiconductors”. AstrodyneTDI. https://www.astrodynetdi.com/blog/what-is-the-future-for-gan-and-sic-in-the-semiconductor-industry
Bates, A. M., Preger, Y., Torres-Castro, L., Harrison, K. L., Harris, S. J., & Hewson, J. (2022). “Are solid-state batteries safer than lithium-ion batteries?”. Joule, 6(4): 742–755. https://doi.org/10.1016/j.joule.2022.02.007.
Bhattacharyya, N., & Saikia, H. (2024). “ANTHROPOMETRY IN INTERIOR DESIGN”. In Noble Science Press eBooks. https://doi.org/10.52458/9788196897444.nsp2024.eb.ch-19.
Bhatnagar, P., Gururaj, H., Shreyas, J., & Aithal, H. (2024). “ElectroPath: A Predictive Model for Electric Vehicle Range Optimization Through Mobile Application”. In 2024 International Conference on Emerging Technologies in Computer Science for Interdisciplinary Applications (ICETCS): 1-6. https://doi.org/10.1109/ICETCS61022.2024.10543641.
Brooks, J., Mims, L., Jenkins, C., Lucaciu, D. et al. (2018). “A User-Centered Design Exploration of Fully Autonomous Vehicles’ Passenger Compartments for At-Risk Populations,” SAE Technical Paper 2018-01-1318.https://doi.org/10.4271/2018-01-1318.
Chen, F. (2020). “Special Issue on HMI and Autonomous Driving. Automot”. Innov. 3: 1–2. https://doi.org/10.1007/s42154-020-00094-1.
Cheng, Y., Wang, C., Kang, F., & He, Y.-B. (2022). “Self-Healable Lithium-Ion Batteries: A Review”. Nanomaterials, 12 (20). https://doi.org/10.3390/nano12203656.
Dai, D., Chen, Z., Bao, P., & Wang, J. (2021). “A Review of 3D Object Detection for Autonomous Driving of Electric Vehicles”. World Electric Vehicle Journal, 12 (3). https://doi.org/10.3390/wevj12030139.
DeLuchi, M., Wang, Q., & Sperling, D. (1989). “Electric vehicles: Performance, life-cycle costs, emissions, and recharging requirements”. Transportation Research Part A: General, 23 (3): 255–278. https://doi.org/10.1016/0191-2607(89)90007-1.
Dutta, A., & Rathore, A. P. S. (2020). “Estimating Ergonomic Compatibility of Cars: A Fuzzy Approach”. Procedia Computer Science, 167: 506–515. https://doi.org/10.1016/j.procs.2020.03.270.
Fleetwood, J. (2017). “Public Health, Ethics, and Autonomous Vehicles”. American Journal of Public Health, 107 (4): 532–537. https://doi.org/10.2105/AJPH.2016.303628.
Fridman, L. (2018). Human-centered autonomous vehicle systems: Principles of effective shared autonomy. https://doi.org/10.48550/arXiv.1810.01835.
Gao Jin, N. (2014). “Robust optimization and sensitivity analysis of hardpoints on suspension characteristics and full vehicle handling performance”. Journal of Jiangsu University (Natural Science Edition), 35 (3): 249.
Guerra, M. (2024, June 7). “Self-healing flexible batteries: Safer and better for wearables”. Battery Tech. https://www.batterytechonline.com/materials/self-healing-flexible-batteries-safer-and-better-for-wearables.
Hawkins, T.R., Gausen, O.M. & Strømman, A.H. (2012). “Environmental impacts of hybrid and electric vehicles—a review”. Int J Life Cycle Assess 17: 997–1014. https://doi.org/10.1007/s11367-012-0440-9.
Imato, K., & Otsuka, H. (2018). “Reorganizable and stimuli-responsive polymers based on dynamic carbon–carbon linkages in diarylbibenzofuranones”. Polymer, 137: 395–413. https://doi.org/10.1016/j.polymer.2018.01.038.
Kim, J. G., Son, B., Mukherjee, S., Schuppert, N., Bates, A., Kwon, O., Park, S. (2015). “A review of lithium and non-lithium based solid state batteries”. Journal of Power Sources, 282: 299–322. https://doi.org/10.1016/j.jpowsour.2015.02.054.
Kribus, A., & Epstein, M. (2021). “Chapter 2 – Thermochemically regenerative flow batteries for solar electricity generation and storage”. In A. Datas (Ed.), Ultra-High Temperature Thermal Energy Storage, Transfer and Conversion: 35–56. https://doi.org/10.1016/b978-0-12-819955-8.00002-8.
Li, J. (2024, June 3). “Power Electronics for Electric Vehicles 2025-2035: Technologies, markets, and forecast: IDTechEX”. IDTechEx. https://www.idtechex.com/en/research-report/power-electronics-for-electric-vehicles-2025-2035-technologies-markets-and-forecasts/1014
Liu, M. (2023). “Development and challenges of solid-state lithium-ion batteries.” Applied and Computational Engineering, 23: 197-205. https://doi.org/10.54254/2755-2721/23/20230652.
Linda. (2022, March 14). “Solid-state batteries: the new frontier of electrification?”. Flash Battery. https://www.flashbattery.tech/en/how-solid-state-batteries-work/
MSE Supplies. (2019, October 17). “Source of detrimental dendrite growth in lithium batteries discovered”. MSE Supplies LLC. https://www.msesupplies.com/blogs/news/source-of-detrimental-dendrite-growth-in-lithium-batteries-discovered.
Park, C., & Kee, S.-C. (2021). “Online Local Path Planning on the Campus Environment for Autonomous Driving Considering Road Constraints and Multiple Obstacles”. Applied Sciences, 11(9). https://doi.org/10.3390/app11093909.
Patil, H., & Kalkhambkar, V. (2021). “Grid Integration of Electric Vehicles for Economic Benefits: A Review”. Journal of Modern Power Systems and Clean Energy, 9(1): 13-26. https://doi.org/10.35833/MPCE.2019.000326.
Rahmati Y, Samimi Abianeh A, Tabesh M and Talebpour A (2021). “Toward Human-Centered Design of Automated Vehicles: A Naturalistic Brake Policy”. Front. Future Transp. 2:683223. https://doi.org/10.3389/ffutr.2021.683223.
Sharma,Y. (2023, December 28). “1,36,000 litres of water used to put out Tesla EV fire! How solid-state batteries can redefine safety”. The Times of India. https://timesofindia.indiatimes.com/auto/electric-cars/136000-litres-of-water-used-to-put-out-tesla-ev-fire-how-solid-state-batteries-can-redefine-safety/articleshow/106347405.cms
Ulrich, K. T. (2005). “Estimating the technology frontier for personal electric vehicles”. Transportation Research Part C: Emerging Technologies, 13(5): 448–462. https://doi.org/10.1016/j.trc.2006.01.002.
U.S. Department of Energy. (n.d.). “Batteries for Electric Vehicles. Alternative Fuels Data Center: Batteries for Electric Vehicles”. https://afdc.energy.gov/vehicles/electric-batteries.
Welsh, D., Gomez, A., & Pierce, J. (2018). “Designing an Interior and Exterior Acoustical Brand Identity for Electric Vehicles by Means of Sound Synthesis”. INTER-NOISE and NOISE-CON Congress and Conference Proceedings, 258 (4): 3557-3568.
Yalda Rahmati and Alireza Talebpour. 2017. “Towards a collaborative connected, automated driving environment: A game theory based decision framework for unprotected left turn maneuvers”. In 2017 IEEE Intelligent Vehicles Symposium (IV). IEEE Press: 1316–1321. https://doi.org/10.1109/IVS.2017.7995894.
Ye, L., Lu, Y., Wang, Y. et al. (2024). “Fast cycling of lithium metal in solid-state batteries by constriction-susceptible anode materials”. Nat. Mater., 23: 244–251 . https://doi.org/10.1038/s41563-023-01722-x.