Supervised by: Dr Callum Parr MBBS BSc (Hons). Callum studied Medicine at Imperial College London and secured a place as an Academic Foundation Doctor in the competitive North West London region. He received multiple distinctions for his performance throughout medical school. He intercalated in Biomedical Engineering, also at Imperial College London, and received First Class Honours. He has published work in prestigious journals, including the Lancet Respiratory and BMJ.
Abstract
Cardiology is the field of medicine involving prognosis, diagnosis, and treatment of disorders relating to the heart. Artificial intelligence (AI) is gaining prevalence throughout this field, with applications ranging from cardiac risk assessment, early detection of cardiac disease, and treatment for disorders of the heart. Various AI technologies are able to perform these functions accurately and with minimal human input. The usage of AI throughout medicine underscores the significance of technology in delivering accurate, personalised healthcare. Ethically, however, artificial intelligence raises concern regarding patient privacy, reliability, and the reasoning process of the AI model. Doctors and researchers alike must find a way to balance medical opportunity and ethical dilemmas to ensure safe and secure usage. The accuracy and demographic generalisation of AI technologies, especially when analysing patients with genetic or disease-related abnormalities, must also occur for the widespread usage of AI in healthcare to occur.
Introduction
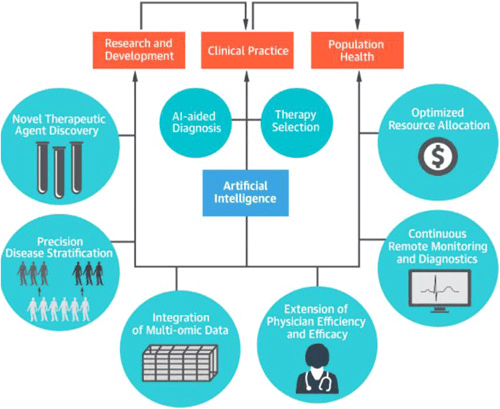
Figure 1: Applications of Artificial Intelligence in Cardiology (1)
Cardiology is a medical speciality that deals with the diagnosis and treatment of the heart. AI is currently a leading tool in this field due to its capabilities of data synthesis and analysis (2). Typically, a cardiologist would manually assess, analyse, and diagnose a patient. However, human diagnoses are frequently variable, inaccurate, and unreliable, all of which can cost a patient their life. Thus, the application of AI in cardiology is quickly growing. Recent studies have demonstrated that AI has the ability to outperform a trained cardiologist and accurately predict oncoming cardiac episodes. The speed of an AI system is also extremely promising and points to a growing emphasis on early detection and disease prevention. The future of AI in cardiology and in medicine remains bright as the collaboration between technology, researchers, and clinicians continues to improve (2). “The promise of artificial intelligence and machine learning in cardiology is to provide a set of tools to augment and extend the effectiveness of a cardiologist” (3). That is, AI will not “replace” doctors in a traditional sense, but rather aid doctors in diagnostic duties and supplement the decision-making process of a doctor.
Cardiovascular disease is currently the leading cause of premature death in both the United States of America and the United Kingdom (4, 5). Globally, heart diseases are responsible for the death of 17.9 million people each year (6). The ability of an AI to quickly process and respond to data can expedite disease detection and provide a more personalised healthcare experience. All aspects of cardiology can similarly benefit with the integration of AI. The applications of AI in diagnosis and treatment, as well as the ethical concerns of AI in cardiology, will be explored.
AI in Cardiac Disease Diagnosis
Using Retinal Scans to Predict the Onset of Myocardial Infarctions
Throughout ophthalmology, retinal scans are routinely obtained to ensure proper eye function and detect the onset of eye disease (7). Recent studies have demonstrated links between retinal biomarkers and cardiac function. Retinal blood vessel density and retinal vascular tortuosity—that is, the abnormal curvature of blood vessels (8)—can aid doctors in identifying patients at risk for cardiac disease and, more specifically, myocardial infarctions (7). Myocardial infarctions occur due to a lack of blood flow to the heart, causing insurmountable damage to the heart muscle (9). Myocardial infarctions cause chest pain, shortness of breath, dizziness, and often lead to death when not identified in a timely manner (9). A combination of AI, patient diagnostics, and the retinal imaging tools already in use by opticians can assess high-risk patients with an accuracy between 70% to 80% (10). This promising technique can have an impactful application in eye clinics as a secondary referral tool to identify those in need of specialised treatment.
The use of AI to analyse retinal scans could revolutionise the cardiac screening process and decrease the 17.9 million individuals who die due to cardiovascular disease (6). The early identification of individuals at risk of myocardial infarctions can provide an opportunity for preventative treatment and referral to a cardiologist before a heart attack occurs. Early detection can also protect individuals against the lifelong complications that follow a cardiac episode.
Applications:
The use of AI in detecting cardiac disease can make screening a more accessible and affordable process. Retinal scans are relatively inexpensive and easy to obtain, an added bonus that will make cardiac screening available to a larger demographic (7). Additionally, this method is less invasive than traditional screening methods, such as heart traces or heart scans, the latter of which involves exposure to potentially harmful x-rays (11).
To analyse retinal scans, the AI utilises a specific subset of machine learning: deep learning (7). The deep learning (DL) algorithmic model closely mimics that of a human neural network, allowing the AI to produce an output similar to that of a human (12). DL is especially beneficial in this application as the algorithm does not require that the input data be pre-processed (12). The AI simply receives the raw input data—much like a human would—and utilises past information and new inferences to make a prediction (12). In this case, the deep learning AI was trained using retinal scans and demographic data from 5,663 patients (7). The AI then used this data to estimate an individual’s risk of experiencing a myocardial infarction within the year (10). Instead of directly anticipating future myocardial infarctions, the AI was designed to detect changes in left ventricular mass (LV) and left ventricular end-diastolic volume (LVEDV) as an intermediary step (7). LV is the weight of the left ventricle of the heart and is closely indicative of the impact of blood pressure on the heart (13). A higher LV is indicative of increased stress on the heart due to blood pressure, an important biomarker of cardiac risk (13). LVEDV describes the amount of blood in the left ventricle of the heart measured the moment before the heart contracts (14). A healthy, effectively-working heart is able to efficiently push blood through the ventricles (14). Thus, a low LVEDV is demonstrative of efficient heart function and blood flow (14). An increase in both LV and LVEDV are indicative of cardiac disease as the enlargement of the left ventricle reduces heart-pumping efficiency and increases blood pressure (11). This enables the technology to not only predict cardiac episodes and identify at-risk individuals, but also to generally assess left ventricular performance and function (7). This approach also provides a reasoning behind the predicted result, enhancing transparency in the decision-making process of the AI (7).
Challenges and Future Directions:
Despite the progress of this AI, the technology still requires a great deal of development. Currently, the AI experiences a drop in effectiveness when used on patients with a higher LV and LVEDV (10). The AI model has not yet been trained for patients with these and other abnormalities, and retinal scans unfortunately lack some of the data needed to assess cardiac function (10). Individuals with diabetes were notably excluded from this database as this condition also causes changes to retinal blood vessels (7). When the algorithm was tested on patients with age-related macular degeneration (AMD), a decrease in accuracy was observed. AMD results in changes in the retina, specifically in terms of retinal pigmentation and macular anatomy (7). Some of the most important retinal biomarkers are found by analysing retinal vessels and AMD results in substantial changes to the features of the retinal vascular system (7). Thus, the presence of AMD impedes the AI’s predictive power (7). Moving forward, the AI will require input data sets from a wider variety of patients to ensure accuracy throughout diverse populations.
This case study provides a recent and relevant example of the medical applications of AI. This and other AI models are crucial to advancements in healthcare and delivering a more personalised healthcare experience.
Wearable Devices in Cardiovascular Care
The persistent scientific progress of the human race has allowed the invention of wearable fitness technology, allowing consumers to monitor their own health and keep track of their own vital signs (15). Devices like smartwatches, rings, and wristbands have helped clinicians glean new insights into cardiovascular care due to the various sensors embedded in the devices (15). Even after receiving treatments in the hospital, lifestyle-related changes and regular monitoring of illness are very important in managing established cardiovascular conditions (16). Thus, the development of algorithms and sensors in wearable devices will undeniably expand cardiology’s horizons in terms of disease prevention, diagnosis and management (17).
Wearable sensors and cardiovascular applications:
With health monitoring gradually becoming more accessible, it is useful for consumers to be aware of some of the most common sensors behind data processing of their wearable devices (18). These sensors provide the necessary input data to make AI algorithms possible.
Photoplethysmography sensors function as the heart of smartwatch technology. Photoplethysmography (PPG) is a non-invasive technique involving the usage of infrared light to detect volumetric changes of blood in peripheral circulation (19). Biometric PPG sensors detect heart rate and rhythm and are typically found at the back of smartwatches. Here, high-intensity green light-emitting diodes (LEDs) and photodetectors help monitor cardiac functions like pulse rate. The LED light is differentially absorbed and reflected off tissues. The variation in light intensity through a series of wave and peak detection is associated with changes in blood flow (19). Oxygen saturation (SaO2) is also one of the most common applications of PPG technology. This application is able to detect whether users have the necessary amounts of blood oxygen, a key component of cardiovascular health assessment (20). Good oxygen saturation is vital in ensuring the critical functioning of bodily organs, ensuring the sustainability of metabolic reactions. PPG sensors in smartwatches play significant roles in many areas relating to cardiovascular health. These sensors aid in the aftercare treatment and monitoring of cardiovascular diseases and heart failure management (20). In addition, PPG sensors help patients and doctors analyse the data collected by AI to spot cardiac abnormalities and perform risk prediction in healthy individuals or those with established cardiovascular disease (20). Atrial fibrillation (A-fib) is an irregular heart rhythm that can lead to blood clots in the heart, dramatically increasing the risk of stroke, heart failure, and other heart-related issues. A progression model in a recent study projected the trend and growth rate of A-fib, demonstrating that the incidence of A-fib is expected to double from 1.2 million cases in 2010 to 2.6 million cases in 2030 (21). Accessories like smartwatches and smart rings utilise AI to predict and provide notifications of this condition using PPG to detect pulse irregularities (22).
Consumer grade wearable devices also show potential in measuring other factors important to cardiologists like physical activity (PA), examined through an accelerometer. An accelerometer is an activity sensor measuring the acceleration of objects. An accelerometer is useful for measuring PA as the sensor also shows the intensity and frequency of movements made by an individual. By integrating accelerometer data with respect to time, velocity and displacement can also be obtained (24). Such sensors are often found in medical earbuds, chest straps, smart rings, and clothing and shoe-embedded sensors. Maintaining a healthy weight is essential to heart disease prevention, as increased body weight is linked to increased blood pressure and high cholesterol, all factors contributing to heart disease. Physical activity, coupled with a healthy diet, can help an individual achieve this healthy weight. Accelerometers in wearable activity monitors have been revolutionary in terms of increasing physical activity for those who are undergoing cardiac rehabilitation, as suggested by recent systematic reviews24. Peripheral vascular disease is a blood circulation disorder that causes the narrowing, blockage or spasming of blood vessels outside of one’s heart and brain. Treatment involves a supervised exercise programme that is initiated with gradual intensity. Two randomised trials of patients with peripheral vascular disease showed that accelerometer devices, accompanied by wearable-guided exercise prescriptions, can help to enhance walking ability (25).
Challenges and Future Directions:
Wearable devices play a valuable role in diagnostic applications, risk assessment, and lifestyle intervention. The role of WAMs (wearable accelerometer-based sensors) in clinical practice will most likely grow as community-based health services become more prominent (26). However, the full potential of AI in cardiology has not yet been realised. The lack of coordination across the healthcare system as well as the questions surrounding the ethical barriers of wearable devices greatly prevents the widespread implementation of AI. Thus, discussion revolving around the challenges and future direction of AI in cardiology are needed to move towards the democratisation of health.
AI in Cardiac Disease Treatment
AI in Surgery
AI can do wonders for surgeons and patients when it comes to making better predictions and decisions; AI can quickly learn from and sort through vast amounts of data with a deep learning model. The DL model assesses areas of data ranging from patient history, values, anatomy images to risk factors (27). Digital surgery (London)—a company that explores the applications of technology, robotics, and AI in a surgical setting—have recently patented a successful AI platform, which the company claims can produce surgical procedure roadmaps in order to make surgeries safer (29). In addition to planning and decision-making, autonomous robotic surgery suggests the idea of surgeons overseeing robotic surgery in the near future. Current robotic surgical devices possess no intelligence and serve, primarily, to improve precision, control, and flexibility (30). In this regard, AI robotic surgery would greatly revolutionise cardiology, combining the accuracy of robotics with the intelligent assistance capabilities of AI.
Robotic Surgery and Cardiology
With the help of machine learning (ML), robotic surgical skills could be learned through demonstration by experienced surgeons or the modelling of human movement through sets of recorded data (30, 29). The DaVinciTM system is an advanced robotic platform that makes it possible for surgeons to now perform less invasive procedures (surgical procedures that require incisions and appreciably alter skin or underlying tissues at the time of the procedure). Before the docking of the DaVinci™ system (Intuitive Surgical, Inc., Sunnyvale, CA, USA), markings were made on the patient’s thorax for a trocar (a medical device used in laparoscopic procedures) introduction (Figure 2) (32). The DaVinci™ system (Intuitive Surgical, Inc., Sunnyvale, CA, USA) was used to operate on all patients who gave consent to have the surgery performed and have their data to be recorded and evaluated in the project conducted by Poffo et al. The system consisted of four robotic arms, an image capture and recording system, and a console through which the surgeon could control the movements of the robot (31). According to Poffo et al., robotic-assisted cardiac surgery proved to be safe and effective to be applied in the correction of intra and extracardiac pathologies, supported by the positive surgical outcomes and excellent secondary aesthetic results obtained from the study.
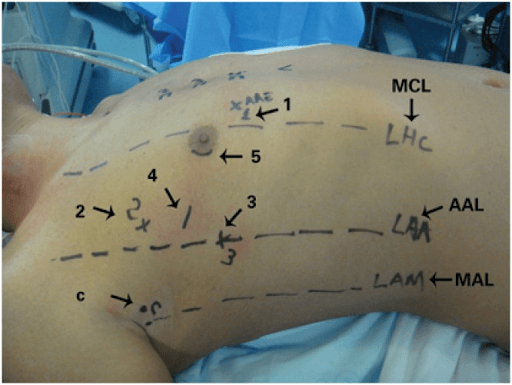
Figure 2: Preoperative markings and sites for the introduction of (1) left atrial retractor; (2) left robotic arm; (3) right robotic arm, (4) working trocar; (5) micro camera. C: transthoracic aortic clamp; MCL: midclavicular line; AAL: anterior axillary line; MAL: mid-axillary line (32)
Ethics of AI in Cardiology
To ensure the equitable and secure use of AI in cardiology, ethical precepts must be the main driver behind the implementation of such technology. A large variety of ethical concerns are associated with the use of AI in medicine, including the transparency of the decision-making of the AI, patient privacy, and ensuring researchers emphasise ethical efforts when developing AI (32). Most, if not all, of an AI system’s data is stored in an online database or sourced from electronic medical records (33). In addition, for AI to predict cardiac disease with a high degree of efficiency, the technology must be given access to a high volume of patient information (32). This raises questions about the security of AI analyses and whether or not patients truly have control over their health information (33). Legal implications of patient security may stunt the expansion of AI in healthcare, as the nature of AI directly opposes that of privacy; That is, AI thrives on obtaining as much information as possible. If patient privacy is not protected, the safety of many individuals may be in danger (33).
Data security and governance is undeniably a major issue when it comes to consumer privacy concerns. The ever-growing internet of things makes it inevitable for humans to now leave digital traces of their respective locations, digital communications and activity. Stakeholders also constantly seek consumer data collection in order to yield financial breakthroughs (34). Thus, deriving a method to protect sensitive wearable data from undesired data breaches should be prioritised as it is expected that data constantly moves from one platform to another.
Careful attention towards big data storage as well as the tracing of data origin and movements is needed to unleash the full potential of AI. Considering that patient records are not easily accessible by the patient themselves, but are shared across multiple health-care facilities and platforms, this subjects patients to feeling concerned over how data is managed and who manages said data. Therefore, new policies need to be constructed to ensure a safe and ethical exchange of data without compromising the patient’s privacy or accessibility to any data collected (34).
In conclusion, the plethora of data that can be made available from AI technologies necessitates that expectations are put in place between patients and medical providers. Data can provide clinicians with cardiovascular risk assessment, diagnosis and management tools, helping physicians develop even more personalised treatment and interventions for the patient. As long as health-care leaders and policymakers adapt and work through the challenges above, which are just a few among many others, AI integration in cardiovascular practice can be accelerated and perhaps, lead towards a new age of patient care (34).
In addition, the lack of an explanation behind the output of an AI system can decrease patient trust and reliability (34). Considering the vast data processing ability of AI, an explanation of the relationship between the input and output data becomes difficult. Understanding how millions of variables and data sets culminate to produce a final output is currently impossible (34). These “black box” rationalisations suggest that patients may not be able to give informed consent to an AI algorithm whose internal reasoning processes are unclear. Transparency should always exist between physicians and patients alike, and the integration of AI may impede this connection (34). Further research is encouraged to supplement the decision-making process of the AI. It is crucial for cardiologists, in the interest of their patients, to use AI-based tools with these ethical concerns in mind.
Conclusion
The use of AI in cardiology has the potential to change how doctors think, make decisions, and interpret data. There is a major need for AI in cardiology to supplement accurate predictions, diagnoses, and outcomes. The emergence of new, AI-driven diagnostic technologies, such as retinal scan analysis and wearable sensors, highlight the vast applications of AI in a cardiologic context. Via DL, the algorithm responsible for retinal scan analysis is able to predict the risk of a myocardial infarction with a high degree of accuracy. This technique aids in early detection and prevention, allowing patients to quickly receive the specialised care that is required. Similarly, wearable devices increase access to important diagnostic tools. These sensors provide the necessary input data to make AI algorithms possible. The biometric PPG sensor aids in both risk prediction and aftercare treatment of cardiovascular disease by recording various biomarkers, such as blood oxygen saturation. PA also has very important connections to cardiology. Monitoring an individual’s PA via accelerometers has been proven to increase PA and thus improve cardiovascular health.
Robotic surgery, too, points towards the improvement of longstanding healthcare practices. With the intervention of AI, surgery can become a safer and more effective process. ML and AI allow these robotic surgeons to replicate the work of experienced surgeons to a higher degree of accuracy than humanly possible.
Despite the promising outlook of these developing technologies, AI integration into healthcare is impeded due to ethical concerns. Questions surrounding safe data storage, patient privacy, and the gaps in reasoning of an AI system must be resolved before the widespread implementation of AI occurs. Ethical principles must guide the relationships between physicians, patients, and technology, especially in the context of AI.
AI has the potential to vastly improve diagnosis and treatment on a level that has been previously unseen. Crucial to the enhancement and personalisation of healthcare, AI will undoubtedly see major development in the coming years. The consideration of ethical precepts will ensure a sincere desire to develop, implement, and realise the revolutionary opportunities that AI provides. AI will continue to play a leading role in shaping the future of healthcare as a transformative tool for doctors and patients alike.
References
-
Khalsa RK, Khashkhusha A, Zaidi S, Harky A, Bashir M. Artificial intelligence and cardiac surgery during COVID‐19 era. Journal of Cardiac Surgery. 2021 Feb 10;36(5):1729–33. Doi: 10.1111/jocs.15417
-
Lopez-Jimenez F., Attia Z., Arruda-Olson A. M, Carter R., Chareonthaitawee P., Jouni H., Kapa S., Lerman A., Luong C., Medina-Inojosa J. R, Noseworthy P. A, Pellikka P. A, Redfield M. M, Roger V. L, Sandhu G. S, Senecal C., Friedman P. A, Artificial Intelligence in Cardiology: Present and Future. 2020;1015-1039. doi. 10.1016/J.mayocp.2020.01.038
-
Johnson K. W, Torres Soto J., Glicksberg B. S, Shameer K., Miotto R., Ali M., Ashley E., Dudley J. T, Artificial Intelligence in Cardiology. Journal of the American College of Cardiology. 2018; 2668–79. doi: 10.1016/J.JACC.2018.03.521
-
Org.uk British Heart Foundation. UK Factsheet. 2022 https://www.bhf.org.uk/-/media/files/research/heart-statistics/bhf-cvd-statistics—uk-factsheet.pdf
-
Centres for Disease Control and Prevention. Heart disease. 2022 https://www.cdc.gov/heartdisease/facts.htm
-
World Health Organisation. Cardiovascular diseases . 2019 https://www.who.int/health-topics/cardiovascular-diseases
-
Diaz-Pinto A, Ravikumar N, Attar R, Suinesiaputra A, Zhao Y, Levelt E, Dall’Armellina E, Lorenzi M, Chen Q, Keenan T. D. L, Agron E, Chew E. Y, Lu Z, Gale C. P, Gale R. P, Plein S, Frangi A. F. Predicting myocardial infarction through retinal scans and minimal personal information. Nature Machine Intelligence 2022 ;4(1):55–61. doi: 10.1038/s42256-021-00427-7
-
Ramos L, Novo J, Rouco J, Romeo S, Álvarez MD, Ortega M. Computational assessment of the retinal vascular tortuosity integrating domain-related information.Science Reports 2019 ;9(1):19940. doi: 10.1038/s41598-019-56507-7
-
CDC. Heart attack symptoms, risk, and recovery. Centres for Disease Control and Prevention. 2022. https://www.cdc.gov/heartdisease/heart_attack.htm
-
Lewis D. AI can identify heart disease from an eye scan. University of Leeds. 2022. https://www.leeds.ac.uk/main-index/news/article/5000/ai-can-identify-heart-disease-from-an-eye-scan
-
Schuster-Bruce C. Artificial intelligence could be used to detect heart disease from an eye scan, scientists say. Business Insider. 2022. https://www.businessinsider.com/ai-artificial-intelligence-heart-disease-diagnosis-diagnose-eye-scan-research-2022-1
-
Hewlett Packard Enterprise. What is Deep Learning? Deep learning. 2022 https://www.hpe.com/ca/en/what-is/deep-learning.html?jumpid=ps_s3kmeau4fc_aid-520061736&ef_id=CjwKCAjw5s6WBhA4EiwACGncZc-gESY9fIakEBXUDv3IJQkmRuqlbvCyFXHNXIXQKCeLhN1GNVgQWRoCED4QAvD_BwE:G:s&s_kwcid=AL!13472!3!595029827020!e!!g!!what%20is%20deep%20learning!17059486266!137675254042&
-
Kinno M, Waller A. H, Gardin J. M. Approaches to echocardiographic assessment of left ventricular mass: What does echocardiography add? American College of Cardiology. 2016 https://www.acc.org/latest-in-cardiology/articles/2016/02/02/08/21/approaches-to-echocardiographic-assessment-of-left-ventricular-mass
-
Nall R, MSN, CRNA. End-diastolic volume: What it is, effects, and conditions. Healthline. 2018 https://www.healthline.com/health/end-diastolic-volume
-
Phaneuf A. Latest trends in medical monitoring devices and wearable health technology . Insider Intelligence. 2022. https://www.insiderintelligence.com/insights/wearable-technology-healthcare-medical-devices
-
Bayoumy K., Gaber M., Elshafeey A., Mhaimeed O., Dineen E. H., Marvel F. A., Martin S. S., Muse E. D., Turakhia M. P., Tarakji K. G., & Elshazly M. B. Smart wearable devices in cardiovascular care: where we are and how to move forward. Nature Reviews Cardiology. 2021 18(8), 581–599. Doi: 10.1038/s41569-021-00522-7
-
Lee, S., Chu, Y., Ryu, J., Park, Y. J., Yang, S., & Koh, S. B Artificial intelligence for detection of cardiovascular-related diseases from wearable devices: A systematic review and meta-analysis. Yonsei Medical Journal. 2022;63(Suppl):S93. doi: 10.3349/ymj.2022.63.S93
-
Bayoumy, K., Gaber, M., Elshafeey, A., Mhaimeed, O., Dineen, E. H., Marvel, F. A., Martin, S. S., Muse, E. D., Turakhia, M. P., Tarakji, K. G., & Elshazly, M. B. Smart wearable devices in cardiovascular care: where we are and how to move forward. Nature Reviews Cardiology. 2021 18(8) 581–599. doi: 10.1038/s41569-021-00522-7
-
Norreel, J.-C. How wearable sensors are changing healthcare? Digital Health Central. 2021. https://digitalhealthcentral.com/2021/04/22/wearable-sensors-2/
-
Cheriyedath S, Smith Y. Photoplethysmography (PPG). News-medical.net. 2016 https://www.news-medical.net/health/Photoplethysmography-(PPG).aspx
-
Perez, M. V., Mahaffey, K. W., Hedlin, H., Rumsfeld, J. S., Garcia, A., Ferris, T., Balasubramanian, V., Russo, A. M., Rajmane, A., Cheung, L., Hung, G., Lee, J., Kowey, P., Talati, N., Nag, D., Gummidipundi, S. E., Beatty, A., Hills, M. T., Desai, S., Apple Heart Study Investigators.Large-scale assessment of a smartwatch to identify atrial fibrillation. The New England Journal of Medicine. 2019 Nov 14;381(20):1909–17. doi: 10.1056/NEJMoa1901183
-
PPG Sensors In Modern Technology. Sky Labs. 2021. https://skylabs.io/ppg-sensors-in-modern-technology/#:~:text=Utilising%20a%20 PPG%20 sensor%20is%20a%20great%20 way
-
Colilla, S., Crow, A., Petkun, W., Singer, D. E., Simon, T., & Liu, X. Estimates of current and future incidence and prevalence of atrial fibrillation in the U.S. adult population. The American Journal of Cardiology. 2013 Oct;112(8):1142–7. doi: 10.1016/j.amjcard.2013.05.063
-
Yang, C.-C., Hsu, Y.-L. A review of accelerometry-based wearable motion detectors for physical activity monitoring. Sensors (Basel). 2010 Aug 20;10(8):7772–88. doi: 10.3390/s100807772
-
Hannan, A. L., Harders, M. P., Hing, W., Climstein, M., Coombes, J. S., & Furness, J. Impact of wearable physical activity monitoring devices with exercise prescription or advice in the maintenance phase of cardiac rehabilitation: systematic review and meta-analysis. BMC Sports Science Medicine and Rehabilitation. 2019 Jul 30;11(1). doi: 10.1186/s13102-019-0126-8
-
Gardner, A. W., Parker, D. E., Montgomery, P. S., & Blevins, S. M. Step-monitored home exercise improves ambulation, vascular function, and inflammation in symptomatic patients with peripheral artery disease: a randomised controlled trial. Journal of the American Heart Association. 2014;3(5):e001107. doi: 10.1161/JAHA.114.001107
-
Maher, C., Szeto, K., & Arnold, J. The use of accelerometer-based wearable activity monitors in clinical settings: current practice, barriers, enablers, and future opportunities. BMC Health Services Research. 2021;21(1):1064. doi: 10.1186/s12913-021-07096-7
-
Newmarker C. Digital Surgery touts artificial intelligence for the operating room. Medical Design and Outsourcing. 2018. https://www.medicaldesignandoutsourcing.com/digital-surgery-touts-artificial-intelligence-for-the-operating-room/
-
Dias, R. D., Shah, J. A., & Zenati, M. A. Artificial intelligence in cardiothoracic surgery. Minerva Cardioangiologica. 2020 Oct 1;68(5):532–8. doi: 10.23736/S0026-4725.20.05235-4
-
Kassahun, Y., Yu, B., Tibebu, A. T., Stoyanov, D., Giannarou, S., Metzen, J. H., & Vander Poorten, E. Surgical robotics beyond enhanced dexterity instrumentation: a survey of machine learning techniques and their role in intelligent and autonomous surgical actions. International Journal of Computer Assisted Radiology and Surgery. 2015 Oct 8 11(4), 553–568. doi: 10.1007/s11548-015-1305-z
-
Poffo, R., Toschi, A. P., Pope, R. B., Celullare, A. L., Benício, A., Fischer, C. H., Vieira, M. L. C., Teruya, A., Hatanaka, D. M., Rusca, G. F., Makdisse, M. Cirurgia robótica em Cardiologia: um procedimento seguro e efetivo. Einstein (São Paulo) 2013;11(3):296–302. doi: 10.1590/s1679-45082013000300007
-
Thirumalaikolundusubramanian P, Meenakshisundaram R, Senthilkumaran S. Ethics, legality, and education in the practice of cardiology. In: Heart and Toxins. Elsevier; 2015. p. 595–623. doi: 10.1016/B978-0-12-416595-3.00023-2
-
Souza Filho EM de, Fernandes F de A, Pereira NC de A, Mesquita CT, Gismondi RA. Ethics, Artificial Intelligence and Cardiology. 2020; 115(3): doi: 10.36660/abc.2020014
-
Fenech M.E., Buston O., AI in Cardiac Imaging: A UK-Based Perspective on Addressing the Ethical, Social, and Political Challenges. Frontiers in Cardiovascular Medicine. 2020; 7: 54. doi: 10.3389/fcvm.2020.00054